Exploring Self-Service Analysis: A Comprehensive Guide
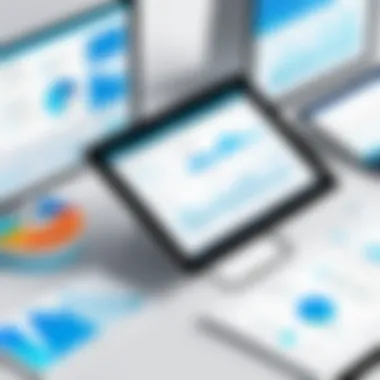
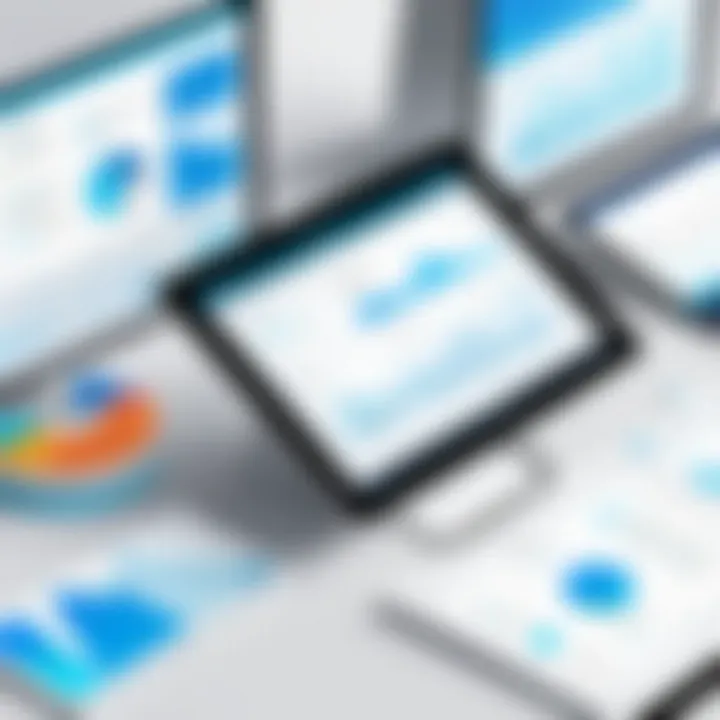
Intro
In today’s data-driven environment, self-service analysis emerges as a pivotal component for organizations looking to enhance their decision-making capabilities. This guide aims to provide a detailed exploration of self-service analysis, focusing not only on its relevance but also on the components that make it a powerful tool.
By understanding self-service analysis, decision-makers can empower users to extract insights from data independently. This independence diminishes reliance on IT departments, leading to more agile and informed decisions. As workplaces evolve, the demand for tools that facilitate self-analysis will only continue to grow.
The following sections will delve into the key software features that are integral to effective self-service analysis. Alongside, we will also look at comparison metrics, as they are essential for evaluating different self-service platforms. Understanding these facets will set the stage for navigating the broader technological landscape tailored for modern enterprises.
Intro to Self-Service Analysis
In today's rapidly evolving data landscape, self-service analysis stands out as a critical capability for organizations aiming to foster a data-driven culture. This approach enables users, often non-technical individuals, to analyze data independently without relying heavily on IT departments. The introduction of self-service analysis enhances the decision-making process, leading to more timely and informed choices.
The relevance of self-service analysis cannot be overstated. It allows businesses to harness the vast volumes of data they generate. With easily accessible tools, users can extract insights that were previously locked away in complex datasets. Moreover, this empowerment leads to innovation and agility. Organizations are now better positioned to respond to market changes, customer preferences, and internal inefficiencies.
Despite its benefits, organizations must consider various aspects before implementing self-service analysis. Establishing a clear strategy for data governance and security is imperative. This ensures that while users have the freedom to explore data, they do so within a framework that protects sensitive information. Additionally, the level of expertise among users can vary significantly. Offering appropriate training and support becomes essential to maximize the utility of self-service tools.
Definition and Context
Self-service analysis refers to the ability of users to access, visualize, and analyze data without direct assistance from IT or data specialists. It encompasses various tools and platforms designed to make analytics user-friendly and intuitive. This democratization of data analytics has transformed how businesses operate, allowing for faster and more effective data-driven decisions.
In this context, self-service analysis serves as a bridge between raw data and meaningful insights, making it a vital component of modern business intelligence strategies. Organizations leverage this capability to create a more engaged workforce that can independently derive actionable insights from data.
Historical Development
The journey of self-service analysis began with the emergence of business intelligence solutions in the early 2000s. Traditional models of analytics were often cumbersome and required extensive technical knowledge. As businesses recognized the need for quicker insights, the demand for more user-friendly tools grew.
Over time, advancements in technology, such as cloud computing and enhanced data visualization techniques, propelled the development of self-service tools. Vendors like Tableau and Microsoft Power BI emerged, providing accessible platforms that catered to a broader audience. This evolution marked a significant shift in how organizations viewed data analytics, steering them towards a model where users could become active participants in the analytical process.
"Self-service analysis is not just about tools; it's about fostering a culture where data is accessible and usable for everyone."
Today's self-service analysis tools continue to evolve, integrating features like machine learning and advanced analytics. This evolution signifies a move towards a more sophisticated understanding of data and its implications across various industries.
Core Components of Self-Service Analysis
In understanding self-service analysis, one must recognize its foundational elements, which serve as a framework for enabling users to independently explore data and derive insights. The core components play a crucial role in shaping the effectiveness of self-service initiatives within organizations. They not only facilitate user engagement but also ensure that the underlying data systems are robust and efficient.
User Interface Design
A well-designed user interface is paramount for the success of any self-service analysis tool. The interface must be intuitive and user-friendly to accommodate a range of skill levels among users. It should allow individuals to navigate through various functionalities without requiring extensive training.
Key aspects include:
- Simplicity: Designs must focus on ease of use, minimizing clutter while providing essential features.
- Responsiveness: The interface should adapt across devices, allowing access from desktops, tablets, or smartphones.
- Exploration capabilities: Users should be able to filter, sort, and visualize data intuitively.
These design considerations significantly empower users. When individuals can access and manipulate data with minimal barriers, they are likely to engage with the tools more frequently and effectively.
Data Accessibility and Integration
Data accessibility is another critical component of self-service analysis. It ensures that users have quick and reliable access to data sources. Moreover, integration capabilities enable these users to bring together data from disparate systems seamlessly.
Organizations need to:
- Prioritize data availability: Data should reside in a centralized repository that users can query easily. This could be through platforms such as Microsoft Power BI or Tableau.
- Implement strong APIs: Application Programming Interfaces facilitate connectivity between different data systems, helping users pull in relevant data without technical barriers.
Effective data accessibility not only enhances user experience but also fosters better decision-making. When users can access the information they need swiftly, they are more likely to derive meaningful insights.
Analytical Tools and Techniques
The range of analytical tools and techniques available to users forms the backbone of self-service analysis. These tools must cater to different analytical needs, allowing users to perform varying levels of complexity in their analyses.
Important features include:
- Predictive analytics: Tools should enable users to apply statistical methods to forecast future trends.
- Visualization options: Users should be able to create and customize dashboards that translate complex data into understandable visuals.
- Collaboration capabilities: Tools need to allow sharing and collaboration among team members, enhancing collective data-driven decisions.
Equipped with effective analytical tools, business users can turn data into actionable insights with greater autonomy. This results in informed strategies that drive organizational growth.
Benefits of Self-Service Analysis
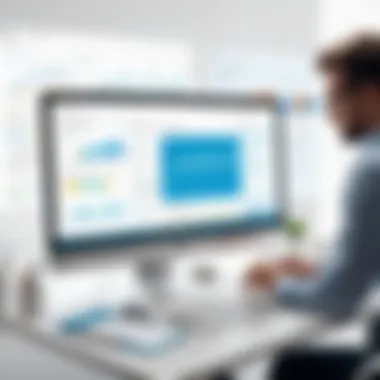
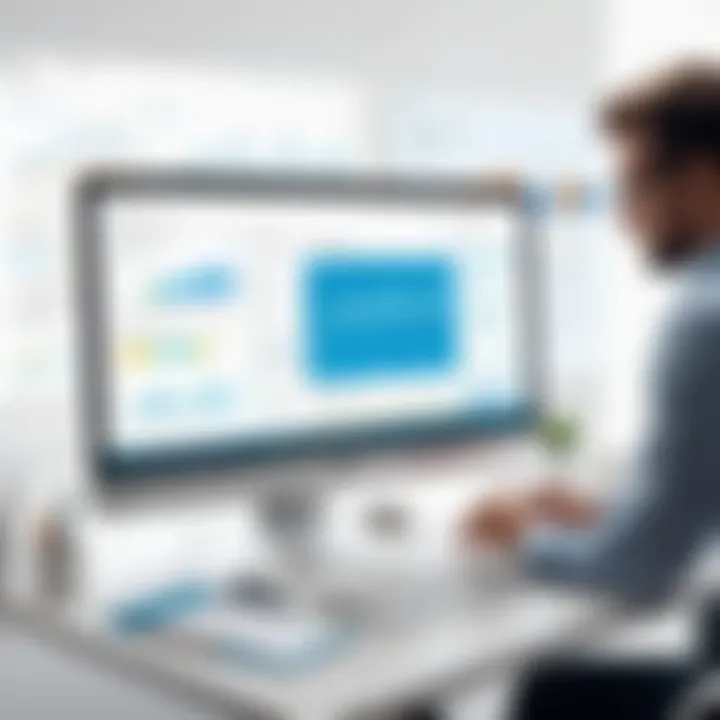
Self-service analysis has rapidly become a core element in modern business intelligence. Its importance lies in its capacity to empower users to take charge of data analysis without relying heavily on IT departments. This shift helps organizations make data-driven decisions with greater speed and efficiency. The benefits of self-service analysis can be categorized into three primary areas: user empowerment, enhanced analytical capabilities, and cost efficiency. Each of these aspects plays a vital role in driving an organization's effectiveness in today’s data-centric landscape.
Empowerment of Business Users
One of the most significant advantages of self-service analysis is the empowerment it provides to business users. Traditionally, users depended on specialized IT analysts to access and interpret data. This often resulted in bottlenecks and delays in obtaining insights due to resource constraints or availability. With self-service tools, users can independently query data and generate reports.
- Accessibility: Users can access real-time data from various sources without needing advanced technical skills.
- Agility: Quick access to insights allows business users to adapt strategies, respond to market changes, and meet customer needs effectively.
- Personalization: Users can tailor reports and dashboards to their specific requirements, enhancing relevance and utility.
Empowerment leads to increased engagement and a growth mindset among employees, fostering a culture of continuous improvement within organizations.
Increased Analytical Capability
Self-service analysis enhances the analytical capabilities of organizations significantly. It enables employees across all departments, not just data scientists or analysts, to perform complex data explorations and gain valuable insights. This democratization of data analysis fosters a data-centric culture.
- Skill Development: Users can develop analytical skills over time by engaging with various tools and data sets.
- Diverse Insights: By allowing employees with different backgrounds to analyze data, organizations can uncover diverse perspectives and innovative solutions.
- Faster Decision-Making: The ability to analyze data on-demand means that businesses can derive insights and make decisions more quickly. As a result, they can adapt to changes in the market or company goals more efficiently.
Through these enhanced analytical capabilities, organizations can maintain a competitive edge in their respective industries.
Cost Efficiency and Resource Management
Cost efficiency is another critical benefit of self-service analysis. Organizations can significantly reduce costs related to data analysis by minimizing reliance on IT resources.
- Reduced IT Burden: Self-service tools alleviate the pressure on IT departments to fulfill frequent ad-hoc requests, allowing them to concentrate on strategic initiatives.
- Lower Training Costs: Investing in user-friendly analytical tools means that extensive training is unnecessary. Users can quickly learn to navigate and utilize these tools effectively.
- Improved ROI: Organizations can achieve a better return on investment by utilizing existing resources more effectively while enhancing productivity.
Overall, integrating self-service analysis into an organization’s operations can lead to substantial cost savings and better resource management, ultimately supporting growth strategies.
"Self-service analysis is not just a trend; it is an essential component of modern business strategy that enhances both efficiency and decision-making processes."
Challenges and Considerations
In exploring self-service analysis, it is critical to acknowledge the inherent challenges and considerations that accompany its implementation. This section addresses key elements such as data governance and security, user expertise and training needs, and the integration with legacy systems. Recognizing these challenges is vital for effectively deploying self-service analysis solutions, thereby maximizing their benefits while mitigating potential risks.
Data Governance and Security
Data governance is a foundational element when implementing self-service analysis. Organizations must establish robust frameworks that ensure data integrity, compliance, and security. When users access and analyze data autonomously, the risk of unauthorized data exposure increases. Therefore, policies should be in place to determine who can access specific types of data, under what conditions, and how that data should be used.
Moreover, implementing technologies such as encryption and multi-factor authentication can enhance data security. Decision-makers need to prioritize the creation of clear guidelines for data handling to prevent breaches. This not only protects sensitive information but also fosters a culture of accountability among users.
"Data security is not just a responsibility of the IT department; it is a shared accountability across the organization."
User Expertise and Training Needs
The success of self-service analysis heavily relies on user expertise. Businesses often face the challenge of ensuring that end users are equipped with necessary skills. Training programs should be tailored to the organization's specific analytical tools and data sources.
A strategic approach to training might include:
- Initial training sessions focused on tool usage.
- Ongoing support systems to address questions and challenges.
- Creating a knowledge base or forum for users to share experiences and solutions.
Investing in user expertise empowers employees, allowing them to leverage data effectively. Thus, the organization can tap into the insights generated by its workforce, ultimately enhancing its competitive advantage.
Integration with Legacy Systems
The challenge of integrating self-service analysis tools with existing legacy systems cannot be overstated. Many organizations depend on outdated software, which may not be compatible with modern self-service solutions. This incompatibility can hinder data accessibility, thus affecting analysis capabilities.
To overcome this, organizations can consider the following steps:
- Assess current systems: Understand the compatibilities and limitations of existing infrastructure.
- Invest in middleware solutions: These tools can bridge the gaps between legacy systems and new technologies.
- Develop a phased integration strategy: Gradually replace or upgrade legacy systems to avoid operational disruptions.
Key Technologies Supporting Self-Service Analysis
Self-Service Analysis is not just a concept; it encompasses a specific technological framework that empowers users with necessary tools and capabilities. Understanding the technologies that support these processes is critical for ensuring their effective implementation. There are three primary technology pillars that enable self-service analysis: Business Intelligence Platforms, Data Visualization Tools, and Cloud Computing and Infrastructure. Each element plays an essential role in providing accessibility, insight, and performance.
Business Intelligence Platforms
Business Intelligence (BI) platforms serve as the backbone of self-service analysis. These tools consolidate, analyze, and present data in a manner that keeps businesses informed. They are designed to extract business insights from vast amounts of data quickly. Prominent examples include Tableau, Microsoft Power BI, and Domo.
The benefits of using BI platforms are numerous:
- User Empowerment: They allow business users to make data-driven decisions without needing IT support.
- Real-Time Insights: BI platforms often provide immediate insights that help organizations respond to market changes and opportunities quickly.
- Scalability: They can easily adjust to accommodate the growing data needs of organizations as they expand.
- Collaboration Features: Many BI solutions enable teams to share insights seamlessly, fostering collaboration.
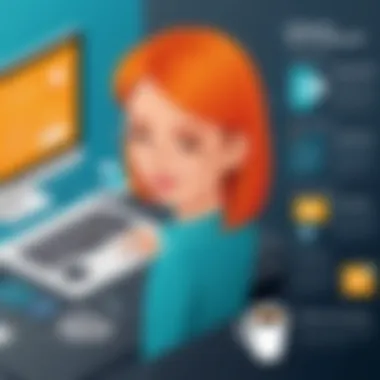
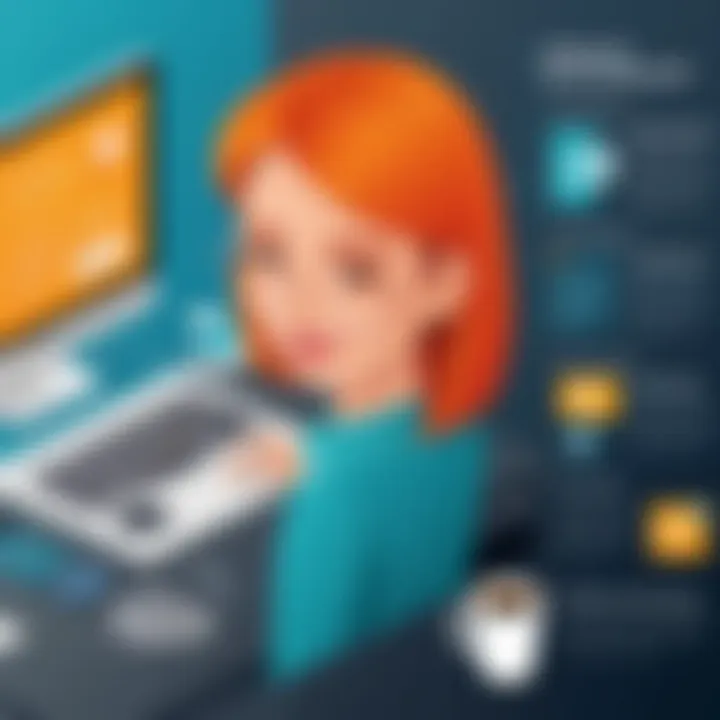
However, businesses must consider data governance for security and integrity. Additionally, user interface design needs to ensure that tools remain accessible to non-technical users.
Data Visualization Tools
Data Visualization Tools are essential for translating complex data sets into visual formats. Tools such as Google Data Studio and Qlik Sense allow users to create intuitive visual representations of data. This helps in quickly grasping trends and patterns.
The advantages of these tools include:
- Improved Comprehension: Visual representation can make complex data significantly easier to understand.
- Engagement: Interactive visualizations keep users engaged while exploring data insights.
- Decision Support: Well-designed graphics help in communicating critical information to stakeholders effectively.
However, a potential pitfall is the risk of oversimplifying data, which can lead to poor decision-making. Users must remain aware of the underlying data and analysis.
Cloud Computing and Infrastructure
Cloud Computing and Infrastructure represent a critical component of the overall ecosystem supporting self-service analysis. Services like Amazon Web Services, Google Cloud Platform, and Microsoft Azure provide the infrastructure needed to store and process data efficiently.
The significance of cloud computing lies in:
- Flexibility: Organizations can scale their resources up or down based on demand, resulting in cost efficiency.
- Accessibility: Cloud services allow users to access data from anywhere, at any time, thus enabling remote work.
- Collaboration: Multiple users can access and analyze data concurrently without conflicts.
Nonetheless, reliance on cloud solutions means organizations must prioritize data security and privacy. Ensuring compliance with regulations like GDPR is essential to avoid legal repercussions.
"Implementing self-service analysis technologies not only enhances decision-making but also catalyzes organizational agility."
Implementing Self-Service Analysis in Organizations
Implementing self-service analysis within organizations is a crucial step towards achieving data-driven decision making. This process allows users to access, analyze, and visualize data independently, thus reducing reliance on IT departments. The significance lies in the empowerment it affords business users, enabling them to derive insights that can influence strategic directions effectively.
However, a successful implementation is contingent on several factors, including assessing the organization’s needs, choosing appropriate tools, and establishing best practices tailored to specific environments. The benefits of self-service analysis are evident in improved speed of data retrieval, enhanced user satisfaction, and ultimately, better business outcomes.
Assessing Organizational Needs
When it comes to assessing organizational needs, it is essential to understand the existing data infrastructure. Identifying challenges such as data silos, inadequate toolsets, or a lack of user training forms a baseline for the implementation strategy.
Key considerations include:
- User Profiles: Different departments may require distinct data insights. For example, marketing may lean heavily on customer data while finance focuses on revenue tracking.
- Current Tools: Evaluate the tools already in place. Are they sufficient for self-service capabilities? If not, what gaps exist?
- Data Quality: Ensuring that the data is accurate and as up-to-date as possible is vital for any analysis. Poor data quality can lead to misleading insights.
Taking time to understand these elements can significantly enhance the effectiveness of self-service analysis.
Choosing the Right Tools
Choosing the right tools plays a pivotal role in the implementation of self-service analysis. The tools must align with organizational needs and user capabilities. Not all tools offer the same level of functionality or ease of use.
Considerations include:
- User Friendliness: The software should have an intuitive interface that encourages user adoption without extensive training.
- Integration Capabilities: Tools need to seamlessly integrate with existing systems. For instance, options like Tableau or Microsoft Power BI provide strong analytical capabilities and can easily connect with various data sources.
- Scalability: As organizations grow, their data needs will also evolve. Tools must be flexible enough to accommodate increased data volume and complexity.
A thoughtful selection process ensures that the chosen tools support the organization's strategic goals.
Establishing Best Practices
Establishing best practices is vital to ensure sustainability and continued success in self-service analysis. Clear guidelines and frameworks help mitigate potential issues.
Best practices should focus on:
- Training and Support: Providing adequate training equips users with the skills needed to utilize the tools effectively. Continuous support encourages users to explore and maximize the capabilities of the tools.
- Data Governance: Implementing a data governance framework can address issues like data privacy and compliance, ensuring users can trust the data they analyze.
- Feedback Loops: Regularly gathering user feedback helps refine tool usage and identifies areas for improvement in processes.
By focusing on these best practices, organizations can create an environment conducive to successful self-service analysis, ensuring that users can leverage data effectively.
Real-World Applications and Case Studies
Real-world applications of self-service analysis provide a tangible understanding of its relevance. This practical framework illustrates how self-service analysis can enhance decision-making processes across various industries. Case studies reveal the real impact on operational efficiency, user empowerment, and strategic insights. As self-service analysis tools become widely adopted, they facilitate better data access and faster response times, which are crucial for maintaining a competitive edge.
Case Study: Retail Industry
In the retail sector, self-service analysis has transformed how companies operate. Organizations like Walmart utilize these tools to enable managers to analyze sales data promptly. This capability helps them identify trends, optimize inventory levels, and enhance customer satisfaction.
Retailers often collect large volumes of data from point-of-sale systems, online sales, and customer feedback. With self-service analysis tools, teams can visualize this data. They generate reports without relying on IT departments, speeding up the decision-making process. For example, a store manager might discover that a particular product is underperforming. With self-service analytics, they can drill down into customer feedback and sales patterns to take immediate action, like altering pricing or launching a marketing campaign.
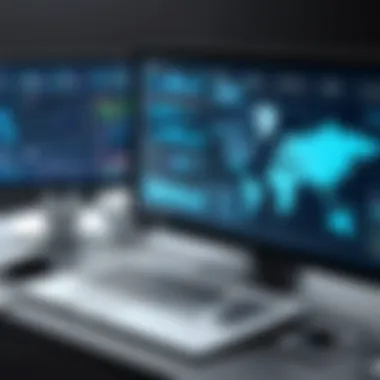
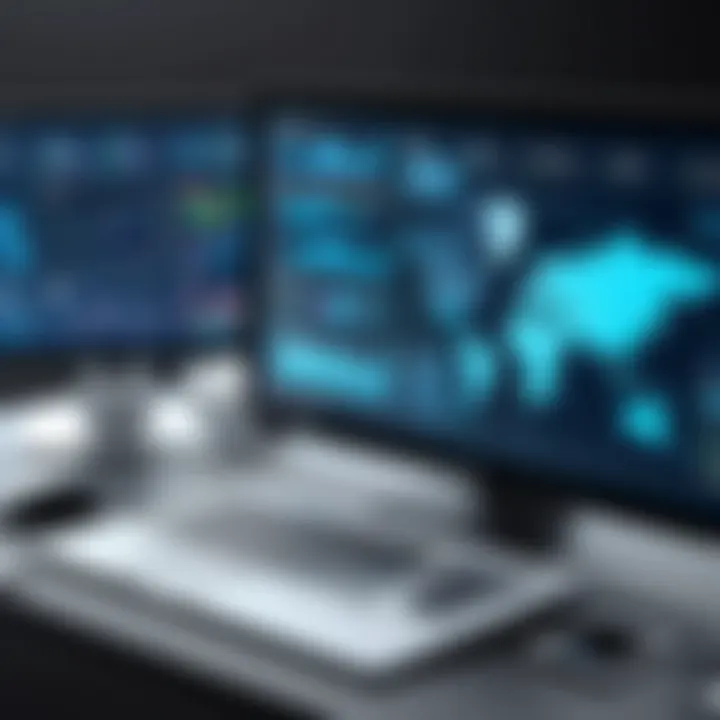
- Benefits:
- Faster response to market trends.
- Improved customer insights.
- Cost savings by minimizing reliance on specialized IT support.
Case Study: Financial Services
The financial services industry has also embraced self-service analysis, enabling companies like Goldman Sachs to manage vast amounts of data effectively. Analysts can access real-time data analytics tools to evaluate market trends, manage portfolios, or assess risks without depending on other departments.
Self-service analysis allows financial experts to create customized dashboards that reflect the metrics most relevant to them. This capability is essential for risk management and customer service. For instance, if a financial advisor notices unusual activity in a client’s account, they can analyze related transaction data. This quick action enables them to provide timely insights and maintain client trust.
- Benefits:
- Real-time access to market data.
- Improved regulatory compliance through better tracking.
- Enhanced client relationships via personalized services.
Case Study: Healthcare
In healthcare, self-service analysis has proven invaluable for hospitals and clinics. Take the example of the Cleveland Clinic, which uses these tools for operational efficiencies. Staff can track patient outcomes, analyze treatment efficacy, and identify bottlenecks in service delivery.
Healthcare providers collect extensive patient data, including treatment history and medication records. With self-service tools, they can visualize this data to improve patient care. For example, identifying which treatments yield the best outcomes helps in formulating better patient management strategies. Additionally, dashboards may reveal trends in patient readmissions, prompting administrators to adjust practices or procedures.
- Benefits:
- Better patient outcomes through data-driven decisions.
- Improved operational efficiencies.
- Enhanced ability to meet compliance standards.
Self-service analysis empowers organizations to leverage their data effectively, enabling quick, informed decision-making across diverse industries.
Future Trends in Self-Service Analysis
Self-service analysis is rapidly evolving as organizations seek agile, data-driven decision-making processes. As users gain more access to analytical tools, it's vital to explore the trends that shape this field. Staying ahead of the curve ensures companies harness the full potential of self-service analysis. Emerging trends point to several areas, notably artificial intelligence, data privacy, and user-centric innovations. Each factor contributes significantly to the effectiveness and security of self-service analytical practices.
The Role of Artificial Intelligence
Artificial intelligence (AI) plays a transformative role in self-service analysis. By automating routine tasks, AI enables users to focus on interpretation rather than manual data manipulation. This shift is essential for enhancing productivity.
Enhanced predictive analytics is one area where AI can make a significant impact. By leveraging machine learning algorithms, organizations can uncover patterns in data that they might not have noticed otherwise. This capacity allows users to make informed decisions based on statistical predictions rather than solely relying on historical data. Moreover, AI can provide real-time insights that greatly reduce response times in fast-paced business environments.
Cloud AI services from companies like Google and Microsoft make integrating these advanced features simpler for users. For many decision-makers, the adoption of AI tools in self-service contexts offers a streamlined approach to extracting value from complex data sets.
Growing Importance of Data Privacy
With the increasing reliance on data, maintaining privacy is a pressing concern. Organizations must prioritize data protection as self-service capabilities grow. Vulnerability to data breaches can lead to significant financial and reputational damage.
Users engaging in self-service analysis can inadvertently expose sensitive information. Therefore, companies must establish robust data governance frameworks. These frameworks should include auditing access controls and ensuring compliance with regulations like GDPR. Companies must educate end-users about data security best practices. Implementing clear guidelines helps minimize privacy risks while promoting a secure analytical environment.
"Data privacy is no longer a luxury; it’s an absolute necessity in the world of self-service analysis."
User-Centric Innovations
User experience plays a critical role in adopting self-service analysis. Innovations focused on user-centric design can enhance how users interact with data. Intuitive dashboards and simplified interactions can reduce the learning curve for non-technical users.
Organizations are increasingly utilizing feedback mechanisms to adapt their tools to meet user needs. Conducting user testing can provide valuable insights into how analytical tools are perceived by different user groups. This approach fosters innovation that consistently aligns with user expectations.
Additionally, the rise of mobile access presents an opportunity for on-the-go data analysis. As more users rely on smartphones, it becomes essential to design apps that provide real-time insight with ease of use. This shift toward mobile platforms demonstrates a significant trend that prioritizes user engagement and accessibility in self-service tools.
In summary, understanding these trends in self-service analysis is critical for organizations that want to stay competitive. The influence of artificial intelligence, the necessity for data privacy, and the emphasis on user-centric design all converge to shape the future landscape of self-service analytics.
Finale
The final section of this article encapsulates the core themes surrounding self-service analysis, underscoring its importance as a significant framework for modern data management and decision-making processes. Self-service analysis democratizes access to data, allowing users to engage with analytical tools directly, which fosters a culture of data-driven decision-making across organizations.
Summarizing Key Insights
Throughout the article, we have explored various facets of self-service analysis:
- User Empowerment: Business users gain autonomy over data analysis, alleviating reliance on IT departments. This leads to faster decision-making and more agile responses to market changes.
- Cost Efficiency: By reducing the need for extensive data analysis teams, organizations can allocate resources more effectively, resulting in significant cost savings.
- Challenges in Implementation: Despite the benefits, issues such as data governance, user training, and system integration must be carefully managed to realize the full potential of self-service analysis.
The integration of self-service tools fosters an environment where insights and data can be available to all relevant personnel, not just data specialists. This shift is crucial in cultivating a data-centric culture where important decisions are backed by solid data analysis.
Final Thoughts on Self-Service Analysis
As organizations increasingly adopt self-service analysis, keeping abreast of best practices and technological advancements will be pivotal. Leaders and decision-makers must prioritize training and support systems to maximize the potential of self-service analysis, ensuring its effective and responsible use.
"Empowering users to analyze data independently not only enhances productivity but also promotes innovative thinking and responsiveness in business."
As we move forward, organizations committed to harnessing the power of self-service analysis are likely to find themselves better positioned to compete in the data-driven economy.