Leveraging Predictive Analytics in Talent Acquisition
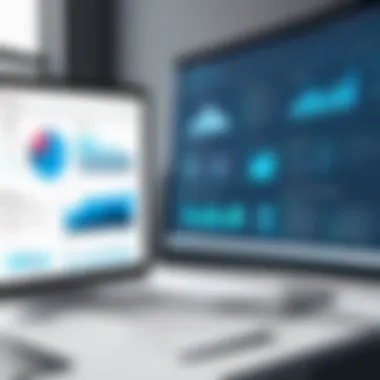
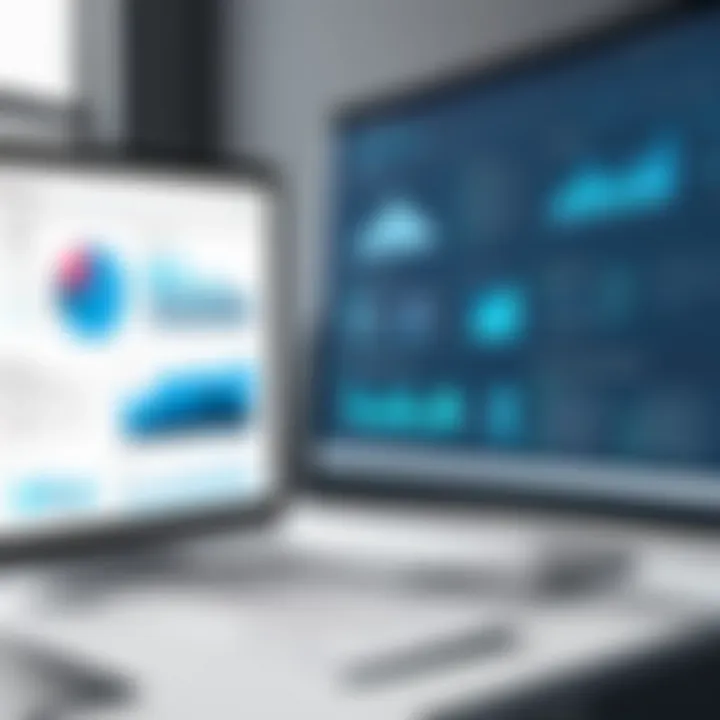
Intro
In the evolving landscape of recruitment, the integration of data-driven strategies has become pivotal. As organizations face a torrent of applications from diverse candidates, predictive analytics rises as a beacon to streamline the talent acquisition process. By utilizing historical data and statistical algorithms, companies can foresee hiring trends, enhance decision-making, and ensure that the right talent is not merely identified, but effectively engaged.
This article will delve into how predictive analytics reshapes talent acquisition, examining impactful methodologies and the multifaceted benefits it offers. Moreover, we shall not shy away from the complexities surrounding this approach, providing a balanced view that informs business professionals and decision-makers alike. Understanding the metrics that underpin effective recruitment can be the difference between mere hiring and strategic talent acquisition.
Key Software Features
When evaluating predictive analytics tools for talent acquisition, certain features stand out as essential.
Essential functionalities
- Data Integration: Seamlessly combining data from various sources such as applicant tracking systems and HR databases ensures that decision-makers have a holistic view of the candidate pool.
- Predictive Modeling: This functionality allows organizations to craft algorithms that predict candidate success based on historical hiring data and performance metrics. By applying these models, hiring managers can improve their selection processes significantly.
- Reporting and Dashboards: Dashboards that visualize data insights in a user-friendly manner help stakeholders easily interpret complex facets of recruitment performance. Visual analytics can often highlight trends and anomalies that are not discernible in raw data.
Advanced capabilities
- Machine Learning Algorithms: Some tools employ sophisticated machine learning techniques to continuously refine predictions based on incoming data patterns. This ongoing learning process enhances the accuracy of hiring predictions over time.
- Natural Language Processing (NLP): Advanced software can analyze the language of resumes and cover letters to assess cultural fit and soft skills, which often play a significant role in candidate success.
"Investing in the right predictive analytics tools for talent acquisition isn't just about hiring faster; it's about hiring better."
Comparison Metrics
Evaluating the performance of predictive analytics software involves several key metrics.
Cost analysis
The cost of implementing predictive analytics in recruitment needs careful consideration. Expenses can range significantly, impacted by factors such as:
- Licensing fees for software
- Implementation and training costs
- Ongoing maintenance and support
Organizations should weigh these costs against the potential for reduced turnover and improved hiring outcomes, which can lead to significant long-term savings.
Performance benchmarks
When it comes to measuring the effectiveness of predictive analytics in talent acquisition, organizations can utilize various benchmarks, including:
- Quality of Hire: Evaluating how well new hires perform and contribute to organizational goals.
- Time to Hire: The duration from initial job posting to successful onboarding.
- Candidate Satisfaction Scores: Gathering feedback from candidates about the hiring process can offer valuable insights into areas for improvement.
These metrics are essential for continuous improvement in recruitment strategies and should be monitored regularly.
Understanding Talent Acquisition
Talent acquisition represents a crucial element in any organization’s success. Its importance goes beyond merely filling vacant positions; it involves strategically attracting, identifying, and selecting the right talent to drive organizational goals. In today’s competitive job market, the ability to connect with skilled candidates—those who fit the company culture and possess the necessary technical capabilities—is paramount.
When organizations prioritize effective talent acquisition, they create a solid foundation for sustained growth and innovation. A well-defined recruitment strategy aligns closely with business objectives and ensures that the right individuals are in the right roles. This approach not only enhances overall productivity but also nurtures employee satisfaction.
Definition and Scope
Talent acquisition encompasses the entire process of recruiting, from identifying staffing needs to onboarding new hires. It is not a one-off task, but rather an ongoing endeavor that adapts to shifting market conditions and organizational requirements.
The scope of talent acquisition extends to various functions, including:
- Workforce planning: Understanding the skills and roles essential for the future.
- Employer branding: Establishing a strong organizational identity in the job market.
- Candidate experience management: Creating a positive and engaging application process.
The Significance of Recruitment
Effective recruitment has far-reaching implications for an organization. It’s the bridge between available talent and the specific needs of the company. Understanding recruitment’s significance unveils various aspects, including how it aligns talent with organizational goals and its impact on overall business performance.
Aligning Talent with Organizational Goals
Aligning talent with organizational goals strikes at the very core of a successful recruitment strategy. This alignment ensures that the skills and aspirations of new hires dovetail with the company’s vision and objectives. One of the key characteristics of this approach is its ability to foster a sense of purpose among employees, enhancing their engagement and retention.
Organizations that successfully align talent with their goals often experience reduced conflicts and higher collaboration among team members. The concept of alignment not only leads to heightened employee morale but also cultivates a culture of accountability where everyone pulls in the same direction.
However, the unique feature of this alignment comes with its challenges. It requires intensive upfront commitment to understand and define both organizational goals and the attributes of the ideal employee. Without this groundwork, mismatches can lead to frustration on both sides, harming relationships and productivity in the long run.
Impact on Business Performance
As the saying goes, "a chain is only as strong as its weakest link." The impact of targeted recruitment on business performance can’t be overstated. Organizations that invest time and resources into obtaining talent that fits their culture often see substantial improvement in their bottom line. A critical aspect of this is that effective recruitment reduces the turnover rates that typically drain resources.
Filling positions with the right individuals can lead to quicker project turnaround and enhanced innovation—because when employees feel they belong, they contribute more. It's not solely about finding candidates with the right skill set; it’s about securing those who add value to the organizational ethos. Key characteristics of a well-implemented recruitment process include efficiency and effectiveness in identifying such candidates.
A unique advantage here lies in analytics. Data-driven insights guide recruitment strategies, allowing organizations to identify patterns of success and the qualities that lead to high performance. Despite the definite benefits, a downside exists: the challenge of quantifying the impact can sometimes leave managers grappling with subjective assessments of performance.
Introducing Predictive Analytics
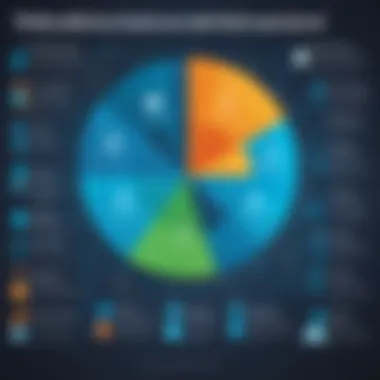
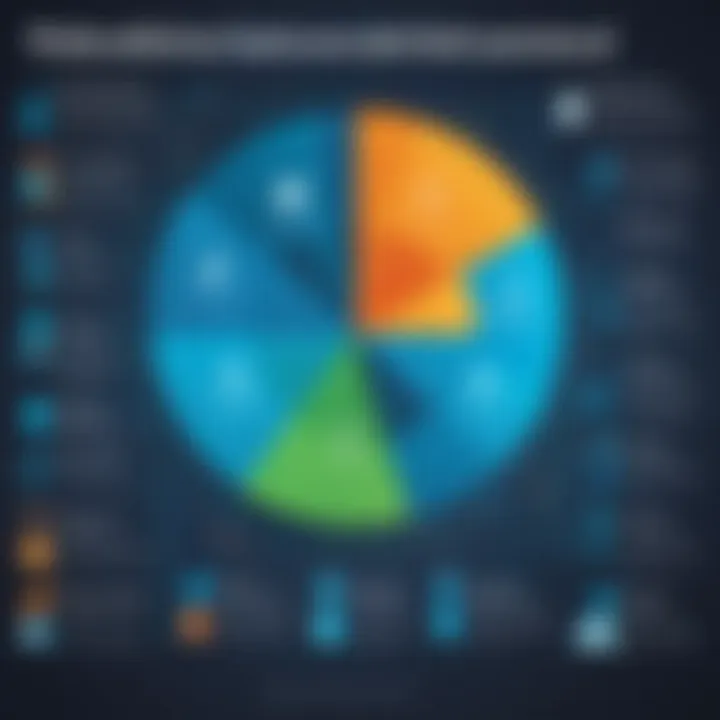
In today’s fast-paced hiring landscape, the need for effective recruitment strategies is more pressing than ever. Predictive Analytics stands at the forefront of this evolution, offering a lens through which organizations can gain significant insights into their talent acquisition processes. By harnessing the power of data, businesses can not only streamline their recruiting but also enhance the accuracy of their hiring outcomes.
Understanding predictive analytics involves recognizing its core function: using historical data to forecast future events. In the realm of talent acquisition, this means analyzing past recruitment successes and failures to better predict the suitability of future candidates. This predictive capability is crucial in identifying patterns that might not be visible otherwise, such as specific traits correlated with high performance or rapid turnover.
Defining Predictive Analytics
Predictive Analytics refers to the methods and technologies that analyze current and historical data to make predictions about future events. It is not just a fancy toolbox but a strategic approach that empowers organizations to make informed decisions about their workforce. The foundation of predictive analytics in recruitment lies in understanding not only who to hire but which candidates are likely to thrive within the company culture and objectives.
Key Components of Predictive Analytics
Predictive analytics is not a singular concept but a composite made of key elements that function together to achieve precise outcomes.
Data Collection
Data Collection serves as the cornerstone for any predictive analytics initiative. To get meaningful predictions, the right data needs to be gathered—and this goes beyond mere resumes. Key Characteristics of effective data collection in talent acquisition involve gathering diverse data points such as applicant qualifications, previous job performances, and even psychometric assessments. This multi-faceted approach enriches the dataset and enables robust analysis.
A unique feature of effective data collection techniques is their capacity to integrate both quantifiable performance metrics and qualitative insights. However, the challenge may arise in dealing with data privacy issues, necessitating adherence to strict regulations like the GDPR in the EU. Balancing thorough data collection while carefully managing candidate confidentiality is a critical balancing act for HR teams.
Statistical Algorithms
Statistical Algorithms form the backbone of prediction models in recruitment. They can sift through vast data pools and identify trends or correlations that may escape even seasoned human eyes. These algorithms can assess numerous variables at once, making them a popular choice in predictive analytics since they can instantly process information that a human analyst might take weeks to evaluate.
The unique attribute of these statistical algorithms lies in their adaptability. Different algorithms can be tailored to fit varying datasets, allowing flexibility in analysis. However, while powerful, they’re only as good as the quality of data inputted; skewed or biased data can lead to misleading predictions, emphasizing the importance of clean, representative data.
Machine Learning Applications
The potential of Machine Learning Applications within predictive analytics is remarkable. It takes the power of statistical analysis a step further by creating models that learn from new data over time. This characteristic allows for continuous improvement in the accuracy of predictions, making it a very beneficial choice for recruitment processes. Hiring managers can adapt their strategies as new trends emerge without starting from scratch.
A defining feature of machine learning applications is their capability to uncover hidden patterns within datasets. Unlike traditional methods, which often rely on human intuition, these systems use algorithms that evolve as new data streams in. However, organizations must also consider the learning curve involved in implementing machine learning tools, as there may be significant resource and training requirements.
"Data-driven recruitment is no longer a futuristic concept; it's a present necessity for organizations looking to thrive."
As we progress in understanding the intersection of technology and talent acquisition, it’s clear predictive analytics plays a pivotal role in shaping modern recruiting strategies. It not only enhances decision-making processes but also optimizes overall efficiency, giving businesses an edge in a competitive hiring landscape.
The Intersection of Predictive Analytics and Talent Acquisition
Over recent years, organizations have increasingly turned to predictive analytics to refine and bolster their talent acquisition strategies. This intertwining of sophisticated data analysis with human resource practices marks a pivotal shift in how companies approach hiring. Here, we’ll delve into various aspects that illuminate the significance of this intersection and its broader implications for businesses seeking to enhance their recruitment efficacy.
Utilizing Data for Better Hiring
Identifying Candidate Fit
Identifying candidate fit is paramount in hiring, as it directly influences employee performance and retention. With predictive analytics, employers can sift through a rich pool of data to pinpoint what traits correlate with success in specific roles. By evaluating past hires and their performance metrics against future applicants, organizations can develop a clearer picture of what a suitable candidate looks like.
One major characteristic of this approach is its reliance on quantitative analysis rather than subjective opinions. This method not only bases decisions on tangible factors but also reduces the risk of unconscious bias creeping into the hiring process. Highlighting culture fit alongside technical skills—derived from extensive data evaluations—makes identifying the optimal candidate an informed choice.
Thus, the unique feature of utilizing this data-driven insight is enhancing the overall quality of hires. While the advantages are prominent, it’s critical to remain cognizant of potential oversights, as excessive reliance on data might diminish the human aspect of hiring.
Projecting Future Performance
Another crucial element of predictive analytics in talent acquisition is projecting future performance. This involves analyzing various indicators, such as past accomplishments and behavioral assessments, to forecast a candidate's potential success within the organization. By studying patterns in high-performing employees, companies can identify similar characteristics in prospective candidates.
This facet of predictive analytics aids in armoring the recruitment process against common pitfalls. The key characteristic here is the ability to create predictive models that gauge possible future outcomes based on existing data. This predictive capability makes it a popular tool for organizations wishing to make informed hiring choices.
A unique feature of projecting performance is its inclusivity of both hard and soft skills, fostering a holistic understanding of what a candidate can bring to the table. However, organizations must tread carefully; overvaluing projections without incorporating qualitative insights could lead to overlooking valuable talent nuances.
Improving the Recruitment Process
Streamlining Applicant Tracking
In any recruitment endeavor, managing the influx of applications can be daunting. Streamlining applicant tracking through predictive analytics can significantly enhance this process, allowing teams to automate key functions. This automation not only expedites the sorting of resumes but also ensures that the selection process leans on data rather than gut feelings alone.
The key benefit here is efficiency. Companies can save countless hours that would otherwise be spent on manual sorting and categorizing. Moreover, utilizing data to track applicant sources can inform staffing strategies, helping organizations allocate resources effectively. One particular advantage is the ability to continuously improve the tracking system—updating algorithms based on hiring outcomes—creating a cycle of evolution in recruitment practices. On the downside, there’s a risk that automated systems may miss out on qualified candidates who don't fit the algorithmic mold, necessitating a balanced approach.
Enhancing Candidate Experience
Finally, enhancing the candidate experience stands as a fundamental advantage of integrating predictive analytics into recruitment. A positive recruitment journey can set the tone for the entire employee lifecycle. By analyzing candidate feedback and satisfaction surveys, organizations can tailor their hiring processes to better meet applicant needs.
This aspect underscores the importance of communication. Candidates appreciate transparency and timely updates during the recruitment process. The key characteristic here is that data-driven insights can help organizations align their processes with the expectations of their potential hires.
While a seamless hiring experience does often lead to better talent acquisition outcomes, it’s crucial for companies to maintain a personal touch amidst automation, ensuring applicants still feel valued and heard. Without this, organizations risk creating an impersonal experience that could tarnish their reputation.
"In an era where data reigns supreme, it’s essential not to forget the human element in recruitment—after all, people hire people."
The interplay between predictive analytics and talent acquisition offers a powerful toolkit for organizations aiming to improve their hiring processes. By grasping these various aspects and integrating them thoughtfully, businesses can cultivate a recruitment strategy that is not only effective but also meaningful.
Benefits of Predictive Analytics in Recruitment
Predictive analytics serves as a transformative force within the recruitment landscape. Talent acquisition has evolved immensely with the sheer volume of data available today. As organizations strive to hire the right candidates, predictive analytics provides a structured approach that integrates data-driven strategies with intuitive hiring practices. This section focuses on how utilizing predictive analytics enhances decision-making, improves cost efficiency, and ultimately boosts recruitment success.
Enhanced Decision-Making
Data-Driven Insights
Data-driven insights represent a core benefit of predictive analytics in recruitment. This approach relies on historical data to forecast which candidates are likely to perform well in specific roles. The beauty of data-driven insights lies in their ability to distill complex information into comprehensible metrics, allowing recruiters to make informed decisions rather than relying solely on gut feelings.
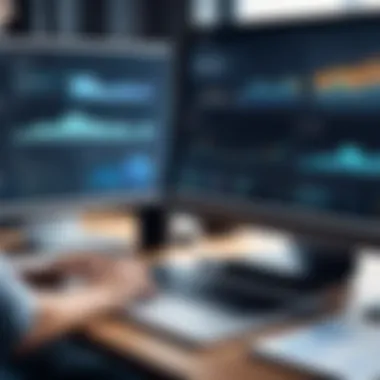
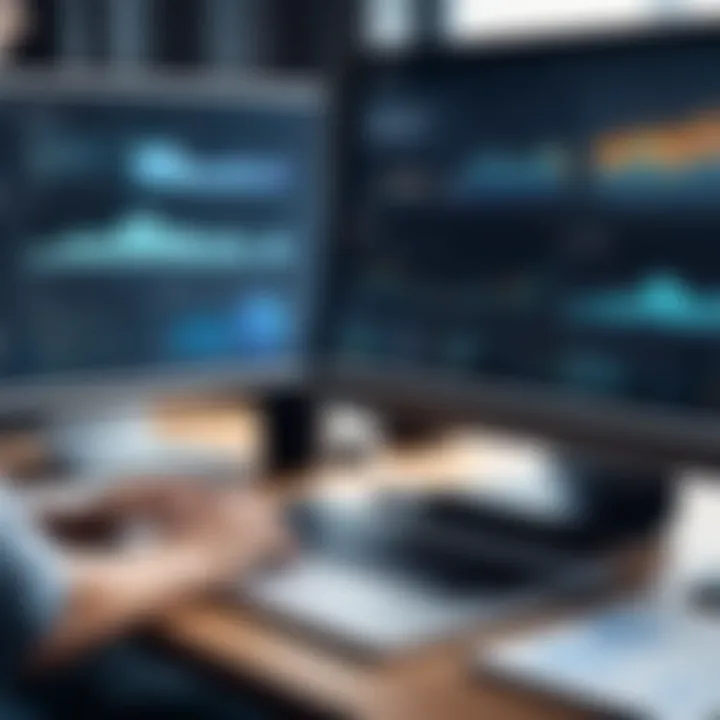
One key characteristic of data-driven insights is their grounded nature in empirical evidence. Organizations leverage various algorithms to analyze past hiring outcomes, employee performance metrics, and demographic data. Consequently, recruiters can pinpoint candidates whose backgrounds and experiences align with what has previously indicated success within the organization.
However, it’s essential to acknowledge one unique feature of data-driven insights: their predictive capacity can sometimes misrepresent a candidate’s potential by overly relying on historical patterns. This can sometimes lead to overlooking fresh talent that deviates from established profiles but may offer innovative capabilities.
In summary, employing data-driven insights can lead to a more structured and reliable process in which the odds of selecting high-performing candidates significantly increases.
Reducing Bias in Hiring
Reducing bias in hiring is another vital area where predictive analytics proves indispensable. Traditional selection processes can inadvertently perpetuate biases, whether based on age, gender, ethnicity, or educational background. By deploying predictive analytics tools, organizations can significantly minimize these biases, leading to a fairer hiring environment.
This method’s key characteristic is its capacity to rely on quantitative data instead of personal judgments that often come with unconscious biases. Predictive models assess job candidates against specific performance indicators rather than relying on subjective lenses. Such a method not only champions diversity but also enhances the process's credibility.
Moreover, the unique feature of reducing bias through analytics serves the dual purpose of opening up candidate pipelines and ensuring that the chosen candidates showcase a broader spectrum of perspectives and skills. While this toolkit champions bias reduction, caution is necessary; relying solely on algorithms could introduce a new type of bias if not carefully managed.
Cost Efficiency
Lower Turnover Rates
A significant benefit accrued from predictive analytics is the potential for lower turnover rates. Higher employee retention translates to substantial cost savings concerning recruitment and training. By utilizing predictive analytics, organizations can uncover patterns correlating job fit, satisfaction, and tenure.
The pivotal characteristic of lower turnover rates is their impact on the overall stability of the workforce. When great fits are identified during the hiring process, organizations often find employees remain longer, fostering loyalty and a harmonious work environment. This stability can reduce the churn costs associated with constantly bringing in new recruits.
A unique feature of this benefit is its cyclical nature; as turnover rates decrease, organizations typically experience enhanced collective morale and productivity, further improving their reputation in the job market. But, it’s important to remember that such analytics can only predict tendencies, not absolutes. Some factors remain unpredictable and require a human element to navigate.
Optimizing Resources
Optimizing resources is where predictive analytics can truly shine within recruitment strategies. Organizations can utilize analytics to allocate time, effort, and finances more effectively. By understanding which recruitment channels yield the best candidates, teams can concentrate their efforts on the most fruitful approaches.
A key characteristic of optimizing resources is its direct correlation to increased efficiency. Recruiters can allocate their time to high-potential candidates while limiting efforts spent on less relevant applications. Moreover, it streamlines the recruitment process, allowing for quicker placements which directly affects organizational performance.
A unique feature of this optimization includes the ability to test various recruitment strategies through data analysis, leading to continuous improvement. However, there can be a downside; focusing solely on optimization might lead to overlooking broader strategic goals in talent acquisition. Hence, while predictive analytics is immensely valuable, organizations must balance efficiency with long-term workforce planning.
Challenges in Implementing Predictive Analytics
Integrating predictive analytics into talent acquisition is not without its hurdles. Organizations often find themselves wrestling with several distinct challenges that can hinder the seamless adoption of these technologies. This discussion is vital, as it addresses key elements that can either make or break the success of predictive analytics in recruitment strategies. Understanding these difficulties allows for more effective planning and execution, thus fostering the adoption of data-driven insights that can significantly improve hiring outcomes.
Data Privacy and Security
Protecting Candidate Information
Protecting candidate information stands as a cornerstone of ethical recruitment practices. It is not just a matter of compliance but also about fostering trust with potential hires. When organizations use predictive analytics, they tend to collect vast amounts of personal data, from resumes to assessment results. The need to safeguard this information cannot be overstated.
One of the unique features of protecting candidate data is the implementation of encryption methods. This technical measure ensures that sensitive information is unreadable to unauthorized individuals. The inclusion of such measures not only secures data but also enhances organizational credibility, making it a popular choice among companies aiming to attract top talent.
However, relying too heavily on technical solutions can sometimes lead to drawbacks, such as the possibility of data breaches if those systems are not frequently updated. Further, organizations must also consider the potential for malicious insider threats, which underscore the need for a comprehensive and multi-layer approach to data protection. Therefore, while protecting candidate information is beneficial, it requires continual oversight and adaptation to ever-evolving technological landscapes.
Compliance with Regulations
Compliance with regulations serves as another crucial element in implementing predictive analytics in talent acquisition. Organizations face a labyrinth of laws and regulations, such as GDPR and CCPA, that dictate how personal data should be managed. Adhering to these rules is of utmost importance, as non-compliance can lead to severe penalties and reputation damage.
The characteristic of compliance with regulations often involves regular audits and assessments of data handling procedures. This ensures that all aspects of data collection and usage are in line with legal requirements. Such a proactive approach is not just critical for avoiding fines but is also beneficial in establishing a sense of accountability within the organization.
Nonetheless, compliance can be a double-edged sword. The rigorous nature of these regulations can sometimes slow down the hiring process or lead to increased administrative burdens that could frustrate HR teams. Still, by embedding compliance into the predictive analytics framework from the ground up, companies can mitigate risks while maintaining efficient recruitment practices.
Integration with Existing Systems
Technology Compatibility
Technology compatibility plays a significant role in the successful integration of predictive analytics in recruitment. Organizations often have existing systems that need to communicate effectively with new predictive tools. Ensuring that these platforms work together harmoniously is crucial for optimizing workflows and data accuracy.
The key characteristic of technology compatibility is interoperability, which allows different systems to share data seamlessly. This is a beneficial aspect for businesses looking to enhance their recruitment capabilities without having to completely overhaul their existing setups.
Nevertheless, achieving this level of compatibility is not always straightforward. Organizations may struggle with legacy systems that are resistant to integration with modern analytics tools. Additionally, the costs associated with upgrading or replacing outdated software can be a significant hurdle. Therefore, balancing the need for compatibility with budget constraints is a critical consideration for many organizations.
Overcoming Resistance to Change
Overcoming resistance to change is often an overlooked but fundamental factor in the implementation of predictive analytics. Employees may be reluctant to adopt new technologies, perceiving them as threats to their jobs or simply preferring the ways things have always been done. This resistance can undermine even the best efforts to leverage analytics effectively.
A key characteristic of overcoming this barrier involves proactive communication and training. By actively engaging employees in the transition process and providing comprehensive training programs, organizations can cultivate a culture receptive to change. This approach not only enhances acceptance but also instills a sense of ownership among employees.
Yet, it’s important to note that change management is not a one-size-fits-all solution. Different teams may have different concerns, and addressing these requires a tailored approach. Failing to do so can result in disengagement, which ultimately detracts from the potential advantages of employing predictive analytics in the recruitment process.
In summary, recognizing and addressing these challenges in implementing predictive analytics is essential for organizations aiming to revolutionize their talent acquisition strategies. By tackling issues of data privacy, compliance, technology compatibility, and employee acceptance, companies can pave the way for a more data-driven and effective recruitment process. Thus, while hurdles exist, they are not insurmountable.
Case Studies and Real-World Applications
Exploring case studies and real-world applications provides a vital compass for those looking to implement predictive analytics in talent acquisition. It allows organizations to learn from others' successes and missteps in applying these data-driven approaches. These narratives illuminate the multifaceted benefits of effectively harnessing data while also revealing the potential roadblocks that need to be navigated.
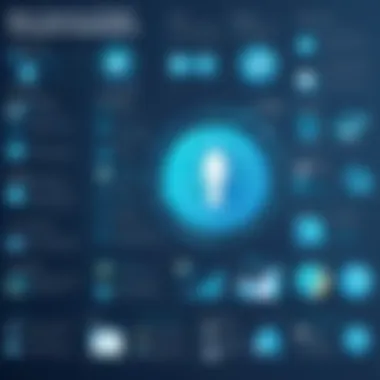
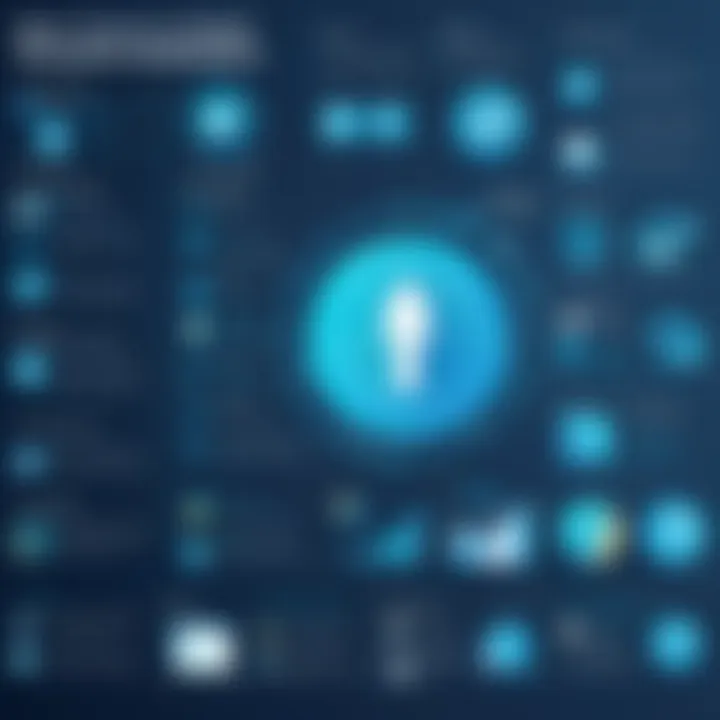
In the realm of talent acquisition, leveraging real-world examples not only builds credibility but also showcases practical applications that can inspire and guide decision-makers. Case studies shed light on how companies have transformed their recruitment strategies, driving home the significance of predictive analytics in making informed hiring decisions.
Successful Implementations
Examples from Industry Leaders
When we investigate examples from industry leaders, a shining illustration is Google. This tech giant has mastered the art of data-driven hiring, employing predictive analytics to refine their recruitment strategy. Utilizing data from previous hires, Google has engineered a hiring algorithm that assesses candidates for cultural fit and skill competency efficiently. The unique characteristic of Google’s approach lies in its comprehensive analysis of employee performance metrics over time, allowing the company to refine its hiring criteria continuously. This method stands as a beneficial choice as it not only streamlines the hiring process but also delivers a more fitting candidate pool, improving retention rates.
Another notable case is IBM, which has implemented an advanced predictive analytics tool known as Watson. This initiative analyzes a vast array of data points to assist recruiters in identifying high-potential candidates. The distinct advantage of IBM's approach is its focus on cognitive technology, making the hiring process less prone to human bias. This positions IBM as a pioneer in this arena, illustrating the vast potential that predictive analytics has for organizations looking to enhance employee selection.
Results Achieved
The results achieved through these implementations reflect the positive impact that predictive analytics can have on talent acquisition. For instance, studies have shown that organizations like Google have witnessed a significant decrease in both time-to-hire and employee turnover. The key aspect here is not just the speed of hiring but also the quality of hires, which translates into increased productivity and morale within teams. These outcomes underline why employing predictive analytics is a popular strategy among leaders.
Moreover, IBM reported that utilizing Watson for recruitment reduced the time spent sorting through CVs by up to 30%. This efficiency allows recruiters to focus on high-value tasks, promoting a more strategic approach to hiring. However, one should note that such technology can initially come with a steep learning curve and significant investment, necessitating a calculated approach to its adoption.
Lessons Learned
Pitfalls to Avoid
Even as organizations strive toward integrating predictive analytics, it’s essential to identify pitfalls to avoid. One crucial aspect is being overly reliant on data without understanding its context. For instance, a company that only relies on algorithms without human oversight risks missing out on valuable insights that cannot be quantified. In this regard, it is essential to maintain a balanced approach, ensuring that data-driven analysis doesn’t overshadow the human element that plays a significant role in the hiring process.
Additionally, companies should be wary of inadequate data governance. Poor data quality can lead to misguided conclusions, which might actually harm their recruitment efforts. This key characteristic is critical because neglecting it can waste resources and time, indicating why it’s a fundamental consideration.
Best Practices
To harness predictive analytics effectively, there are some best practices one can adhere to. Firstly, fostering a culture of continual learning and adaptation significantly contributes to better outcomes. Organizations should regularly revisit and revise their predictive models based on new data and shifting market conditions. Such an approach ensures that they remain aligned with their hiring objectives and the evolving landscape of talent acquisition.
Another best practice involves building interdisciplinary teams that incorporate HR professionals, data scientists, and business leaders. This collaborative effort enhances understanding and integration of analytics within the recruitment strategy. The unique benefit here is that it enables companies to harness diverse perspectives, ultimately leading to more innovative solutions in the hiring process.
In summary, the application of predictive analytics within talent acquisition is supported by a wealth of successful case studies, highlighting the journey taken by industry leaders. These narratives serve both as a guide and a cautionary tale, reminding organizations of the potential rewards and risks associated with data-driven hiring. By embracing these lessons and insights, decision-makers can effectively navigate the realm of talent acquisition and optimize their recruitment processes.
Future Trends in Talent Acquisition and Predictive Analytics
The landscape of talent acquisition is continually shifting, largely influenced by advancements in predictive analytics. As organizations embark on the journey to streamline their recruitment processes, understanding these future trends becomes crucial. By exploring emerging technologies and the evolving workforce dynamics, businesses position themselves to navigate this transformation effectively. The essence of these trends lies not only in adopting new tools but also in grasping how these innovations can bolster hiring strategies and outcomes.
"The ability to predict hiring needs before they manifest can be a game changer for businesses navigating the talent acquisition maze."
Emerging Technologies
Artificial Intelligence
The role of artificial intelligence (AI) in talent acquisition is profound. At its core, AI enables smoother, data-driven decision-making processes that were previously reliant on intuition or anecdotal evidence. This technology excels in automating repetitive tasks, which not only saves time but also improves the accuracy of recruitment efforts. A key characteristic of AI is its ability to analyze vast amounts of data quickly.
Organizations are leveraging AI tools to sift through resumes, assess candidates’ skills, and even predict long-term cultural fit in the workplace. The unique feature of AI lies in its predictive modeling capabilities—organizing historical hiring data to forecast future talent needs effectively. However, potential disadvantages include reliance on data integrity; biased data can lead to biased outcomes, a factor organizations need to navigate carefully.
Natural Language Processing
Natural Language Processing (NLP) is another pillar of modern recruitment strategies. By breaking down human language, NLP helps organizations interpret unstructured data such as job descriptions or candidate communications, leading to more efficient candidate evaluation. A hallmark feature of NLP is its ability to understand context, enhancing the nuance in how job requirements are described and assessed against applications.
For example, an NLP tool can analyze job postings across multiple platforms, ensuring consistent language that attracts the right talent. While NLP brings many advantages—improving candidate interaction and understanding—there are challenges too. It requires continuous learning and adaptation to remain effective, which might necessitate ongoing investment and adjustment as language and industry jargon evolve.
Changing Workforce Dynamics
Remote Work Trends
Remote work is reshaping talent acquisition strategies worldwide. The shift to virtual working environments has opened a broader playing field for talent, allowing organizations to tap into skill sets previously limited by geographical constraints. A notable characteristic of remote work is flexibility; not only do employees benefit, but companies also expand their candidate pool tremendously.
Incorporating remote work trends enables organizations to access niche skills that may not have been available locally. However, this trend demands an evaluation of how performance is monitored and how team dynamics are facilitated, since traditional methods might not apply effectively in virtual settings.
Diversity and Inclusion Efforts
Diversity and inclusion efforts are becoming vital for modern organizations, particularly in talent acquisition. The growing recognition of the benefits that diverse teams bring to innovation and problem-solving is a key reason these efforts are emphasized now more than ever. One significant characteristic in this regard is the emphasis on equity in hiring practices to ensure all candidates are evaluated equally, regardless of background.
Unique to this trend is the role of predictive analytics in identifying gaps in diversity and aiding organizations in setting actionable goals. Although incorporating diversity initiatives can be resource-intensive, the strategic long-term advantages—like improved employee satisfaction and heightened company reputation—far outweigh these costs.
In summary, as predictive analytics entwines with emerging technologies and changing workforce dynamics, organizations that embrace these trends will likely emerge ahead in the competitive landscape of talent acquisition. The agility to pivot and adapt not just leaves a mark but builds an adaptive organization poised for future challenges.
Epilogue: The Future of Talent Acquisition
As we wrap up our exploration of predictive analytics in talent acquisition, it’s vital to recognize the transformative impact this integration has on how organizations attract and select talent. Talent acquisition is no longer merely about filling positions; it’s about strategically aligning recruitment practices with the overarching goals of a business. By leveraging data analytics, companies can make informed decisions that enhance their hiring effectiveness and ultimately drive better business outcomes.
Predictive analytics not only streamlines recruitment but also improves candidate experience by providing insights that refine the screening process. In the years to come, those organizations that adopt these analytical tools will likely claim a competitive edge in securing top talent.
Key Takeaways
- Data-Driven Evolution: Talent acquisition has evolved from a process based mainly on intuition and past experiences to one firmly rooted in data analysis. Understanding trends and patterns in hiring processes helps organizations predict candidate success better.
- Enhanced Hiring Outcomes: Companies employing predictive analytics can achieve significantly lower turnover rates, as they can match candidates more precisely with roles that suit their skills and values.
- Continuous Adaptation: The adoption of new technology and methodologies, such as AI and machine learning, is not a one-off task but a continuous journey. Businesses must be willing to adapt and refine analytical frameworks for sustained success.
- Focus on Diversity and Inclusion: Predictive analytics provides a clearer picture of recruitment efficacy, allowing organizations to identify potential biases and work towards a fairer hiring process, which improves overall workplace diversity.
Strategic Recommendations
- Invest in Training: Equip your HR teams with the necessary skills to interpret data and utilize predictive analytics tools effectively. Understanding these systems is critical for strategic decision-making.
- Utilize Comprehensive Data Sources: Go beyond standard metrics; incorporate various data points from internal and external sources to build a holistic view of your hiring landscape.
- Prioritize Candidate Experience: While focusing on data-driven decisions, do not forget the human aspect. Ensure that the processes remain respectful and engaging for candidates throughout their recruitment journey.
- Continuous Evaluation: Periodically assess the predictive analytics tools and metrics in use. An agile approach ensures that your strategies remain relevant in a constantly evolving market.
- Foster a Culture of Data-Driven Decision-Making: Encourage the entire organization to adopt a data-centric mindset. Engaging all levels of leadership in analytics-driven discussions can cultivate an environment ripe for insights and innovative recruitment strategies.
By implementing these recommendations, organizations can nurture a robust talent acquisition strategy that will not only meet current demands but is also adaptable to future changes in the workforce landscape. The future of talent acquisition lies in the intelligent application of predictive analytics, ensuring organizations are positioned for sustained success.