Understanding Predictive Pricing: Comprehensive Insights
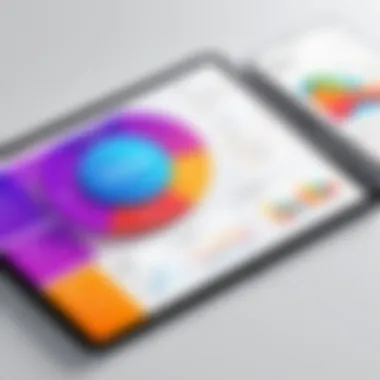
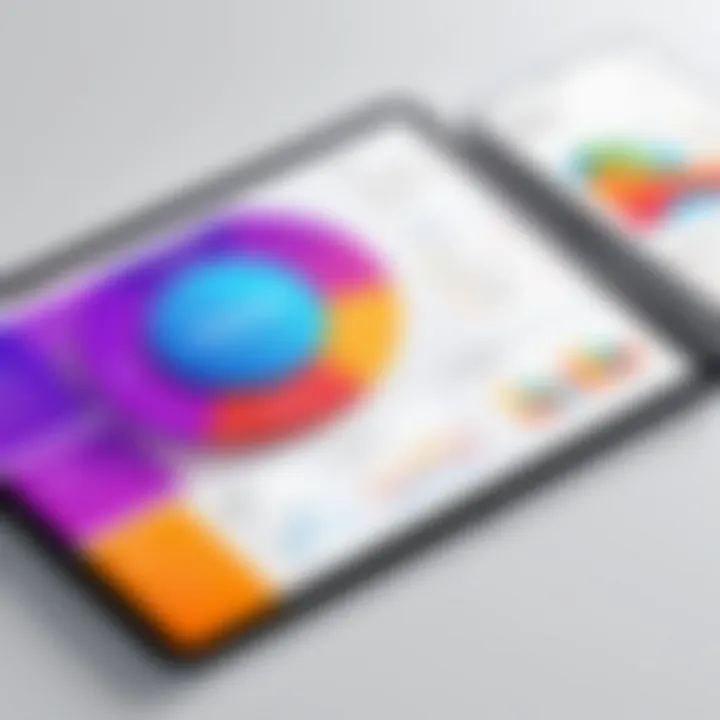
Intro
Predictive pricing is an evolving strategy that utilizes data analytics to forecast optimal pricing for products or services. This method integrates various data sources, employing statistical techniques and machine learning models to analyze consumer behavior, market trends, and other influencing factors. With the rapid advancement of technology, organizations are increasingly recognizing the importance of predictive pricing in maintaining competitive advantage.
The significance of this approach can be reflected in its ability to enhance revenue management, align pricing with customer willingness to pay, and optimize inventory levels. Furthermore, sectors such as retail, e-commerce, and hospitality can significantly benefit from such methodologies. This article will provide a comprehensive exploration of predictive pricing, highlighting its features, methodologies, and the technology that supports it, along with its implications for market dynamics and consumer behavior.
Key Software Features
The effectiveness of predictive pricing relies heavily on the features and capabilities of the software systems that facilitate its implementation. Organizations need to understand what functionalities are essential and which advanced capabilities can enhance their predictive pricing strategies.
Essential functionalities
When evaluating predictive pricing software, there are several core features that are critical:
- Data Integration: Ability to gather and process data from various sources, including sales history, market conditions, and consumer behavior.
- Analytics Tools: Incorporation of statistical methods and algorithms to analyze data and predict pricing trends.
- User-Friendly Interface: A simple interface that allows decision-makers to easily navigate the system, visualizing the pricing strategies and outcomes.
- Reporting Capabilities: Generation of comprehensive reports that provide insights into the effectiveness of pricing strategies and their impact on sales.
Advanced capabilities
In addition to essential functionalities, organizations can benefit from advanced features that offer deeper insights and more refined predictive capabilities:
- Machine Learning Models: The integration of machine learning enhances the accuracy of predictions based on historical data patterns.
- Real-Time Analytics: Providing up-to-the-minute pricing suggestions based on immediate changes in market conditions or competitor pricing.
- Scenario Analysis: The ability to simulate various pricing scenarios and assess their potential impacts on revenue and market share.
"Predictive pricing not only rationalizes price adjustments but also aligns them with real-time consumer sentiment."
Comparison Metrics
When selecting a predictive pricing solution, comparing different options requires a systematic approach. Key comparison metrics should include cost analysis and performance benchmarks.
Cost analysis
Evaluating the cost-effectiveness of predictive pricing software involves:
- Upfront Costs: The initial investment required for the software itself, which may vary significantly between options.
- Operational Costs: Ongoing expenses associated with data management, updates, and personnel training.
- Return on Investment: Estimating potential increases in revenue due to optimized pricing strategies can help justify the costs.
Performance benchmarks
Assessing the performance of predictive pricing software is crucial for ensuring it meets organizational objectives:
- Accuracy of Predictions: How closely the software’s predictions match actual price performance can reveal its effectiveness.
- User Adoption Rates: Understanding how well the team integrates the software into daily operations can indicate ease of use and utility.
- Impact on Sales: Monitoring the changes in sales volume and revenue after implementing the predictive pricing strategy provides insight into overall success.
Through careful evaluation of these features and metrics, decision-makers can choose the right predictive pricing software that aligns with their organizational goals, maximizing both efficiency and profitability.
Defining Predictive Pricing
Predictive pricing represents a sophisticated approach to pricing strategy, aimed at optimizing revenue through data-driven insights. This section seeks to illuminate the significance of understanding predictive pricing, particularly in a landscape increasingly influenced by data analytics and technological advancements.
Effective predictive pricing entails anticipating future customer behavior and market trends. Businesses leveraging predictive pricing can better position their products and services, ensuring they capture maximum value. With the rise of big data, organizations need to grasp this concept more than ever to remain competitive.
Incorporating predictive pricing can lead to various benefits, such as improved pricing accuracy, enhanced profitability, and a refined customer experience. Understanding its intricacies becomes paramount for decision-makers and IT professionals tasked with implementing such strategies.
Clarifying the Conceptual Framework
The conceptual framework of predictive pricing includes several critical components. At its core, predictive pricing integrates various analytical techniques, including statistical analysis, machine learning, and algorithmic modeling. These techniques analyze historical data to forecast future pricing strategies effectively.
Central to this framework is the understanding of how market dynamics influence pricing. Different sectors may exhibit distinct characteristics that warrant custom-tailored predictive models. For instance, retail might emphasize seasonal trends, while e-commerce could focus on real-time inventory levels. A solid grasp of these differentiating factors is essential for creating a functional predictive pricing model.
Historical Context and Evolution
Historically, pricing strategies were predominantly reactive, relying more on intuition and less on empirical data. However, with the advent of technology and data availability, the evolution toward predictive pricing has been significant. This shift can be traced back to early attempts at dynamic pricing, where companies adjusted prices based on simple demand forecasts.
As technology advanced, so did the sophistication of predictive pricing models. The introduction of advanced analytics, combined with machine learning's computational power, has transformed how businesses understand and implement pricing strategies. Today, predictive pricing is not merely an option but a necessity in various industries.
The journey from rudimentary pricing adjustments to highly developed predictive models illustrates the critical role data plays in shaping contemporary pricing strategies. Understanding this evolution can help businesses appreciate the importance of investing in relevant technologies and methodologies.
Key Components of Predictive Pricing Models
Understanding the key components of predictive pricing models is vital for businesses aiming to optimize their pricing strategies. These components incorporate systematic and structured approaches that help in forecasting price movements and consumer responses effectively. By mastering these elements, organizations can achieve a competitive advantage in an evolving market landscape.
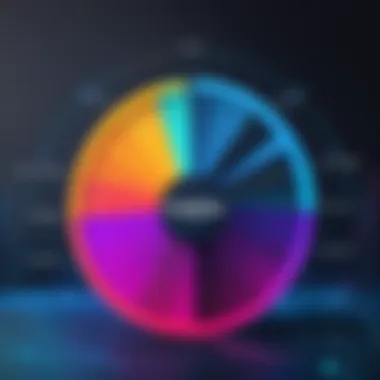
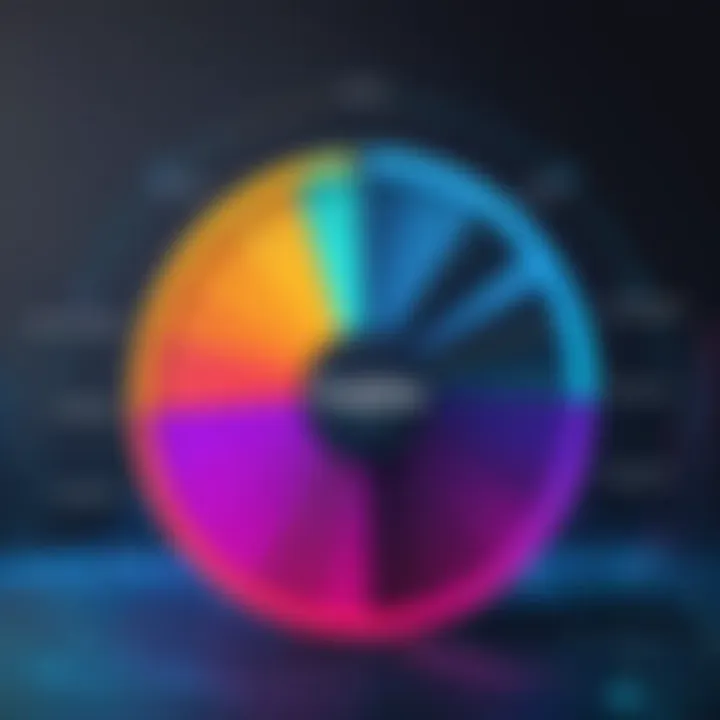
Data Collection Methodologies
Data collection stands as the backbone of any predictive pricing model. Accurate data is essential for reliable predictions. There are various methodologies for gathering this data, including surveys, transaction histories, and web scraping techniques. Each method has its own advantages and challenges, which decision-makers need to consider carefully.
Surveys can yield valuable information directly from consumers, shedding light on their preferences and price sensitivities. However, they can be subject to biases. Transactional data provides a historical perspective on consumer behavior and sales trends, underpinning the model's analytical framework. Web scraping collects real-time data on competitor pricing, allowing businesses to adjust their strategies dynamically.
Integrating different data sources enhances the model's robustness. Following best practices, such as ensuring data accuracy and relevance, significantly increases the quality of insights drawn from the data.
Analytical Techniques Employed
Once data is collected, the next step involves employing analytical techniques to make sense of it. Various statistical methods and models play a crucial role in understanding price elasticity, predicting demand, and identifying market trends. Techniques such as regression analysis are frequently used to establish relationships between different variables.
Predictive analytics can uncover patterns that are not immediately obvious. Through these techniques, businesses can formulate pricing strategies that are not just reactive but proactive, anticipating market shifts before they occur.
The interplay of analytical techniques and data sets the foundation for successful predictive pricing. Key performance indicators should be clearly defined to measure the effectiveness of these models over time, allowing continuous refinement.
Machine Learning and AI Integration
The integration of machine learning and artificial intelligence has the potential to revolutionize predictive pricing. These advanced technologies allow for more sophisticated modeling techniques that adapt to changing market conditions and consumer behaviors automatically over time.
Machine learning algorithms can analyze vast datasets at speeds unattainable by humans. They detect patterns and correlations that can drive pricing decisions based on real-time data. AI can also simulate various pricing scenarios to predict outcomes under different situations. This helps businesses prepare strategies well in advance.
Furthermore, machine learning models improve over time. With enough data, they self-optimize, enhancing predictive accuracy with each additional dataset. As market dynamics shift, these models can adjust without manual intervention, providing businesses with a significant edge.
Integrating robust data collection, effective analytical techniques, and advanced machine learning algorithms creates a powerful predictive pricing model.
Applications of Predictive Pricing
Understanding predictive pricing becomes essential when evaluating its various applications across different industries. The capacity to analyze historical data coupled with current market trends significantly influences pricing strategies. More companies prioritize predictive pricing as it enables them to enhance their competitive edge and achieve better financial outcomes.
Retail Sector Innovations
In the realm of retail, predictive pricing has brought forth innovations that redefine how retailers interact with customers. Retailers utilize data analytics to determine optimal pricing strategies based on consumer behavior and market conditions. By leveraging historical purchase data and patterns, these businesses can forecast how price changes might influence sales volumes. This allows them to set prices that balance profit margins with consumer demand effectively.
Additionally, some retailers implement dynamic pricing strategies, adjusting prices in real time based on competitor pricing, demand shifts, or inventory levels. The adoption of these techniques can lead to increased sales and improved customer loyalty. Consumers, who perceive tailored pricing as more favorable, often respond positively, further solidifying the retailer's position in the market.
E-commerce Strategies and Trends
E-commerce businesses also experience significant advantages through predictive pricing. With extensive data at their disposal, online retailers can analyze shopping behaviors and preferences across a vast audience. This data allows for the segmentation of consumers based on various factors such as purchase history, location, and even time of day.
The implementation of algorithm-driven pricing tools enables these businesses to adjust prices automatically based on real-time analytics. For instance, Amazon employs such advanced pricing models, dynamically adjusting prices in minutes based on numerous variables. This strategic agility not only boosts conversion rates but enhances overall customer satisfaction through perceived value.
Predictive Pricing in Hospitality
In the hospitality sector, predictive pricing optimizes revenue management practices. Hotels and resorts analyze data from booking patterns, seasonality, and local events to set room rates that maximize occupancy while maintaining profitability. Such sophisticated pricing strategies help hospitality businesses manage their yield effectively.
For example, a hotel might increase its rates during peak vacation seasons or major local events by analyzing historical booking trends. On the other hand, predictive pricing can also assist in identifying times when discounts or promotions might encourage bookings. This increases occupancy rates during typically low-demand periods, thus balancing revenue throughout the year.
"Predictive pricing is not just about setting prices; it's about aligning with customer expectations and market realities."
The multifaceted use of predictive pricing models across different sectors showcases their ability to adapt strategies to changing environments. A thorough understanding of these applications sets the groundwork for making informed decisions about implementing predictive pricing solutions.
Impact on Consumer Behavior
Predictive pricing plays a significant role in understanding consumer behavior. By analyzing data and trends, businesses can gain valuable insights into how consumers react to price changes. This section explores two critical aspects: changes in price sensitivity and the perception of value and fairness.
Changes in Price Sensitivity
Price sensitivity refers to how responsive consumers are to price changes. Predictive pricing can alter this sensitivity drastically. For instance, if a customer knows that prices fluctuate based on demand or timing, they may alter their buying habits accordingly. Consumers often begin to expect price changes, leading them to delay purchases in anticipation of lower prices. This behavior influences sales forecasting.
- Dynamic Pricing Models: Companies use software that analyzes market demand and adjusts prices real-time. This adaptability can create uncertainty among consumers.
- Consumer Education: Businesses must ensure that customers comprehend their pricing strategies. Educating consumers builds trust. When clients understand the reasons behind price changes, they may perceive fairness even with fluctuations. An informed consumer may be more willing to accept changes in pricing.
Understanding price sensitivity is crucial for retailers and service providers. As they implement predictive pricing, they must consider how it changes customer attitudes as well as spending behavior.
Perception of Value and Fairness
The perception of value is a subjective measure. How consumers perceive the relationship between price and quality can shape their willingness to purchase. Predictive pricing strategies must align with consumer expectations. If customers believe they are getting value, they are likely to accept price adjustments.
- Value Communication: Companies should communicate value propositions clearly. When businesses highlight product benefits or unique selling points, it strengthens the perceived value.
- Sense of Fairness: Price fairness is an essential element in consumer decisions. When customers feel that a price is fair, they are less likely to complain about increases. Predictive models that analyze historical data can help to establish what consumers consider fair pricing.
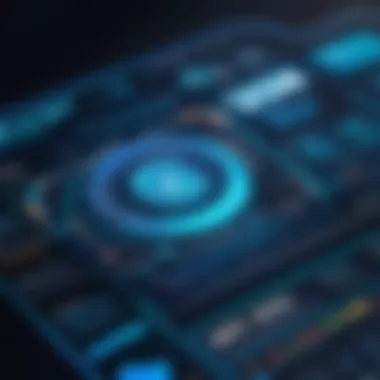
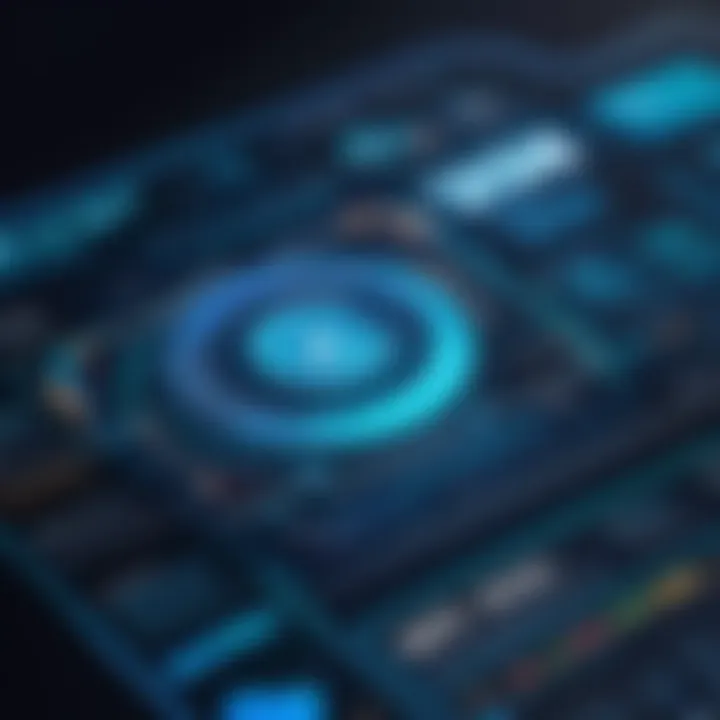
"Understanding consumer perception of value and fairness is essential. It can make or break a company's pricing strategy."
Benefits of Implementing Predictive Pricing
Predictive pricing is not merely a trend but a strategic imperative for businesses aiming to optimize their revenue and remain competitive. By leveraging sophisticated algorithms and analyzing vast datasets, organizations can harness predictive pricing to achieve multiple benefits. This section will delve into the key elements including how it enhances revenue management and improves customer insights. These elements underscore the significance of integrating predictive pricing models into business strategies.
Enhanced Revenue Management
The use of predictive pricing directly influences revenue management. Businesses can use data-driven insights to set prices intelligently, maximizing profit margins while remaining attractive to consumers. By analyzing customer behavior, market conditions, and historical data, firms can anticipate the best prices that consumers are willing to pay. This allows them to adjust pricing in real-time, responding to demand fluctuations.
For instance, airlines often employ dynamic pricing strategies based on seat availability and upcoming travel dates. These adjustments lead to increased revenue as they can fill seats during low demand and capitalize on high demand during peak seasons.
By anticipating shifts in demand, businesses can adjust their pricing strategies and avoid leaving money on the table.
Key Benefits of Enhanced Revenue Management:
- Increased profit margins through optimal pricing.
- Ability to respond quickly to market changes.
- Improved inventory management leading to reduced excess stock.
- Understanding of customer willingness to pay, guiding promotional strategies.
Improved Customer Insights
Another significant benefit of predictive pricing lies in its ability to provide deeper customer insights. Businesses are now using advanced analytics to understand purchasing patterns, preferences, and behavior. This information can be invaluable as it guides not only pricing strategies but also marketing campaigns and product offerings.
For example, by analyzing data from previous sales, companies can identify which products are frequently purchased together. This allows firms to create bundles or targeted promotions that appeal directly to consumer preferences.
Moreover, improved customer insights can lead to enhanced customer experience. When customers feel that prices reflect their expectations of value, their loyalty and satisfaction increase.
Advantages of Improved Customer Insights:
- Tailored marketing strategies that resonate with consumer behavior.
- Enhanced personalization of offers and promotions.
- Increased customer loyalty resulting from perceived fairness in pricing.
- Better understanding of market segments leading to focused product development.
In summary, the implementation of predictive pricing not only enhances revenue management but also improves insights into customer behavior. For decision-makers and entrepreneurs, it's essential to consider these benefits. They provide compelling reasons to adopt predictive pricing approaches, ensuring competitive advantage in the market.
Challenges and Limitations
Predictive pricing, while promising in its potential to optimize revenue and enhance customer insights, is not without its challenges and limitations. Every advancement in technology presents obstacles that need to be addressed. In this section, we will explore two significant issues that can impact the effectiveness of predictive pricing models: data quality and availability issues, along with regulatory considerations.
Data Quality and Availability Issues
Data serves as the backbone of predictive pricing strategies. The quality of data directly influences the accuracy of pricing models. High-quality data must be accurate, timely, and relevant to drive the insights necessary for effective pricing decisions.
However, the challenges in securing quality data often stem from various factors:
- Inconsistent Data Sources: Data can often come from multiple sources, including internal databases and third-party vendors. If these sources lack consistency, they can lead to misleading or erroneous insights.
- Data Completeness: Sometimes, datasets are incomplete. Missing data points can skew results, leading to incorrect pricing strategies.
- Real-Time Data Access: The market is dynamic, and timely data is crucial. Organizations often struggle to access and analyze data rapidly, hindering responsiveness in setting prices.
Poor data quality can result in opportunistic pricing strategies that may alienate consumers or lead to lost revenue. Companies are therefore encouraged to invest in robust data management and cleaning processes to mitigate these issues.
Regulatory Considerations
The landscape of predictive pricing is also shaped by regulatory frameworks. Governments and regulatory bodies have a crucial role in overseeing pricing strategies to ensure fair practices. As predictive pricing evolves, businesses face several regulatory challenges:
- Transparency Requirements: Companies must ensure transparency in their pricing methodologies. Consumers are increasingly discerning about how prices are determined, and opaque practices can sour customer trust.
- Consumer Protection Laws: Compliance with laws that protect consumers from unfair pricing practices is paramount. Companies must navigate these regulations, or they risk legal repercussions.
- Data Privacy Regulations: With the heightened focus on data privacy, regulations such as GDPR in Europe put strict limits on how consumer data is collected and utilized. Companies must balance the use of data for predictive pricing with the need to protect consumer privacy.
These regulatory considerations demand that businesses not only focus on technological implementation but also on legal compliance. The challenge lies in aligning predictive pricing strategies with these evolving regulations.
"The combination of data quality and regulatory compliance presents a dual challenge that organizations must navigate carefully to capitalize on predictive pricing benefits.”
In summary, understanding the challenges related to data quality and regulatory considerations is imperative for decision-makers. Addressing these issues effectively enhances the success of predictive pricing models and fortifies a business’s position in the competitive market.
Predictive Pricing and Software Solutions
The integration of predictive pricing into business strategies is increasingly reliant on specialized software solutions. These tools elevate the effectiveness of pricing strategies through sophisticated data analysis, automation, and actionable insights. Selecting the right software can significantly influence a company's ability to adapt to market changes and optimize revenue. Here, we explore the key elements of these software solutions and how they can provide substantial benefits to various industries.
Key Software Features to Consider
When evaluating software for predictive pricing, decision-makers should prioritize several essential features:
- Data Integration: The ability to pull in data from various sources—such as sales history, customer demographics, and market trends—is crucial. This integration allows for a comprehensive view of pricing dynamics.
- Real-Time Analytics: Software solutions must offer real-time data processing capabilities. This feature ensures that businesses can adjust their pricing strategies quickly in response to market shifts or consumer behavior changes.
- User-Friendly Interface: It is vital that pricing tools are accessible and easy to use, allowing team members to derive insights without extensive training.
- Customizable Algorithms: Different industries have unique pricing challenges. Software should allow customization of predictive models to suit specific business needs.
- Reporting and Visualization Tools: Effective visual tools help in interpreting complex data, facilitating better decision-making by presenting trends and insights clearly.
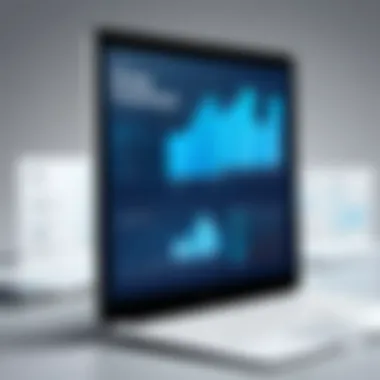
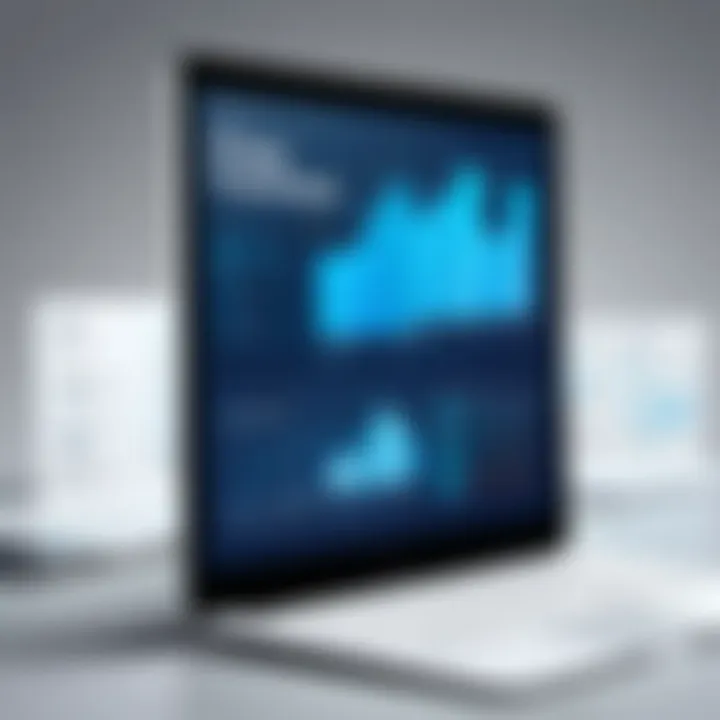
By focusing on these features, enterprises can choose software that supports effective predictive pricing strategies.
Comparative Analysis of Available Tools
The market offers a diverse range of software solutions for predictive pricing, each with its strengths and weaknesses. A comparative analysis can help stakeholders identify which tools best meet their requirements.
- Dynamic Pricing Tools: Tools like Dynamic Pricing by Omnia Retail and Pricefx offer comprehensive solutions that leverage AI to suggest the most effective prices based on market conditions.
- Data Analytics Platforms: Solutions such as Tableau and Qlik provide extensive data visualization and reporting capabilities, but might require additional tools for specific pricing task.
- Dedicated Pricing Solutions: PROS and Zilliant focus specifically on pricing strategies. They offer advanced features tailored to businesses that operate in competitive environments and need agile pricing approaches.
Each option has its advantages. For instance, while Dynamic Pricing by Omnia Retail excels in real-time pricing adjustments, PROS may provide deeper insights into customer segments. Businesses need to align their needs with the offerings of these different solution providers to maximize the benefits of predictive pricing.
Each of these software options plays a distinctive role in leveraging data to create more effective pricing strategies. Choosing the right one can lead to significant enhancements in revenue management.
Case Studies of Successful Implementation
Exploring case studies of predictive pricing serves as a practical lens to understand its relevance and impact in various industries. By analyzing how different companies have successfully integrated predictive pricing into their strategies, we gain insights into best practices, obstacles faced, and measurable benefits realized. These real-world examples showcase the flexibility of predictive pricing across diverse sectors, illustrating its potential to drive innovation and improve revenue.
E-commerce Giants Leading the Way
E-commerce platforms have been at the forefront of predictive pricing implementation. Amazon is a prime example. The company uses sophisticated algorithms that analyze customer behavior, competitor prices, and market trends to adjust its pricing in real-time. This strategy enhances their competitive edge and increases sales volume.
Another notable case is Zalando, a fashion retailer. They employ predictive pricing to optimize their inventory management. By analyzing past sales data and seasonal trends, Zalando can forecast demand more accurately. This approach reduces excess inventory and ensures popular items are always available.
Key aspects from these examples include:
- Real-time data analysis enables rapid response to market changes.
- Enhanced customer experience through personalized pricing strategies.
- Maximization of sales opportunities by predicting demand.
"Predictive pricing allows e-commerce businesses to anticipate customer needs, driving sales and customer loyalty."
Hospitality Sector Success Stories
In the hospitality industry, companies like Hilton leverage predictive pricing to optimize their room rates. By applying machine learning algorithms that take into account factors such as local events, historical booking data, and competitor pricing, Hilton achieves a dynamic pricing model. This ensures they are competitive while maximizing occupancy rates.
Airbnb is another great example of successful implementation. The platform uses predictive pricing tools to help hosts determine appropriate pricing based on demand signals and property specifics. This enhances profitability for hosts while making the platform appealing to guests with varying budgets.
Components of success in the hospitality sector include:
- Increased revenue through data-driven pricing strategies.
- Better inventory management by anticipating customer booking patterns.
- Improved customer satisfaction by offering targeted pricing tailored to different segments.
Through these case studies, it becomes evident that predictive pricing is not merely a theoretical concept but a functional approach that companies can leverage for significant gains.
Future Trends in Predictive Pricing
As businesses navigate an ever-changing economic landscape, understanding the future trends in predictive pricing is crucial. It offers insights into how organizations can leverage data and technology to stay competitive. The evolution of pricing strategies helps firms optimize revenue while maintaining customer satisfaction. Therefore, it's vital to examine emerging technologies and market dynamics that shape predictive pricing methodologies.
Emerging Technologies and Techniques
New technologies continuously influence predictive pricing. Developments in data analytics and machine learning enable organizations to refine pricing models. Modern systems can analyze vast amounts of data, allowing for real-time adjustments based on consumer behavior.
- Artificial Intelligence: It provides algorithms that learn from sales patterns. AI can predict future pricing trends more profoundly than traditional methods.
- Big Data Tools: These facilitate the processing and analysis of large datasets, enhancing decision-making. Companies can use tools like Tableau or Power BI to visualize data.
- Cloud Computing: This offers scalability and accessibility for predictive pricing applications. Organizations can deploy resources without heavy investments in infrastructure.
The combination of these technologies fosters a more personalized pricing strategy. As firms integrate these innovations, they will likely see improved accuracy in their pricing predictions. This advancement leads to better customer targeting and satisfaction.
Impact of Global Market Dynamics
Global market dynamics play a critical role in shaping predictive pricing strategies. The interconnectedness of markets means that companies must be agile. Economic changes in one region can impact prices and demand in another.
Several factors need to be considered:
- Economic Fluctuations: Inflation or economic downturns affect consumer purchasing power. Predictive pricing must account for these shifts to remain relevant.
- Competitor Actions: Organizations should closely monitor their competitors' pricing strategies. Analyzing competitors helps companies adjust their pricing in real time.
- Regulatory Changes: Compliance with laws can influence pricing. Companies need to adapt their pricing models based on new regulations in their industry.
Finale: The Path Forward
The discussion surrounding predictive pricing is essential for businesses aiming to understand their market dynamics and consumer preferences. As various industries move towards data-driven decision-making, predictive pricing offers a strategic way to optimize pricing strategies. The potential benefits of implementing predictive pricing models include enhanced revenue management and improved customer insights, which play a vital role in maintaining competitiveness in today's fast-paced market.
Strategic Recommendations for Businesses
When considering the implementation of predictive pricing, businesses should take the following recommendations into account:
- Invest in High-Quality Data: Accurate and relevant data is crucial. Organizations must prioritize quality over quantity in their data collection efforts, ensuring that the data is reliable and relevant to their pricing strategies.
- Adopt Advanced Analytical Tools: Utilize machine learning and AI technologies to analyze data trends and predict future pricing scenarios. Tools such as IBM Watson and Google Cloud AI can assist in deriving actionable insights.
- Encourage Collaboration Across Departments: Successful predictive pricing initiatives should involve collaboration among marketing, sales, and IT departments. Sharing insights helps create a more coherent strategy that aligns with business objectives.
- Regularly Monitor and Adjust Models: Predictive pricing models should not be static. Continuous monitoring is essential to adapt to changing market conditions and consumer behaviors. This might include periodic reviews of algorithms and adjusting parameters based on recent data.
- Educate Stakeholders: It is vital to ensure that all stakeholders understand the principles of predictive pricing. Training programs can help employees grasp the benefits and methods, thus increasing overall buy-in.
Final Thoughts on Predictive Pricing
Predictive pricing represents a significant evolution in how businesses approach pricing strategies. The integration of technology and data analytics is reshaping the landscape, enabling companies to make informed decisions that cater to customer preferences and market trends. As we move forward, it becomes increasingly important for organizations to embrace these tools.
"Understanding and adopting predictive pricing will not only enhance profitability but also strengthen customer relationships through tailored pricing strategies."