Decoding Seamless AI Pricing Models and Factors
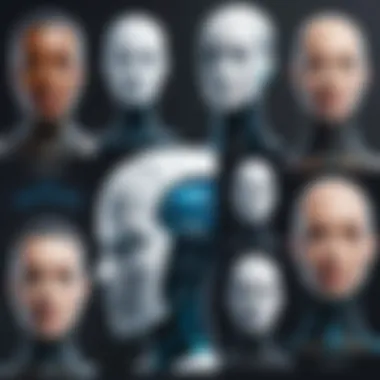
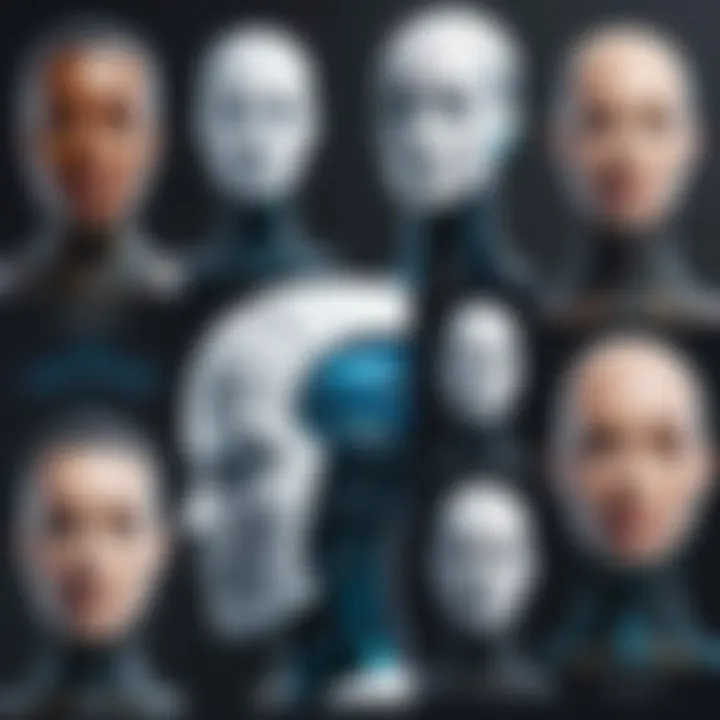
Intro
As businesses increasingly integrate artificial intelligence into their operations, understanding the pricing mechanisms behind these seamless AI solutions becomes crucial. The landscape is not just about adopting technology; it's about making sense of its economic implications. With different models available and various factors influencing costs, decision-makers must navigate through a cloud of complexity. This article aims to shed light on these intricacies, ensuring that stakeholders—from IT professionals to entrepreneurs—can make informed choices suited to their unique requirements.
In the following sections, we will delve into the essential features of AI software, unravel pricing models, analyze cost factors, and explore the implications for business decisions. Readers will not only grasp the core functionalities and advanced capabilities of AI technologies but will also comprehend the metrics that count when evaluating pricing structures.
Key Software Features
Understanding software features is critical when evaluating seamless AI technologies. Businesses need to recognize what sets one solution apart from another.
Essential functionalities
At the heart of any AI system lie its essential functionalities. These are the baseline capabilities that organizations should expect:
- Data Integration: The ability to connect with existing data sources ensures that AI can make informed decisions based on relevant inputs.
- Forecasting Modules: Many solutions include predictive analytics that aids businesses in anticipating future trends, essential for strategic planning.
- User Interface: A clean and intuitive user interface enhances usability, enabling teams to adopt and integrate AI solutions seamlessly.
Advanced capabilities
Moreover, some seamless AI technologies offer advanced functionalities that can transform business operations. These may include:
- Machine Learning Models: Tailored algorithms that learn from data, refining their predictions over time.
- Natural Language Processing (NLP): This allows computers to understand and interpret human language, enabling more effective customer interactions.
- Real-time Analytics: The capability to analyze data as it's generated facilitates quicker decision-making, which can provide a competitive advantage.
These features not only define the technological edge a company can gain, but they also play a significant role in shaping the overall pricing structure. When advanced capabilities are bundled into a seamless AI solution, costs usually reflect this added value.
Comparison Metrics
When considering AI solutions, it’s imperative to apply certain metrics to make a cogent comparison. Users should aim for a thorough cost analysis and performance benchmarks.
Cost analysis
Cost is often the tipping point in decision-making. Understanding the different pricing models—ranging from subscription-based to one-time licenses—provides clarity. Furthermore, ancillary costs associated with setup, maintenance, and training should factor into financial planning.
- Subscription Costs: These are typically billed monthly or annually and can include tiered service levels based on features accessed.
- One-Time Licenses: This model can seem appealing, but it often comes with hidden costs when updates or support are necessary.
- Service Fees: Factors like cloud storage, processing power, and integrations may lead to additional charges that can escalate over time.
Performance benchmarks
Evaluating performance benchmarks is equally vital. Metrics to consider include:
- Response Time: How swiftly an AI system processes requests can directly influence user satisfaction.
- Accuracy Rate: The precision of AI predictions or recommendations is paramount; high accuracy can justify a higher price point.
- Scalability: As businesses grow, their needs change. A flexible AI solution that can scale with the business is a considerable asset.
"The right AI technology can drive efficiencies, but understanding its cost structure is key to leveraging its full potential."
Ultimately, a comprehensive understanding of pricing dynamics in seamless AI should empower decision-makers to choose solutions that align not just with budgetary constraints, but also with strategic objectives. By combining an awareness of software features with an incisive look at cost and performance, organizations are positioned to harness the transformative power of AI efficiently.
Prelude to Seamless AI
In today’s fast-paced world, the rise of artificial intelligence (AI) is transforming not just individual businesses but entire industries. Understanding Seamless AI encapsulates this shift, particularly its pricing aspect. This section sets the stage for exploring how pricing models for AI solutions can greatly affect a company's bottom line and operational efficiency. The topic is crucial for decision-makers, IT professionals, and entrepreneurs who find themselves at the crossroads of incorporating AI into their daily processes.
Definition and Purpose
Seamless AI refers to the integration of artificial intelligence within business operations in such a way that it operates fluidly, without noticeable disruptions. Simply put, the objective is to make the use of AI as natural and advantageous as possible, allowing companies to leverage data intelligently without overwhelming their systems or staff. As for pricing, understanding the costs associated with these AI solutions ensures that organizations can make informed decisions that align with their overall strategic goals.
The heart of the matter is that the right pricing model enables businesses to scale their AI usage efficiently. For instance, a company that opts for a pay-per-use model could avoid high initial costs while gradually adopting AI technologies. This approach can lead to better budget allocation, thus fostering innovation without placing an undue financial burden on the organization. Additionally, clarity in pricing assists in evaluating the potential return on investment—a major concern for firms considering long-term commitments.
Overview of AI in Business
AI today finds applications across various sectors, from healthcare to finance and retail. It's a game-changer, so to speak. In the healthcare sector, for instance, AI technologies analyze vast amounts of data quickly, helping doctors make more informed decisions based on predictive analytics. In finance, AI algorithms streamline transactions and enrich customer experiences, giving businesses a competitive edge they previously lacked. And in retail, AI predicts inventory needs, optimizing supply chain management and enhancing customer satisfaction through personalized recommendations.
The integration of AI isn't just about adopting new technologies. It's about reshaping the way businesses think and operate. Keeping in mind the surge in AI usage, the necessity of understanding its pricing structures becomes paramount. The cost can vary depending on the sophistication of the solution, operational scale, and desired outcomes. As businesses begin to grapple with these complexities, they must weigh the value offered by AI against the costs associated with it.
"In the quest for digital transformation, understanding seamless AI pricing can mean the difference between flourishing and floundering."
This brings us to consider how companies can navigate the intricate web of pricing models available in today’s AI landscape. The next section will dive deeper into the various factors that influence these prices, ensuring that stakeholders can make empowered decisions conducive to their organizational objectives.
Factors Influencing Seamless AI Pricing
When it comes to the pricing of seamless AI technology, understanding the various factors at play is fundamental for decision-makers. Pricing doesn't merely pop out of thin air. It's shaped by a multitude of elements that reflect both the complexity of development and the market environment. Businesses need to get a grasp on these elements to make smart choices that align with their operational goals and budget constraints.
Development Costs
One of the primary components affecting AI pricing is the development costs associated with creating a solution. This involves not only the direct expenses related to hiring talent—data scientists, machine learning engineers, software developers—but also the indirect costs that come with research and innovation. Building AI systems often requires heavy investment in technology infrastructure, such as cloud services, data storage, and computing power. A developer may spend countless hours training models, which adds to the overall cost. The complexity of the task can vary significantly, and thus, estimates can swing wildly.
For example, a company creating a basic chatbot might incur lower development costs in comparison to one developing predictive analytics for healthcare—a field requiring high accuracy and extensive data compliance measures.
Operational Expenses
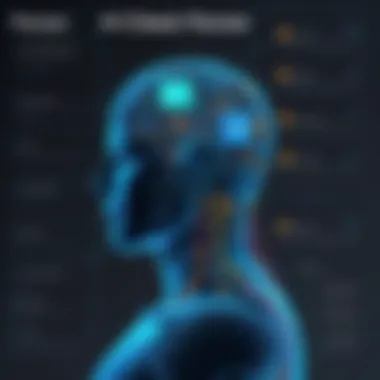
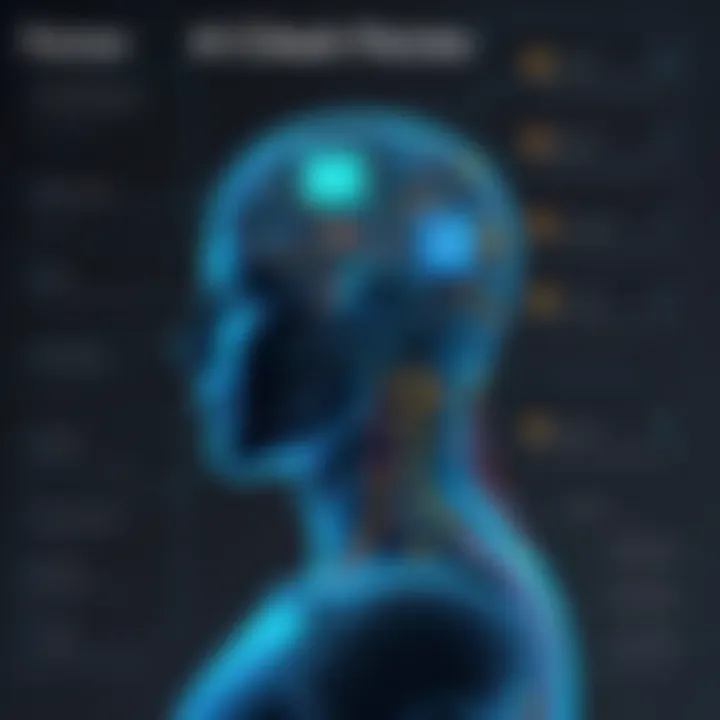
Another critical factor is operational expenses. Unlike development costs, which are more about initial setup, operational costs are ongoing. This includes everything related to maintaining the AI system once it's in place. Think software updates, server upkeep, and the continuous training of AI models. Companies often underestimate these recurring expenses, leading to nasty surprises later.
It's also important to consider labor costs for support and analysis. A highly skilled team is often required to monitor AI performance and make necessary adjustments. Notably, the efficiency and value of AI solutions may decrease without a proper operational framework. The more robust the operational strategy, the better the long-term financial health of the investment.
Market Trends and Demand
Market trends and demand cannot be overlooked when discussing AI pricing. As the adoption of AI in various sectors increases, so do expectations around functionality and performance. This creates a situation where businesses are often willing to pay a premium for solutions that meet contemporary standards and fulfill specific needs.
For instance, sectors such as finance require real-time data analytics, which drives innovation but also inflates costs. Conversely, if a specific AI application sees a surge in demand, pricing may skyrocket as more businesses vie for top-tier solutions, leading to competition among providers.
"Understanding demand dynamics can help companies predict potential price fluctuations and prepare accordingly."
Common Pricing Models for AI Solutions
The pricing strategies for AI solutions can vary wildly, much like choosing the right car to suit one's lifestyle. Understanding the common pricing models is crucial for businesses considering an AI implementation. Selecting the right model not only impacts the budget but also influences the overall success of the AI integration within a company’s existing systems. Several factors should inform this decision, including the scale of usage, return on investment expectations, and specific operational needs.
- Flexibility: With the right pricing model, businesses can adapt to changing requirements more easily.
- Scalability: Many organizations grow over time; a suitable pricing structure enables efficient scaling of AI solutions to meet increasing demands.
- Cost Control: Understanding models helps in anticipating expenses rather than being caught off guard.
Through this exploration, the specific elements, benefits, and considerations of each model will come into greater focus, aiding decision-makers in evaluating what suits their business needs.
Subscription-Based Pricing
Subscription-based pricing has become increasingly popular in the AI landscape. This model typically involves businesses paying a recurring fee to access the service over time, which can be monthly, quarterly, or annually. The primary allure here is predictability in budgeting. Businesses can allocate a fixed amount for AI tools without unexpected expenses cropping up, which can ease financial planning.
- Benefits:
- Predictable Costs: Easier financial management and planning.
- Continuous Updates: Subscribers often receive automatic access to software updates and new features, keeping them on the cutting edge.
- User Support: Many subscription models come with customer support as part of the package.
However, decision-makers need to carefully read the terms of service. Sometimes the base subscription might exclude critical features or impose limits on usage that could incur additional fees down the line.
Pay-Per-Use Pricing
The pay-per-use model aligns costs directly with actual usage. Think of it as paying for your electricity; you use it when needed and only pay for what you consume. This pricing model can be particularly appealing for businesses that want to manage costs tightly or expect irregular usage of AI services.
- Benefits:
- Cost Efficiency: Especially useful for companies that anticipate fluctuating needs, as they won’t pay for idle capabilities.
- Risk Mitigation: Businesses can try out AI solutions without a significant upfront investment and back out if the service doesn’t meet expectations.
Still, this model might not be the best fit for organizations with consistent high usage, as costs can spiral and lead to unexpected expenses. Balance is key.
Tiered Pricing Structures
Tiered pricing is somewhat of a middle ground, offering various levels of service at varying price points. This model allows businesses to pick a tier that aligns with their specific needs; for example, a small team might select a lower tier to start, whereas a larger enterprise could opt for premium features that cater to their extensive needs.
- Benefits:
- Customization: Organizations can find a match based on size, function, and budget constraints.
- Scalable Solutions: As businesses evolve, so can their tier level, making it easier to adapt without switching platforms.
While tiered pricing models offer great flexibility, businesses need to evaluate if they are getting enough value compared to the cost.
Freemium Models
Freemium models are interesting in that they offer basic services for free while charging for advanced features. This strategy can be a double-edged sword. Companies can use the free tiers to experiment and validate the utility of AI services without any financial commitment. However, the catch is that converting free users to paying customers often requires compelling upgrades.
- Benefits:
- Zero Initial Cost: Great for starting out, allowing businesses to explore solutions without expenditure.
- User Engagement: Free offerings can attract users quickly, and with time, many may opt for paying plans based on their needs.
Nevertheless, the freemium model might lead to commitment scatter, where users try bits and pieces without fully understanding the solution’s potential, leading to inefficiency in harnessing AI capabilities around the business.
In summary, understanding these common pricing models provides clearer insights to navigate the complex landscape of AI solutions. Selection depends heavily on the unique business needs, providing opportunities for cost-effective implementations without sacrificing quality or support.
Evaluating AI Pricing Against Business Value
Understanding how AI pricing stacks up against the value it brings to a business is critical for those who aim to make sound financial decisions. When companies invest in AI systems, they expect these solutions to not only solve existing problems but also to provide sustainable growth and efficiency. Evaluating AI pricing against business value involves weighing the costs incurred against the tangible benefits that the technology delivers. A fundamentally misguided focus on initial expenditure can lead to suboptimal outcomes in an organization, making this evaluation even more pertinent.
Cost-Benefit Analysis
A cost-benefit analysis is the foundation of a robust evaluation process. By dissecting all costs involved in acquiring and deploying an AI solution—like software licensing fees, infrastructure upgrades, and ongoing support—businesses can gather a clearer picture of what they stand to lose or gain.
- Direct Costs: This includes expenditures linked to software licenses, hardware, and any consulting services. These elements can add up quickly and should not be overlooked.
- Indirect Costs: Think about the opportunity costs—those losses incurred by not investing the capital elsewhere. A deep dive into how resources will be utilized can be revealing.
- Possible Benefits: Focus not merely on operational efficiency but also on qualitative gains such as improved customer experience or enhanced employee satisfaction.
Conducting this analysis offers stakeholders invaluable insight into whether the investment will yield significant returns. It may even point out that certain solutions are not worth the price tag due to the disparity between expected benefits and actual costs.
Return on Investment Considerations
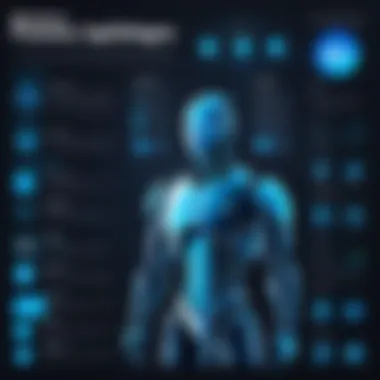
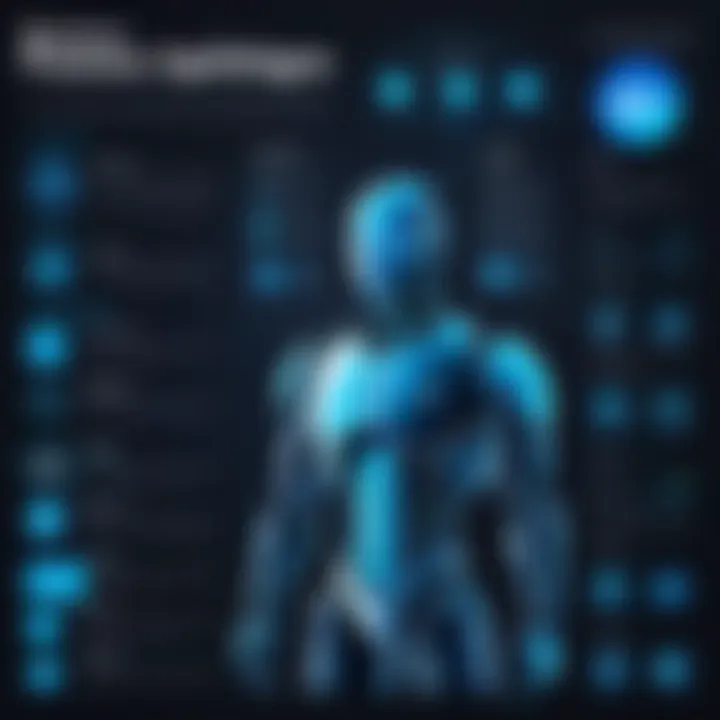
Once the cost-benefit analysis is complete, return on investment (ROI) serves as a critical metric to assess. ROI measures the profitability of an investment relative to its cost, expressed as a percentage. It informs decision-makers about how effectively their capital is being utilized and can offer varying perspectives based on the chosen time frame.
Some important factors in ROI considerations include:
- Time Frame: Short-term vs long-term investments carry different expectations. While short-term AI deployments may provide immediate benefits, long-term investments can yield exponential returns.
- Integration Costs: The ease of implementation affects ROI significantly. If AI systems demand extensive customization or training efforts, the time frame for realizing ROI might extend.
- Scaling Potential: AI solutions that can evolve alongside business growth will often provide a higher ROI. A solution that makes it easier to adapt to changing market conditions is invaluable.
Educating stakeholders on the nuances of ROI can result in more strategic investment decisions, aligning technological needs with financial forecasts. In this ever-evolving landscape, overlooking the relationship between AI pricing and business value can stall innovation.
"A dollar saved is a dollar earned, but a dollar invested wisely could lead to exponential returns."
By implementing thorough evaluations of both cost-benefit analyses and tailored ROI considerations, businesses empower themselves to actively engage in a landscape where AI can be leveraged to its full potential.
Case Studies from Various Industries
Case studies serve as a pivotal tool in illustrating how seamlessly AI pricing strategies have been deployed across diverse sectors. They highlight not only successful implementations but also the challenges and nuances of adopting these technologies in a real-world context. By examining specific instances where AI pricing influences decision-making and operational efficiencies, stakeholders can glean valuable insights that inform their own strategies.
Healthcare Sector
Within the healthcare industry, AI pricing models are particularly transformative. Hospitals and clinics are increasingly turning to AI to enhance patient care while managing costs. For example, consider a major hospital that implemented AI-powered diagnostic tools. The upfront investment for the AI system was significant, but it streamlined operations, reducing average patient diagnosis time by 30%, which in turn increased patient throughput.
The pricing model here often revolves around usage. Hospitals pay based on the number of diagnoses processed or consultations provided. This ensures that the healthcare provider only invests in AI services that correlate directly with their usage, rendering cost controls clearer. As they harness this technology, the ongoing operational expenses balance out against the improved service delivery and patient outcomes.
Notably, the integration of AI in patient monitoring systems has also shown potential cost savings and improved insurance reimbursements, making the case strong for predictive analytics within healthcare pricing frameworks.
Finance and Banking
In the finance sector, seamless AI pricing takes center stage with customer relationship management and risk assessment tools. A notable case is a prominent bank that adopted an AI-driven fraud detection system. The initial setup was not trivial, but the benefits became apparent quickly. The system’s capacity to analyze transaction patterns and flag anomalies resulted in a significant drop in fraudulent transactions.
This sector often leans toward a tiered pricing model based on transaction volume and the sophistication of the analytics desired. As the bank grows its user base, the scalable nature of AI pricing models implies that costs align closely with revenues. A clear advantage here is the tangible improvement in decision-making speed coupled with enhanced customer trust through fewer fraud incidents.
"AI's ability to interpret vast datasets allows banks to create tailored offerings that meet individual customer needs better than traditional methods."
Retail and E-Commerce
In the retail landscape, AI pricing strategies have reshaped how businesses compete. Consider a leading e-commerce platform that employed dynamic pricing algorithms to adjust prices in real-time based on demand, competitor pricing, and inventory levels. This flexible pricing model led to a noticeable uptick in sales during peak shopping periods while also managing inventory more efficiently.
Retailers often adopt a freemium model, where basic analytic tools might be offered for free, encouraging businesses to explore enhanced paid services as their operations scale. The beauty of this approach is that it allows smaller retailers to enter the AI space without overwhelming up-front costs, gradually transitioning to more complex systems as they observe firsthand the value AI can deliver.
By drawing parallels across these industries, it becomes clear that seamless AI pricing is adaptable, responsive, and directly tied to operational outcomes. Each case underscores the importance of aligning AI strategy with business objectives, providing a roadmap for other organizations looking to embark on similar journeys.
Negotiating AI Pricing Contracts
Negotiating AI pricing contracts is a complex yet crucial aspect for businesses looking to integrate seamless artificial intelligence into their operations. For many organizations, this negotiation process can be the make-or-break factor in ensuring they get the most value for their investment while mitigating risks associated with AI deployment. Not only does it involve understanding the financial implications, but it also requires a keen eye for detail when it comes to the terms and conditions that govern the partnership between the vendor and the client.
Having a clear grasp of the negotiation process allows businesses to navigate the labyrinth of pricing dynamics. The importance of negotiating AI pricing goes beyond mere cost savings; it encompasses a wider range of factors, from securing favorable terms to defining the scope of service, performance metrics, and potential liabilities. Knowing the art and science behind these negotiations can set one organization apart from its competitors in the quest for a robust AI solution.
Understanding Terms and Conditions
When diving into the nitty-gritty of AI pricing contracts, the terms and conditions are the backbone that supports the entire agreement. Understanding these terms is not just beneficial; it’s essential. They outline the responsibilities of each party, payment schedules, service level agreements, and contingencies for unforeseen circumstances. A good grasp of the terms enables decision-makers to identify potential pitfalls early on.
- Payment Structure: This includes what you will pay, when you’ll pay it, and if any hidden fees lurk around the corner.
- Service Levels: Clearly defined expectations about performance levels, response times, and maintenance schedules can save significant headaches later.
- Termination Clauses: Knowing the conditions under which either party can exit the contract is vital to avoid being tied down without recourse.
- Liability and Risk: These terms will stipulate who bears the responsibility in case something goes wrong.
Understanding these areas thoroughly can empower a business to negotiate more favorable conditions.
Tips for Effective Negotiation
Navigating through AI pricing contracts is somewhat akin to dancing: it’s all about rhythm, timing, and awareness of your partner's moves. Certain strategies can enhance the likelihood of favorable outcomes during negotiation.
- Do Your Homework: Research before diving into negotiations. Understand market standards, alternative options, and what other vendors offer.
- Clarify Your Needs: Be clear about your organization's requirements and how the AI solution aligns with them. Vague objectives can lead to unfavorable terms on both sides.
- Prioritize Flexibility: Stipulate terms that allow for adjustments as needs change in the future. AI is an evolving field, and your contract should accommodate that evolution.
- Leverage Competitive Offers: When multiple vendors are in play, don’t shy away from leveraging their offers against each other. It’s a solid bargaining chip.
- Seek Expert Advice: For larger projects, involving a legal expert experienced in tech contracts can safeguard your interests and ensure thorough documentation is in place.
By keeping these strategies in mind, decision-makers can approach negotiations not just with confidence but with a clear roadmap that leads to beneficial agreements.
The way contracts are negotiated can often dictate the fundamental health of the relationship between the vendor and the company, making it more than just a mere financial transaction.
Emerging Trends in AI Pricing
As organizations delve deeper into the world of artificial intelligence, the landscape of AI pricing is constantly evolving. Recognizing these emerging trends is crucial for decision-makers wanting to align their strategies effectively. Understanding how these trends shape pricing models can be a game-changer in making informed procurement choices.
Dynamic Pricing Models
Dynamic pricing models are becoming increasingly popular in the AI sector. In essence, these models adjust prices based on various factors, such as demand, competition, and even customer behavior. Think of it like airlines changing ticket prices based on how many seats are left on a flight. This approach allows businesses to maximize revenue while being responsive to market conditions.
Benefits of dynamic pricing include:
- Real-Time Adjustments: Businesses can modify prices instantly to reflect current market trends, ensuring they stay competitive.
- Enhanced Revenue Management: This model allows for optimizing profits by charging more during peak demand and less when competition is fierce.
- Improved Customer Insights: With each pricing decision tied to data analytics, companies can gain valuable insights into customer preferences and behaviors.
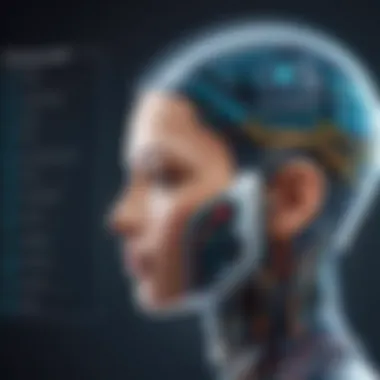
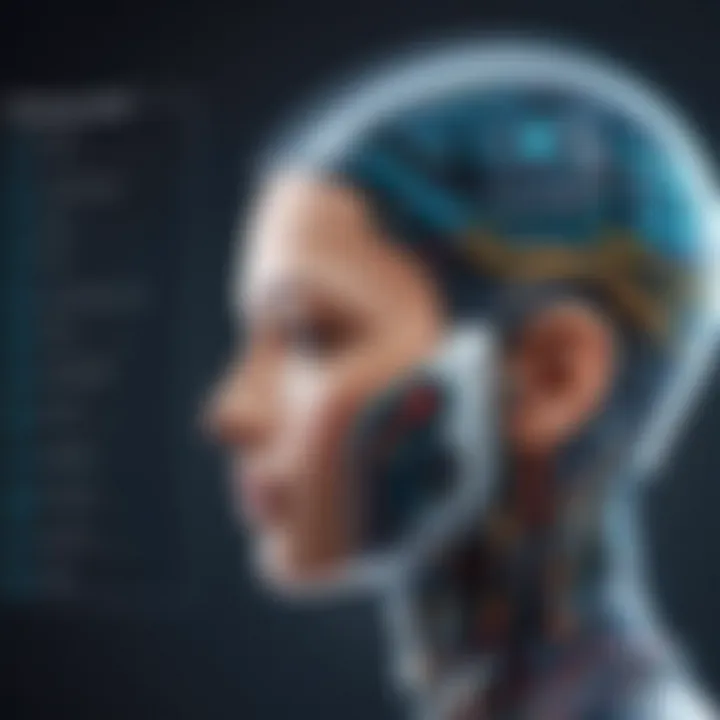
However, implementing this model isn’t without its challenges. Companies must invest in robust analytics tools that can handle fluctuating data reliably, or they risk mispricing and losing out on potential sales.
"The most effective dynamic pricing strategies come from a deep understanding of both the market and the customer."
Value-Based Pricing Strategies
Value-based pricing strategies focus on the perceived value of AI solutions rather than solely on the costs involved in development or implementation. This approach considers what customers are willing to pay based on the actual benefits and enhancements these technologies bring to their business.
Key considerations when utilizing value-based pricing include:
- Customer Focus: It’s essential to understand your customer’s needs and what they value most. Customizing your offering to these aspects can justify higher prices.
- Differentiation: If your AI solution provides unique features or performance that competitors lack, you can command a premium price.
- Long-Term Relationships: When customers perceive high value, there is a greater likelihood of customer loyalty, often leading to recurring revenue.
To effectively implement value-based pricing, a company might use surveys or market research to gather insights about customer perceptions and preferences. This can tailor offerings to ensure they meet customer expectations.
In summary, as the field of AI pricing continues to evolve, staying abreast of dynamic pricing models and value-based pricing strategies will empower businesses to make smarter, data-driven decisions. Leveraging these trends not only aligns pricing with customer value but also enhances overall competitiveness in an increasingly crowded marketplace.
Challenges in Seamless AI Pricing
When diving into the world of AI pricing, businesses quickly realize that they are in for a wild ride. The complexities involved can be dizzying. This section aims to shed light on the key challenges that companies face when trying to navigate the murky waters of seamless AI pricing. Getting these aspects right is not just about saving a few bucks; it can make or break how efficiently an organization can implement AI solutions. The clearer understanding of these challenges, the better decisions can be made.
Transparency Issues
In the realm of AI pricing, transparency often feels like a moving target. Many AI service providers present their pricing structures in ways that can be more puzzling than helpful. This ambiguity can lead to situations where businesses don't fully understand what they are paying for, or worse, the quality and scope of the service being offered. The concept of transparency goes beyond just showing numbers; it involves laying bare the methodologies behind the pricing.
For instance, a company might advertise a subscription plan that includes a range of features, yet the fine print could reveal additional costs tied to certain services. These not-so-obvious extra costs can quickly inflate budgets and catch decision-makers off guard. This lack of clear communication can lead to dissatisfaction, distrust, and potentially even legal disputes.
To tackle transparency issues, companies should insist on comprehensive breakdowns of costs and services. Establishing clear lines of communication with AI vendors can also help manage expectations. A well-informed customer can negotiate better deals and ensure that they are getting their money's worth, thus reducing the risk involved in investment decisions.
Comparative Analysis Difficulties
Another hurdle that businesses must leap over is the challenge of comparative analysis. It's not enough to just understand a single AI pricing model; organizations need to compare various options to determine which is truly the best fit. Unfortunately, comparing AI solutions can become a labyrinthine process.
Different vendors might utilize various metrics to present their pricing, creating an apples-to-oranges situation where direct comparisons are almost impossible. Some companies might emphasize upfront costs, while others might highlight long-term savings associated with lower operational expenses.
To overcome these difficulties, potential buyers should adopt a structured approach:
- Identify Key Metrics: Focus on parameters that align with your business goals, such as scalability, uptime, and customer support.
- Standardize the Comparisons: By developing a checklist or a scoring system, businesses can measure offerings fairly against each other.
- Seek External Advice: Sometimes, a third-party consultant can provide valuable insights and offer a neutral opinion on pricing structures.
In the end, not only does understanding these challenges contribute to making savvy financial choices, but it also lays the groundwork for fostering long-term partnerships with AI vendors.
Recommendations for Businesses
In the ever-evolving realm of artificial intelligence, making informed decisions about pricing can make or break a business's bottom line. With numerous options on the table, a careful approach to selecting the right pricing models is essential for maximizing value. This section focuses on critical recommendations for businesses contemplating the integration of seamless AI solutions, emphasizing the importance of tailored strategies in navigating the complexities of pricing.
Identifying Suitable Pricing Models
When diving into AI pricing, one size does not fit all. Each organization has its own set of unique needs and financial constraints. The first step is to assess the specific business objectives and the desired outcomes from AI implementations. Businesses should consider the following:
- Type of AI Service Needed: Different AI solutions, from natural language processing to machine learning-driven analytics, come with varying price tags. For example, a company seeking machine learning capabilities may benefit from a subscription-based model that caters to fluctuating demand.
- Usage Patterns: Understanding usage is crucial. If an organization anticipates sporadic usage, a pay-per-use pricing model could save costs, whereas a more extensive, consistent use might justify a tiered pricing structure.
- Long-term vs. Short-term Engagements: If a business plans a long-term commitment to AI, negotiating a fixed annual cost can be advantageous. This not only locks in pricing but may also lead to discounts over time.
As organizations navigate through their choices, it’s vital to look beyond initial costs to the potential ROI. By correctly identifying the most suitable pricing models, businesses can ensure they aren’t just buying a service, but investing in a long-term partnership that aligns with their evolving needs.
Allocating Budget Effectively
Budget allocation for AI technology should be treated with the same scrutiny as any sizeable investment. It’s about striking a balance between leveraging innovative solutions and managing risk factors that come with new technology. Here are some practical steps to ensure effective budget allocation:
- Conduct a Comprehensive Needs Assessment: Before putting pen to paper, understanding what the AI solution is expected to deliver can guide budget decisions. Identifying specific applications—be it improving operational efficiency or enhancing customer experiences—will help prioritize expenditures.
- Factor in Hidden Costs: AI implementation isn't just about the sticker price. Consider expenses such as training staff, system integration, and ongoing maintenance.
- Establish a Contingency Fund: Given the fast-paced nature of technology, it's wise to set aside a portion of the budget for unexpected expenses related to AI deployment or upgrades.
- Review Financial Projections Regularly: Markets can change overnight, especially in tech. Regular financial reviews can help make informed decisions about whether to continue, adapt, or even pivot AI strategies.
- Engage Stakeholders in Budget Discussions: Including different teams—production, marketing, finance—in budget talks can provide a broader perspective on how AI can benefit the entire organization and highlight areas where investment is wisely spent.
"Investing in AI isn’t just a financial decision. It’s about reshaping the potential of a business in today’s digital environment."
By taking these measures to allocate their budgets strategically and thoughtfully, organizations can harness the power of AI technologies more effectively, ensuring they extract maximum value from their investments.
The End
The conclusion serves as a crucial element in understanding the broader implications of pricing in the AI landscape. It encapsulates the insights drawn from the various sections of the article, knitting the concepts together while paving the way for future considerations. A well-rounded conclusion highlights the importance of recognizing how pricing structures in AI can directly influence business decisions and outcomes. Understanding the nuances of AI pricing not only aids decision-makers in selecting the right solutions, but also prepares them to navigate the complexities as AI technologies evolve.
Summary of Key Points
In this exploration of seamless AI pricing, several key takeaways emerge:
- Diverse Pricing Models: From subscription, pay-per-use to tiered structures, there's a model for every business.
- Cost Implications: Development costs, operational expenses, and market trends all contribute to the final price of AI solutions.
- Evaluating Value: Conducting a cost-benefit analysis and considering the return on investment is essential to justify AI expenditures.
- Negotiation Strategy: Businesses must be equipped to effectively negotiate pricing contracts to secure the best deals.
- Emerging Trends: Keeping an eye on dynamic pricing and value-based strategies can help organizations remain competitive.
These points underscore the significance of adapting to the ever-changing AI marketplace and prioritizing strategic financial planning.
Future Outlook for AI Pricing
As we peer into the future of AI pricing, a landscape marked by flexibility and responsiveness unfolds. Here are some trends that can define upcoming pricing strategies:
- Increased Customization: As AI technologies become more specialized, companies will likely adopt pricing models that reflect a higher level of customization, addressing specific client needs rather than offering one-size-fits-all solutions.
- AI-Powered Pricing Models: The advent of artificial intelligence in pricing strategy itself can revolutionize how companies evaluate costs. Predictive analytics may guide pricing based on demand forecasts and competitor analysis.
- Focus on Value Creation: Businesses are shifting towards frameworks that align pricing with the actual value gained from AI solutions, rather than just costs incurred.
- Regulatory Influences: With potential regulations on data privacy and security impacting AI operational costs, companies need to be mindful of how these changes can affect pricing structures.
"The future of AI pricing isn't just about costs; it's about aligning technology with strategic business goals and outcomes."
In sum, understanding seamless AI pricing requires ongoing evaluation and adaptation as new trends emerge and markets shift. Decision-makers, IT professionals, and entrepreneurs should stay informed and agile to seize the opportunities that these developments present.