Top Data Analytics Tools of 2020: Comprehensive Insights
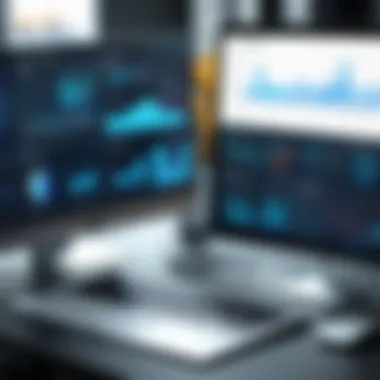
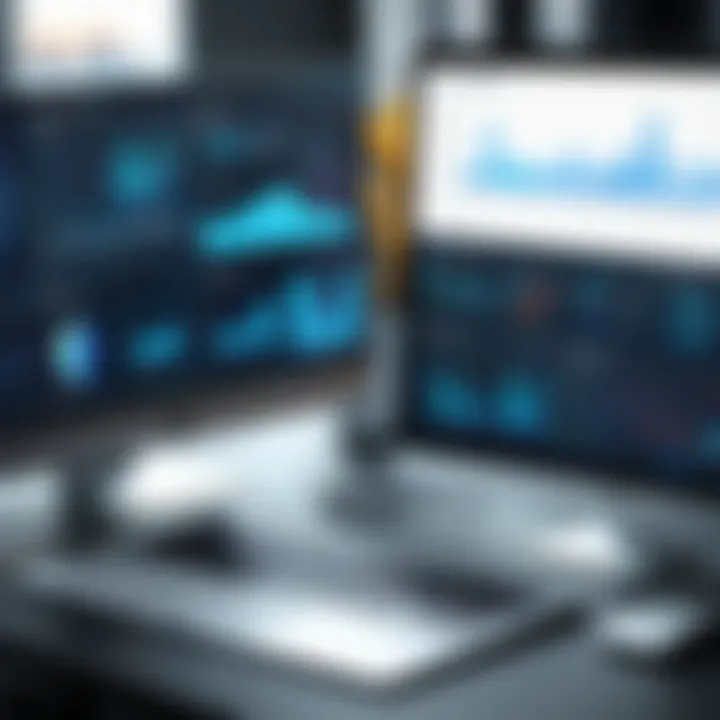
Intro
The world of data analytics has undergone significant transformation in recent years. As businesses increasingly rely on data-driven decision-making, the tools available have evolved to offer enhanced functionality, user experience, and integration capabilities. This comprehensive guide will explore the top data analytics tools available in 2020. By examining each solution, we aim to shed light on how these tools are shaping the industry.
Understanding the features and functionalities of these tools is crucial for professionals. Selecting the right software should align with organizational objectives and technological requirements. Hence, our exploration will address critical aspects ranging from basic features to advanced capabilities.
Key Software Features
Essential functionalities
Data analytics tools come with a variety of essential features. These include:
- Data visualization: Users can easily create charts and graphs to represent data, making it more digestible.
- Data cleansing: This functionality helps in correcting errors and improving data quality.
- Real-time analysis: Enables users to view data as it is generated, facilitating timely decision-making.
- Collaboration tools: These help teams work together in real-time, enhancing workflow.
These core features are fundamental for any data analytics tool. They provide users the capability to interpret data accurately and swiftly.
Advanced capabilities
For more sophisticated users, the advanced capabilities of data analytics tools can significantly enhance functionality. Key aspects include:
- Predictive analytics: Using algorithms to forecast future trends based on historical data.
- Machine learning integration: Allowing tools to learn from data patterns over time, improving insights.
- Custom dashboards: Users can tailor their analytics interface to focus on specific metrics and KPIs that matter most.
These advanced features can provide distinct advantages, enabling professionals to leverage their data more effectively.
Comparison Metrics
Cost analysis
When making a decision on which data analytics tool to use, cost can be a decisive factor. Tools often vary widely in pricing, not only for initial purchase but also for ongoing operational expenses. It is important to assess the total cost of ownership, including subscription fees, maintenance, and support.
Performance benchmarks
Performance is another critical metric for evaluation. This can include speed of data processing, accuracy of results, and overall user experience. Testing various tools against these benchmarks will assist in determining which solution offers the best return on investment for your specific needs.
Understanding the specific capabilities of these tools is essential for making informed decisions that will drive effective data utilization.
Through a structured comparison of software features and metrics, professionals can align their choices with their organization’s goals. With this guide, we hope to remove ambiguity from the selection process, directing users toward the most suitable data analytics tools of 2020.
Preface to Data Analytics Tools
Data analytics tools are essential in today's data-driven environment. They help organizations transform raw data into actionable insights. This is especially significant in the context of the current business landscape where decision-makers need reliable information to steer their strategies. Understanding data analytics tools is a stepping stone towards leveraging sophisticated capabilities for enhancing operational efficiency and competitive advantage.
Definition of Data Analytics
Data analytics refers to the systematic computational analysis of data. It involves gathering, processing, and analyzing data to uncover patterns and trends that can inform decisions. Various methods and tools are employed to analyze the data effectively. Common techniques include statistical analysis, data mining, and predictive modeling. Organizations use these processes to make sense of complex data sets, leading to precise forecasting and improved decision-making.
Importance in Decision-Making
The role of data analytics in decision-making cannot be overstated. It directly influences the strategic planning processes within organizations. Decision-makers rely on data analytics to gain insights into market trends, customer behavior, and operational performance.
"Informed decision-making is reliant on accurate data interpretations."
The importance of data analytics tools is seen in areas such as:
- Risk Management: Identifying potential risks through data analysis helps mitigate unforeseen complications.
- Performance Tracking: Organizations can monitor their performance and make necessary adjustments swiftly.
- Customer Insights: Understanding customer preferences leads to better product development and marketing strategies.
In summary, data analytics tools empower organizations to operate more efficiently and make educated choices, significantly impacting their success.
Criteria for Selecting Data Analytics Tools
Selecting the right data analytics tool is crucial for professionals aiming to extract meaningful insights from data. The criteria for choosing these tools affect not only the effectiveness of the tool itself but also its integration into existing workflows and the overall impact on decision-making processes. Each element warrants careful evaluation to ensure that organizational goals align with the capabilities of the selected solutions.
Functionality and Features
Functionality is one of the foremost criteria that must be considered when selecting a data analytics tool. Different tools offer varying features that cater to specific needs. For example, some tools provide advanced visualization capabilities, which are essential for transforming complex data sets into understandable graphs. Others focus on predictive analytics, which can forecast future trends based on historical data. Key functionalities to assess include:
- Data processing speed: How rapidly can the tool analyze large data sets?
- Visualization options: What types of graphs and charts can be created?
- Reporting features: Are there customizable reporting options?
- Collaboration capabilities: Can teams share insights easily within the tool?
Choosing a tool that meets these functional needs can lead to more comprehensive data analysis and effective strategic decisions.
User Experience and Interface
User experience plays a significant role in the adoption and usability of data analytics tools. A well-designed interface allows users to navigate easily, reducing the time taken to learn the software. It's important to consider how intuitive the tool is for individuals who may have varying levels of technical expertise. Key aspects to evaluate include:
- Ease of navigation: Is the layout user-friendly?
- Learning curve: How much time is needed to become proficient in using the tool?
- Support resources: Are there tutorials or customer support available for users?
Prioritizing a good user experience can enhance productivity and ensure that teams effectively engage with analytics without unnecessary frustration.
Integration with Other Software
Effective data analytics often relies on the ability of a tool to integrate with other software within an organization's tech stack. A data analytics tool should seamlessly connect with existing Customer Relationship Management (CRM) systems or data storage solutions. Some considerations for assessing integration capabilities are:
- Compatibility: Does the tool work well with existing hardware and software?
- APIs and connectors: Are there available APIs for data import/export?
- Collaboration with other teams: How well does the tool interact with platforms used across departments?
Strong integration capabilities result in streamlined workflows, improving overall efficiency and data accuracy.
Cost and Licensing Options
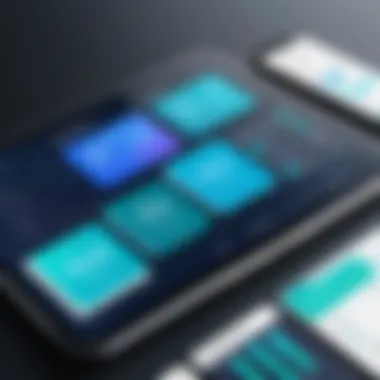
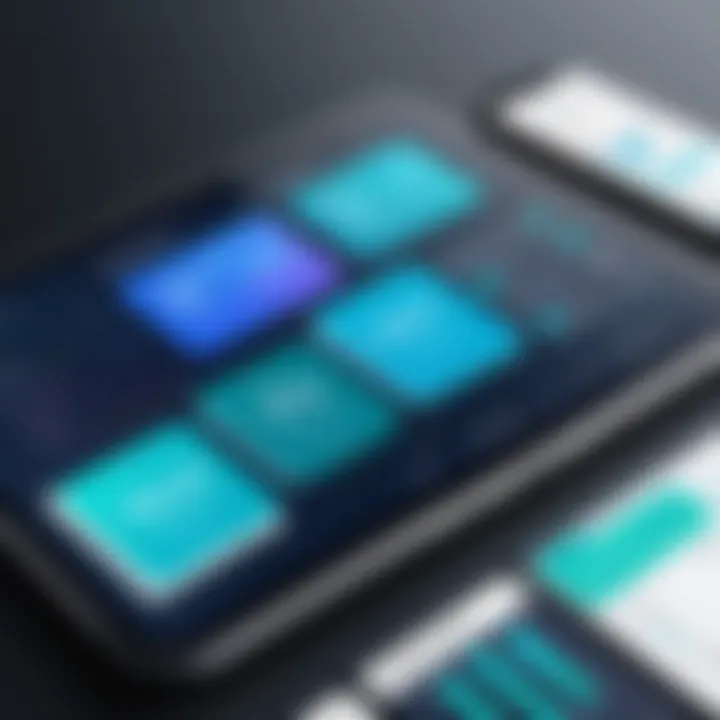
The cost of data analytics tools can vary vastly. Understanding the pricing model is essential for budgeting and ensuring value for money. Tools may offer various licensing options, such as:
- One-time purchase vs. subscription: Is the payment model more suited to the organization's financial structure?
- Scalability costs: How will costs change as the business grows?
- Hidden fees: Are there additional costs for accessories or features?
Understanding these costs can help stakeholders make informed budgetary decisions and choose a tool that fits their financial constraints without compromising functionality.
Overview of the Leading Data Analytics Tools
In the rapidly evolving field of data analytics, being informed about the top tools available is essential for professionals. This section highlights the significance of understanding various data analytics tools that shaped the industry in 2020. Each tool offers unique functionalities, thus impacting how organizations interpret data.
Choosing the right data analytics tool can mean the difference between insightful data-driven decisions and missed opportunities. The tools listed here have established themselves based on robust performance, user satisfaction, and innovative features. By providing a comprehensive overview, readers can weigh the benefits and limitations associated with each tool.
Tool One: Tableau
Key Features
Tableau is renowned for its powerful data visualization capabilities. It supports a wide array of data sources and allows for seamless integration. A standout feature is its drag-and-drop functionality, which simplifies the creation of complex visualizations. This ease of use makes Tableau a preferred choice among users seeking to communicate data insights quickly.
Another key characteristic is the real-time data connection. Users can see live updates, ensuring that the analytics reflect the latest data. Additionally, Tableau's collaboration features facilitate team efforts, making it easier to share visual reports.
Use Cases
Tableau serves diverse industries: from healthcare for analyzing patient data to retail in monitoring sales performance. It is particularly beneficial for business intelligence teams because it enables non-technical users to manipulate and analyze data.
One unique aspect of Tableau is its community-driven resource base which provides extensive tutorials and help forums. This fosters continued learning and helps users maximize the tool's capabilities.
Pros and Cons
The advantages of Tableau include its user-friendly interface and robust analytical functionalities. However, the pricing can be a barrier for smaller organizations. Additionally, while Tableau excels at visualization, it may not be as strong in statistical analysis compared to other tools.
Tool Two: Google Analytics
Key Features
Google Analytics is a cornerstone for web data analysis. Its ability to dissect user behavior on websites stands out. Users can track traffic sources, user engagement metrics, and conversion rates easily.
A significant characteristic is its integration with Google Ads. This allows for efficient performance tracking of marketing campaigns, making it a beneficial tool for digital marketers.
Use Cases
This tool is particularly useful for e-commerce businesses to optimize online sales and for content creators to assess audience interaction with their content. Its dashboard provides a user-friendly way to present critical metrics, which supports decision-making.
One unique feature is the custom reporting, which allows users to tailor data presentation based on specific needs. This flexibility enhances usability for various stakeholders.
Pros and Cons
The strengths of Google Analytics include its comprehensive tracking and free access at a basic level. However, the learning curve can be steep for new users. Moreover, some advanced features may require a subscription to Google Analytics 360, which could add cost constraints.
Tool Three: Microsoft Power BI
Key Features
Microsoft Power BI stands out with its ability to convert raw data into insightful dashboards and reports. One notable aspect is its strong integration with Microsoft products like Excel and Azure, helping provide a seamless experience for users already familiar with the Microsoft ecosystem.
Another characteristic that makes Power BI appealing is its affordability compared to other analytics tools. This accessibility extends to small and mid-sized businesses that are looking for effective data visualization tools.
Use Cases
Power BI is suitable for various applications including financial analysis and performance tracking. Businesses utilize it to visualize complex datasets effectively, thus informing strategic decisions.
Additionally, the ability to publish reports and share real-time insights enhances collaboration among departments.
Pros and Cons
Pros of Microsoft Power BI include its cost-effective pricing model and integration capabilities. Yet, there can be limitations in terms of data storage compared to other tools. Sometimes users may encounter challenges with complex data modeling.
Tool Four: SAS
Key Features
SAS is known for its advanced analytics capabilities. It offers a suite of software solutions for statistical analysis, predictive modeling, and data mining.
The main advantage is its strong analytical power. SAS facilitates the processing of large datasets effectively, making it preferable for sectors requiring extensive data analysis.
Use Cases
SAS is often used in sectors like finance, healthcare, and academia, where detailed statistical analysis is needed. Its applications range from fraud detection to patient outcome analysis.
A unique feature is its programming language, which allows extensive customization and flexibility. However, this can necessitate a higher level of expertise from users to leverage effectively.
Pros and Cons
The strengths of SAS lie in its powerful analytics and data management capabilities. However, it tends to be costly and may not be the best option for organizations with limited budgets. Furthermore, its complexity may discourage new users.
Tool Five: QlikView
Key Features
QlikView is distinctive for its associative data model which enables users to explore data freely rather than by predefined parameters. This characteristic encourages discovery by allowing users to ask questions without rigid structures.
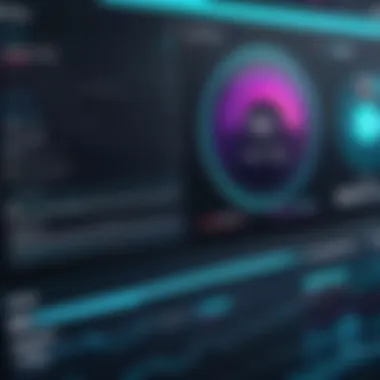
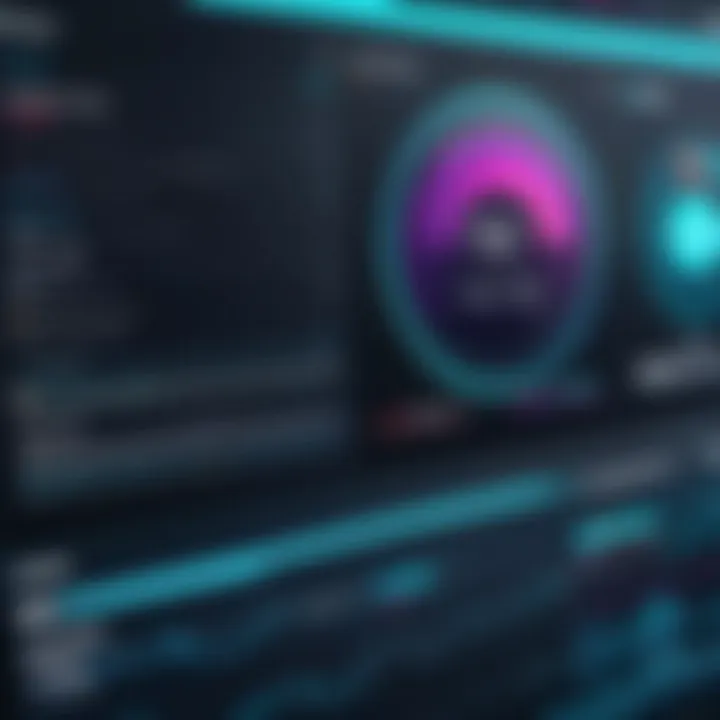
One key feature is its in-memory processing, which enhances speed and efficiency in data retrieval and visualization.
Use Cases
Commonly used in sectors such as retail and manufacturing, QlikView supports use cases that require quick access to data insights. The intuitive dashboard aids users in navigating through vast datasets effortlessly.
The unique selling point is its self-service capabilities, enabling users to create reports without heavy reliance on IT resources.
Pros and Cons
QlikView's associative model is a notable advantage, promoting exploration of data connections. However, its pricing structure can be confusing, and some users find it less adaptable for complex data sets compared to competitors.
Tool Six: IBM Watson Analytics
Key Features
IBM Watson Analytics uses artificial intelligence to enhance data analysis. One of its standout features is its natural language processing capabilities, allowing users to ask questions naturally and get meaningful insights.
The intuitive interface aids users in navigating through analytics without a steep learning curve, making it accessible for various user levels.
Use Cases
Industries such as healthcare and finance have seen benefits from Watson Analytics through improved decision-making based on AI-driven insights. It serves well in predictive analytics scenarios, enhancing strategic planning processes.
The unique aspect is its automated data preparation, which reduces the time needed for data cleaning and structuring.
Pros and Cons
The use of AI is a significant advantage, as it streamlines the analytics process. However, some pricing tiers may hinder smaller businesses from accessing its full range of features.
Tool Seven: Looker
Key Features
Looker emphasizes data modeling, allowing teams to build custom dashboards based on organizational metrics. Its integration with Google Cloud enhances its functionalities, providing stickers to those already using Google products.
A key feature is its LookML language, which provides a consistent framework for business analytics.
Use Cases
Looker is suited for organizations looking to create tailored reporting solutions. Various businesses use it for sales performance analysis and marketing campaign metrics.
The strong focus on customizability makes Looker a flexible choice for businesses that have specific data needs.
Pros and Cons
The main advantage is its flexibility in customizing reports. However, the requirement to understand LookML may pose a challenge for non-technical users.
Tool Eight: Domo
Key Features
Domo provides cloud-based data analytics with an easy-to-use interface. Its notable feature is the platform’s broad capacity to connect with various data sources, enabling extensive integration capabilities.
Domo's real-time data visualizations help improve decision-making processes by allowing organizations to react to live data effectively.
Use Cases
Domo is well-suited for organizations with operations spanning different data sources. Its applications include performance tracking across marketing and sales departments, helping in identifying trends efficiently.
Unique functionalities include its social collaboration feature, allowing teams to share insights in a communicated environment.
Pros and Cons
Domo's strengths lie in its user-friendliness and integration capabilities. However, the cost can be a concern for smaller businesses, as well as the potential complexity in maintaining large datasets.
Tool Nine: TIBCO Spotfire
Key Features
TIBCO Spotfire stands out for its analytics capabilities and data visualization. One of its key features is the built-in analytics engine, which allows for advanced statistical analytics.
Its real-time analytics facilitate dynamic monitoring of business metrics, which is particularly useful in fast-paced environments.
Use Cases
Organizations commonly use Spotfire for operational performance monitoring and to drive decisions based on analyzed data.
An exceptional feature is its ability to ingest diverse data sources, giving users comprehensive insights across different platforms.
Pros and Cons
The analytical depth is a significant advantage. However, the complexity of the interface may overwhelm new users, and some users note a higher learning curve.
Tool Ten: Apache Spark
Key Features
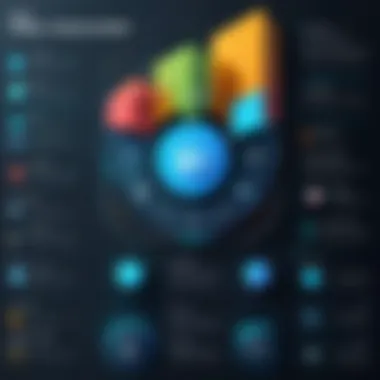
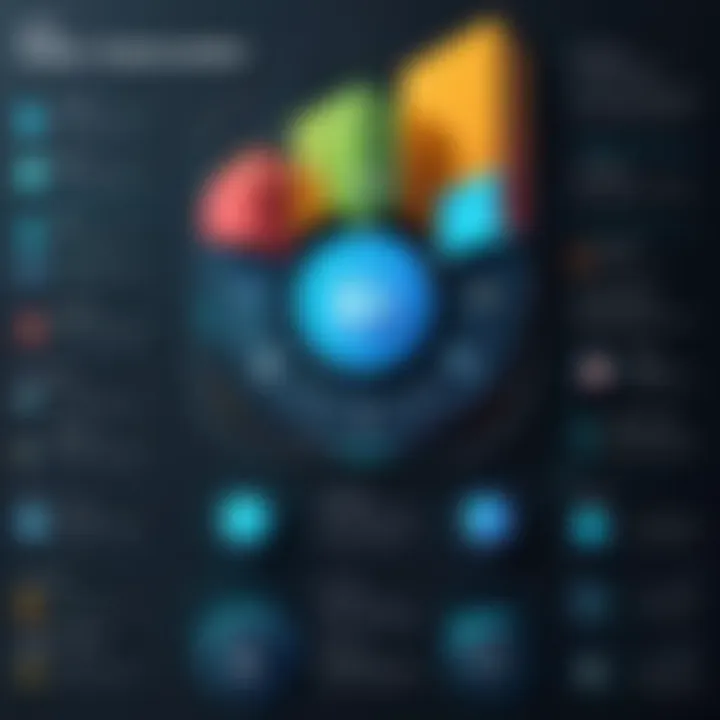
Apache Spark is powerful for big data analytics. It offers speed and processing power, benefiting tasks that require handling large datasets efficiently.
One key characteristic is Spark's ability to perform batch processing and stream processing, making it versatile.
Use Cases
Commonly utilized in data engineering and analytics, Apache Spark serves well in scenarios requiring scalability and performance like machine learning tasks.
Its unique strength lies in its open-source nature, providing flexibility in customization and usage.
Pros and Cons
The major pros include its superior speed and scalability for data engineering tasks. However, it may not be as user-friendly as other tools and often requires deeper technical expertise to manage and implement effectively.
Comparison of the Top Tools
Understanding the differences and similarities among the leading data analytics tools is essential for making informed decisions. This section provides a structured comparison based on the key elements such as functionality, pricing strategies, and user feedback. By evaluating these aspects, professionals can align their choices with their specific needs and organizational goals. The right tool can enhance productivity, improve data insights, and ultimately drive better decision-making processes.
Functionality Comparison
Functionality is a critical metric when assessing data analytics tools. Each tool offers its own set of features designed to cater to varied analytical requirements. Factors to consider include:
- Data Visualization: Some tools, like Tableau, excel at transforming raw data into engaging visual formats, enhancing understanding.
- Data Integration: Compatibility with external data sources. Tools such as Microsoft Power BI and Domo stand out due to their extensive integration capabilities.
- Community and Support: Tools with robust communities can be advantageous. Resources from professional forums or platforms often assist in maximizing the tool's potential.
Ultimately, evaluating each tool's functionality allows users to match features with use cases in their organizations.
Pricing Strategies
Pricing can be a decisive factor for many organizations. The relationship between cost and value should guide selection. Factors related to pricing include:
- Subscription Models: Most tools, including Google Analytics, operate on a subscription basis, which can affect long-term budgeting.
- Free Tiers: Some options, like Looker, offer free tiers or trials that can help users explore functionality before committing.
- Licensing Options: Understanding whether a one-time purchase or a recurring license best fits the organization’s financial strategy is key.
Careful consideration of pricing strategies not only impacts immediate expenses but also long-term investment.
User Feedback Associated
User feedback can provide valuable insights into real-world application and performance of data analytics tools. Key considerations include:
- Reviews: Both positive and negative reviews can highlight strong points and weaknesses. Platforms like Reddit or dedicated forums provide user experiences that can complement official descriptions.
- Use Cases: Real-world examples from users can offer perspectives on how well a tool performed under specific conditions. Sourcing testimonials enhances understanding of practical applications.
- Updates and Innovations: Staying informed on how developers respond to user feedback can indicate a company's commitment to product improvement.
By analyzing user feedback, professionals can better gauge which tool may meet their requirements in a practical setting, mitigating the risks of poor investment.
"Choosing the right data analytics tool demands not only understanding your needs but also how different options align with your operational strategy and budget."
Future Trends in Data Analytics Tools
The field of data analytics is evolving rapidly. Keeping track of these shifts is crucial for professionals who wish to stay relevant and effective. Understanding future trends in data analytics tools helps decision-makers strategize to leverage these technologies for competitive advantage. It is not merely about adopting new tools but also about understanding how these trends shape data methodologies and business models. In this section, we will explore three pivotal areas that are influencing the trajectory of data analytics: integration of artificial intelligence, automation, and increasing focus on data privacy.
Artificial Intelligence Integration
Artificial Intelligence (AI) is profoundly changing how data analytics is performed. AI algorithms enhance data processing capabilities, allowing for deeper insights and more complex analyses. With AI, data can be analyzed in real time, leading to quicker decision-making processes.
- Predictive Analytics: AI's ability to predict trends based on historical data is invaluable. Organizations can anticipate market shifts, consumer behavior, and potential risks with improved accuracy.
- Natural Language Processing: Tools equipped with NLP can make data more accessible. Users can query data in a conversational manner, simplifying complex analyses.
- Machine Learning Models: The incorporation of machine learning allows tools to learn from past experiences. This can lead to improved accuracy over time and more sophisticated analytics.
Incorporating AI into analytics tools not only enhances capabilities but also creates a more intuitive experience for users.
Increased Automation
Automation is another rising trend in data analytics. Many organizations are shifting towards automated data processes. The benefits of automation in data analytics are multifaceted.
- Efficiency: Automated tools save time by performing repetitive tasks without manual intervention. This allows analysts to focus on more strategic tasks.
- Consistency: Data collected and processed automatically is less prone to human error. It ensures accuracy and reliability, which are critical for making informed decisions.
- Scalability: As organizations grow, their data needs increase. Automated solutions can scale easily, handling larger volumes of data without a corresponding increase in human resources.
By automating data collection, processing, and reporting, organizations can significantly boost their productivity.
Growing Importance of Data Privacy
As data analytics tools become more advanced, the importance of data privacy is gaining attention. With regulations like GDPR and CCPA, organizations must prioritize compliance.
- Trust: Protecting user data fortifies trust between businesses and clients. Companies that prioritize data privacy stand to enhance their reputational capital.
- Compliance Costs: Non-compliance can lead to severe financial penalties. Investing in tools that ensure data privacy can mitigate these risks.
- Consumer Awareness: Today's consumers are more aware of their rights concerning data usage. Organizations must adapt their strategies to align with these expectations.
Data privacy is no longer just an afterthought; it is a fundamental aspect of analytics strategies. Companies that integrate robust privacy measures into their analytics processes will likely outperform their peers.
"The integration of AI, increased automation, and a focus on data privacy are not just trends; they are essential components for successful data analytics in the future."
Ending
In summation, the exploration of data analytics tools in this article highlights their growing importance in today's data-driven environment. These tools not only facilitate informed decision-making but also empower organizations to draw meaningful insights from their data. Understanding the nuances of each tool's functionality, user experience, and integration capabilities proves vital for businesses aiming to leverage analytics for competitive advantage.
Importance of Evaluating Data Analytics Tools
The selection of a data analytics tool is far from trivial. It involves a careful consideration of factors such as functionality, usability, and cost. Each tool presents unique features catering to different organizational needs. For instance, Tableau stands out for its data visualization capabilities, while Google Analytics excels in web analytics. Therefore, a comprehensive evaluation enables decision-makers to align their software choices with specific business objectives.
Benefits of Using Appropriate Tools
Using the right analytics tool can lead to significant benefits:
- Enhanced Decision-Making: Quality insights derived through effective analytics can guide strategic choices.
- Efficiency: Automation features save time, allowing teams to focus on higher-value tasks.
- Competitive Edge: Organizations can respond swiftly to market changes by leveraging real-time data insights.
- Improved Collaboration: Many modern tools offer features that promote teamwork and data sharing amongst colleagues.
"Data analytics is not just about the tools, but also about the mindset of turning data into actionable insight."
Considerations for the Future
As organizations continue to recognize the value of data analytics, the landscape will evolve. Firms must stay informed about future trends, including:
- Increasing integration of Artificial Intelligence to automate insights
- The emphasis on data privacy and ethical management of data
- Growing demand for user-friendly interfaces that simplify data analysis for non-experts