Essential Data Integration Tools for Modern Businesses
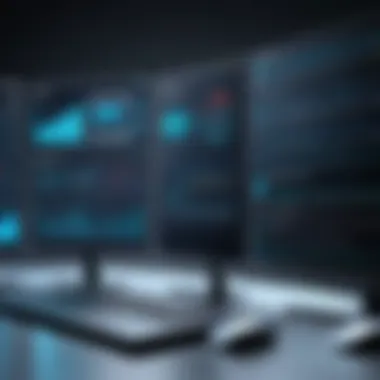
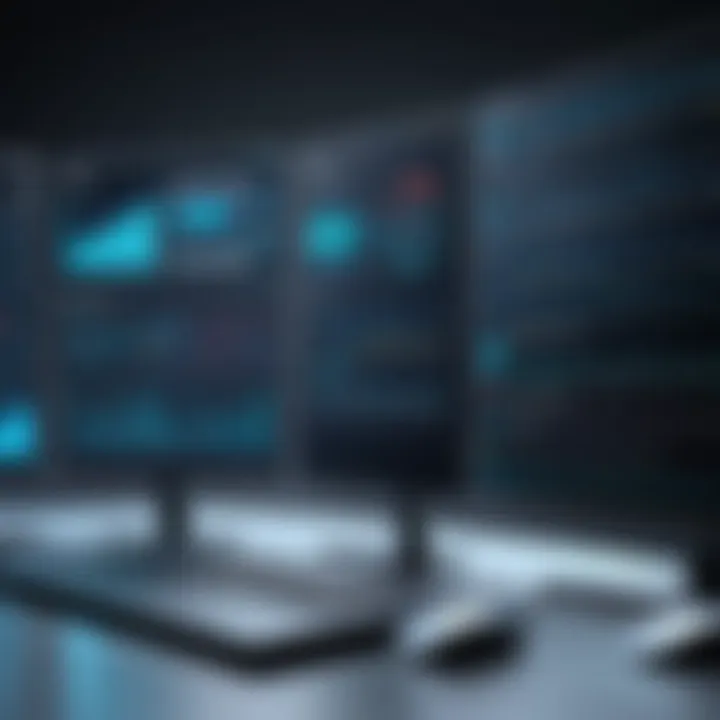
Intro
In today’s data-driven world, integrating different types of data from diverse sources is crucial for the success of any organization. Data integration tools have become the backbone of this vital process, enabling businesses to combine their data into a cohesive format that drives insights and decision-making. But what exactly makes these tools essential?
By leveraging various functionalities, these tools can streamline the process of data collection, transformation, and storage. The complexities of modern data landscapes call for solutions that not only fit specific organizational needs but also enhance operational efficiency across departments. For decision-makers, IT professionals, and entrepreneurs, understanding this landscape is no small feat.
This guide provides an extensive exploration of data integration tools, diving into key software features, comparison metrics, and real-world applications that serve a variety of organizational facets. Additionally, it aims to equip professionals with the insights they need to make informed choices in a sea of options.
Let’s embark on this journey to decipher the intricate web of data integration tools and their role in shaping data management practices.
Key Software Features
When evaluating data integration tools, understanding the key features is paramount. Here are the essential functionalities and advanced capabilities that you should consider:
Essential functionalities
- Data Connectivity: The ability to connect with a variety of sources, from databases, APIs, cloud services to flat files. This ensures seamless data access.
- Data Cleansing: Tools should provide mechanisms to identify and rectify inaccuracies in data. After all, garbage in means garbage out.
- ETL (Extract, Transform, Load): A fundamental process for gathering data from multiple sources, transforming it into a desired format, and loading it into a designated data store.
Advanced capabilities
- Real-time Data Processing: Many contemporary tools can process data as it arrives, offering insights and analytics without delay. This is key for businesses needing agile information flows.
- Visual Workflow Design: A user-friendly interface for designing data flows visually. This helps technical and non-technical staff alike in understanding and managing the data pipeline.
- Scalability: As data volumes grow, the capability to scale up resources is essential. Tools that can handle increasing data loads without significant rework save invaluable time and resources.
"Data integration is not just about merging information but making it meaningful for decision-making." - Industry Expert
Comparison Metrics
Once you have a grasp on the features, the next step is to delve into specific comparison metrics that help gauge the efficacy and suitability of these tools for your organization.
Cost analysis
- Initial Investment: Look beyond just the sticker price. Consider setup fees, training, and infrastructure adjustments that may be necessary before thing get rolling.
- Total Cost of Ownership: Evaluate the long-term costs that might include subscription fees, maintenance, and potential scalability costs over the lifecycle of the tool.
Performance benchmarks
- Data Processing Speed: Benchmarks for processing speed should be carefully considered, particularly for businesses that operate on tight deadlines.
- Uptime Reliability: Consistent uptime is vital. It would be prudent to inquire about the track record of uptime and available support in case of downtimes.
Armed with this comprehensive guide, professionals can navigate through the many data integration options, empowering themselves to make effective choices that positively impact operational efficiency.
Preface to Data Integration
In today’s fast-paced digital environment, data integration has become a linchpin for organizations striving to harness the full power of their data assets. At its core, data integration refers to the process of combining data from various sources to provide a unified view. This ensures that regardless of where the data resides—be it on-premises databases, cloud services, or third-party applications—decision-makers can access a consolidated dataset. The significance of mastering data integration cannot be overstated, as it shapes not just IT infrastructure, but influences overall business strategy and operational agility.
Definition of Data Integration
Understanding what data integration means is crucial for effectively implementing it. Simply put, data integration consolidates data from diverse sources, providing users with a holistic view. This process encompasses several activities, including data extraction, transformation, and loading into a final destination, typically a data warehouse or another form of storage. While many might think of it just as a technical task for IT teams, it’s very much a crucial aspect of business intelligence and analytics. Data must flow seamlessly across departments to foster intelligence-driven decisions.
Importance of Data Integration in Modern Business
The significance of data integration for modern businesses can be likened to a well-tuned orchestra. Each instrument may produce sound individually, but together they create a symphony that resonates far louder and clearer. Here are some key areas where data integration holds immense value:
- Improved Decision-Making: Integrated data offers a comprehensive view, allowing managers and executives to make informed choices rather than rely on fragmented information.
- Enhanced Efficiency: Streamlining data processes eliminates silos, reducing time spent on data reconciliation, and allowing teams to focus on core activities that drive value.
- Better Customer Insights: By integrating customer data from various touchpoints, businesses can develop personalized marketing strategies and enhance customer experiences—turning data into a strategic asset.
- Regulatory Compliance: A cohesive view of data helps ensure that organizations meet industry regulations, keeping them on the right side of compliance issues.
"Integration is not just about technologies; it is about streamlining processes and making data work for us!"
In summary, the essence of integrating data can’t be overlooked in today’s data-driven world. It’s the foundation on which successful analytics and decision-making are built. Understanding its fundamentals sets the stage as we delve deeper into the key concepts, tools, considerations, and best practices surrounding data integration.
Key Concepts in Data Integration
Understanding the key concepts in data integration is essential for anyone diving into the landscape of data tools. It's not just about moving data from point A to point B; it's about ensuring that the data is accurate, accessible, and useful for decision-making.
Types of Data Integration
- Batch Integration
This type involves collecting and storing data over a specified period before processing it in a single batch. Think of it like gathering all your receipts at the end of the month to reconcile your budget. This approach can be cost-effective, but it may lead to outdated information if real-time updates are not a priority. - Real-Time Integration
This method allows data to flow continuously, ensuring that it remains current. A classic example of real-time integration is a stock trading platform where prices update every second. This type of integration is crucial for industries that rely heavily on instant data accuracy, such as finance and e-commerce. - Unidirectional vs. Bidirectional Integration
Unidirectional integration means data flows in one direction—from source to destination—while bidirectional integration allows data to flow both ways. Think of an online shopping site where customer information updates occur in your inventory system (unidirectional) versus a two-way sync where both systems update customer preferences dynamically. - Application Integration
In this scenario, various applications are interconnected to share data seamlessly. Imagine a customer relationship management (CRM) system that integrates with email marketing and an e-commerce platform. This integration allows for a single view of the customer across different systems, resulting in cohesive marketing strategies. - Data Warehouse Integration
This focuses on combining data from multiple sources into a central repository, often called a data warehouse. It plays a pivotal role for businesses that want to analyze historical data over time. Integrating sales, marketing, and customer service data into a single view can provide invaluable insights for strategic planning.
Understanding these types is just the tip of the iceberg. Each type offers distinct advantages depending on organizational needs, scale, and industry requirements. Choosing the right type can significantly affect operational efficiency and decision-making quality.
Common Challenges in Data Integration
Even with the right tools, data integration isn't a walk in the park. Here are some common hurdles that businesses encounter:
- Data Silos
Organizations often find themselves with isolated data pockets scattered across different departments. This leads to inconsistent information, making it challenging for decision-makers to retrieve a complete picture of the situation. - Data Quality Issues
Poor data quality is a persistent problem. Inaccurate, outdated, or duplicate data can compromise analysis and affect outcomes. A business might find itself relying on faulty insights, leading to poor strategies and decisions. - Integration Complexity
The variety of data formats, sources, and systems can create a tangled web. When multiple systems don’t communicate effectively, integration can quickly become a frustrating maze. - Security Risks
As businesses integrate more data sources, they face an increased risk of data breaches. Ensuring data is protected during integration must be a priority. Strong security measures need to be in place to safeguard sensitive information. - Compliance Challenges
Data regulations, such as GDPR, can complicate integration efforts. Organizations must ensure any integrated data complies with these laws, which may require diligent oversight and evaluation.
Overcoming these challenges is crucial. The path to successful integration often involves a blend of strategic planning, choosing the right tools, and cultivating a culture that values data consistency and quality.
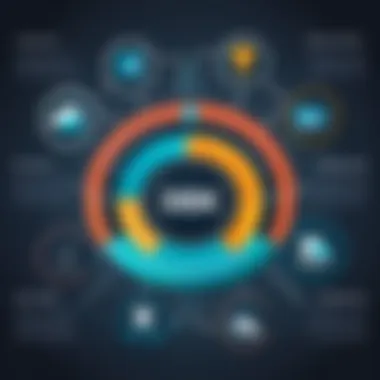
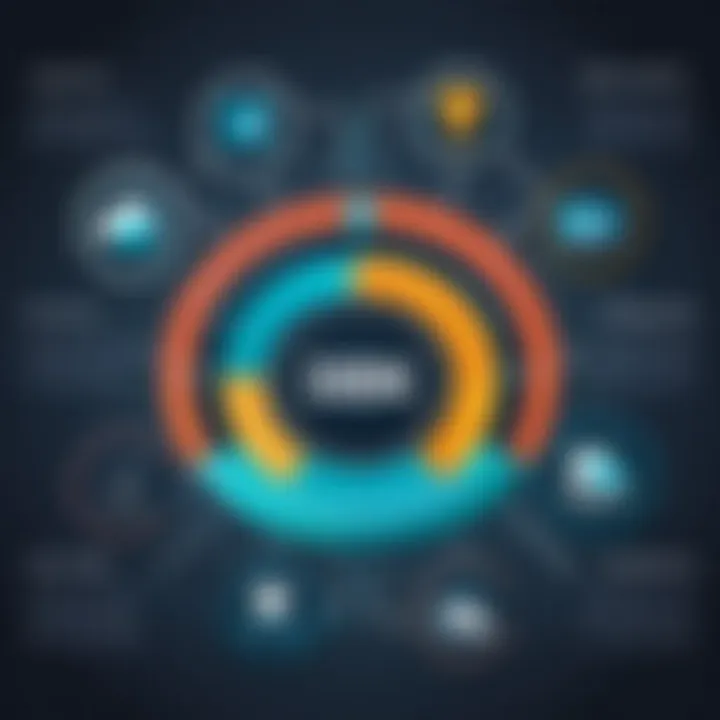
"Integrating data is more than just technical work; it's about creating systems that provide true business value."
In summary, mastering the core concepts and common challenges in data integration is fundamental to leveraging the full potential of your organizational data. This lays a strong foundation for selecting the right tools and strategies that align with your business goals.
Criteria for Selecting Data Integration Tools
Choosing the right data integration tool can feel like trying to find a needle in a haystack, particularly given the number of options available today. But a well-informed decision ensures that organizations can efficiently manage their data, increase productivity, and ultimately drive better business outcomes. There are several critical criteria to consider when selecting a data integration tool, notably scalability, ease of use, and support.
Scalability of Solutions
Scalability refers to a tool's ability to handle increasing amounts of data without sacrificing performance. As organizations grow, so too does the volume of data they generate. A robust data integration tool should not only accommodate current data needs but also be adaptable to future growth.
- Performance Under Load: Whether it's a suddenly massive influx of customer data or the increased complexity of data sources, the tool must maintain quick and reliable data processing.
- Flexible Architecture: Look for modular designs that allow for easy addition of functionality or services. Integration capabilities should enable the tool to communicate seamlessly with other systems.
- Cost-Effectiveness with Growth: Some tools may offer lower costs upfront but require expensive upgrades or redesigns as your data requirements expand. It’s crucial to factor in long-term costs instead of just initial pricing.
Ease of Use and Implementation
A tool that takes a PhD to understand won’t do anyone any good. Organizations want solutions that are intuitive and straightforward to implement. The time it takes to set up the tools can significantly impact productivity and resource allocation. Here are some considerations:
- User Interface: A clean, intuitive interface allows users to navigate with ease, reducing the learning curve drastically.
- Deployment Options: Look for tools that allow easy deployment, whether on-premises, in the cloud, or a hybrid model, to suit your organization’s needs.
- Integration with Existing Systems: The chosen tool should easily integrate with other software and databases already in use to ensure a seamless transition and minimize disruptions.
Support and Community
Having robust support for any software is akin to having a life jacket when you’re on a boat. If something goes wrong, a reliable support system can mean the difference between chaos and smooth sailing. Additionally, the community around the product is vital for learning and troubleshooting.
- Quality of Customer Support: Look for vendors who provide clear support channels—whether through phone, chat, or email. The response time and resolution process should be efficient and user-friendly.
- Documentation and Training Resources: Comprehensive user manuals, tutorials, webinars, and training sessions can empower users to make the most of the tool.
- Active Online Communities: A vibrant forum or user group offers a wealth of information and solutions from other users. Places like forums on Reddit or even Facebook groups dedicated to specific tools can provide insights not found in formal documentation.
"Selecting the right data integration tool is not about finding the biggest name in the game, but understanding which one is the best fit for your specific business needs."
Overview of Data Integration Tools
In today’s fast-paced digital landscape, businesses are swimming in vast seas of data. Managing this data often feels like herding cats; it is often scattered across departments and systems. Here is where data integration tools come into play. These tools help organizations consolidate their data, making it easier to analyze and derive insights. The importance of understanding these integration tools lies in their ability to enhance operational efficiency and support informed decision-making.
Data integration tools are essentially the unsung heroes of data management. They allow companies to combine data from different sources, ensuring accuracy and consistency. This process not only minimizes errors but can also speed up the analysis process. Moreover, in an age where data is considered the new oil, having a coherent data strategy supported by the right integration tools can be the difference between success and falling behind competitors.
ETL (Extract, Transform, Load) Tools
ETL tools are like the workhorse of data integration. They extract data from various sources, transform it into a suitable format, and load it into a target system, usually a data warehouse. The transformation stage is particularly vital, as it involves cleaning and organizing the data. Here, one can set rules or use algorithms to standardize data formats.
Key Features of ETL Tools:
- Data Quality Checks: Ensure the accuracy and integrity of the data.
- Scheduled Jobs: Automate regular data processing tasks.
- Support for Multiple Data Sources: Connect to various databases and APIs.
ETL tools are particularly beneficial when handling data from legacy systems or structured raw data. Companies that need to generate reports or perform complex queries often rely on ETL for efficient data processing.
ELT (Extract, Load, Transform) Tools
ELT, on the other hand, flips the ETL process on its head. Instead of transforming data before loading it to the data warehouse, it loads raw data first and then processes it in the target system. This approach is becoming increasingly popular, especially with the advent of cloud technologies where storage is cheaper and processing power is scalable.
Benefits of ELT Tools:
- Faster Loading Times: Data is available for analysis sooner.
- Flexibility in Analysis: Users can perform ad-hoc transformations, allowing for more diverse analyses.
- Efficient Use of Cloud Resources: Takes advantage of cloud computing power for heavy transformations.
ELT tools are ideal for organizations that use modern data lakes or big data environments. They cater well to ambitious data initiatives where flexibility and access to raw data are paramount.
Data Virtualization Solutions
Data Virtualization Solutions provide a unique approach by retaining the physical data in its original location and displaying it as if it were housed in one single repository. Unlike ETL and ELT, there’s no need to move data around. This can dramatically reduce data latency and storage costs while enhancing accessibility.
Advantages of Data Virtualization:
- Real-time Access: Users can access up-to-date data without delays associated with data movement.
- Cost-effectiveness: Reduces the need for extensive data storage solutions.
- Simplified Data Access: Users can query data across different sources without needing technical know-how.
Data virtualization is especially useful for organizations dealing with heterogeneous data environments where maintaining multiple data sources is necessary, but hassle-free access is key.
"The right data integration strategy can be the backbone of effective decision-making; without it, businesses can find themselves navigating blind."
Overall, the choice of data integration tools varies significantly based on the organizational requirements, data types, and existing infrastructure. Understanding these different tools can provide invaluable insights and guide businesses towards making informed, strategic decisions.
Data Integration Tools List
When discussing data integration tools, it’s pivotal to understand the significance of having a well-structured toolkit geared towards facilitating seamless data flows. The list of data integration tools not only serves as a reference but also highlights the diversity of options available to businesses, each tailored to specific needs and operational contexts. In the competitive realm of data management, choosing the right tool can enhance operational efficiencies, mitigate risks, and promote data-driven decision-making.
Selecting the right data integration tool involves careful consideration of unique features, capabilities, and constraints that align with organizational demands. The ensuing sections will scrutinize various categories of data integration tools, providing insights on their strengths and weaknesses, which empowers decision-makers to make informed choices.
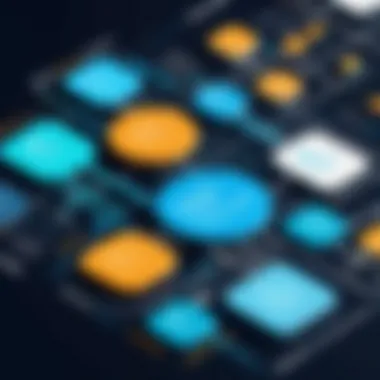
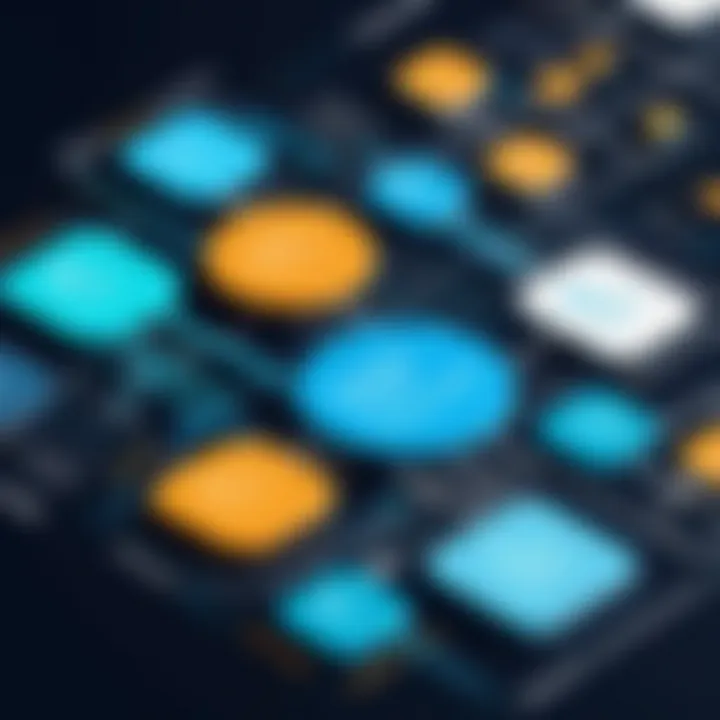
Leading ETL Tools
Tool A: Features and Benefits
Tool A stands out because of its flexible architecture that allows for a wide range of data sources. The key characteristic of this tool is its capacity to handle massive volumes of data while ensuring minimal downtime. This flexibility makes it a preferred choice for organizations looking to consolidate data efficiently. One unique feature that Tool A offers is real-time processing, which is vital for businesses that require immediate data availability.
The advantages are clear: enhanced data quality and a structured workflow allow for more rapid decisions based on accurate data. However, some users mention that its initial learning curve can be steep, particularly for those unfamiliar with similar integration solutions.
Tool B: Use Cases
When discussing Tool B, its application across various sectors like finance and healthcare cannot be overlooked. The prominent use case for Tool B lies in its ability to integrate disparate systems swiftly and efficiently. Its ability to smooth over complexities during large-scale system upgrades makes it appealing in high-stakes industries.
A unique attribute of Tool B is its robust data cleansing feature, enabling organizations to maintain accuracy visually across all integrated datasets. The drawbacks include costs that can escalate significantly based on the number of connections required, limiting some smaller businesses from fully leveraging its capabilities.
Tool C: Industry Applications
Tool C is heralded in certain industry circles for its capacity to adapt to evolving data landscapes, particularly in retail and e-commerce. It efficiently combines transactional data with customer insights, delving into patterns that help enhance customer experiences. This notable characteristic of Tool C is its ability to integrate marketing platforms to improve outreach.
Moreover, Tool C has a unique predictive analytics component, which aids in foreseeing market trends. However, its reliance on extensive configuration may deter organizations looking for an out-of-the-box solution.
Prominent ELT Tools
Tool D: Features and Benefits
Diving into Tool D, it's significant for its cloud-native architecture which sets it apart in the ELT landscape. The tool's inherent strength lies in its capacity to handle both structured and unstructured datasets without significant adjustments to its core. One key feature to note is its auto-scaling capabilities, which ensure performance during peak usage periods.
While widely recognized for its cost-effectiveness, it does present challenges such as the potential for increased latency during large data loads, which can be a concern for real-time analytics.
Tool E: Use Cases
Turning to Tool E, we see its versatile application in the logistics and supply chain sectors, particularly for its streamlined integration with IoT devices. This capability allows for precise tracking and management of inventory, something that organizations are increasingly looking to optimize.
Its ease of use and efficient setup process have made it a popular choice among small to mid-sized businesses, but the unique challenge of Tool E is that it may require additional components for more complex integrations, which can add to total costs.
Tool F: Industry Applications
Focusing on Tool F, its capability in processing data from various cloud services demonstrates its utility in tech startups and digital enterprises. The key characteristic of Tool F lies in its advanced security protocols, which is critical as businesses navigate a landscape increasingly fraught with data privacy concerns.
This tool also features integrations with machine learning models, providing organizations with the flexibility to harness data insights dynamically. Nonetheless, some have noted that its functionality can feel overwhelming due to richness of features, making initial usage a bit of a challenge.
Notable Data Virtualization Tools
Tool G: Features and Benefits
Tool G thrives in environments that prioritize data accessibility. Its distinct advantage is its capability to provide a unified view of data across multiple sources without the necessity of duplication. This is especially beneficial for organizations aiming for high data integrity yet utilizing a mix of on-premises and cloud services.
The elegant design of Tool G allows teams to easily construct queries using familiar languages, keeping the learning curve relatively low. Still, its dependency on network speed may affect performance under high traffic conditions.
Tool H: Use Cases
In the case of Tool H, its application in business intelligence enables organizations to generate reports from across various platforms swiftly. This core function supports decision-makers who need to act quickly based on the latest available data.
Flexibility is a hallmark of Tool H, as it integrates well with other analytics solutions, allowing for enhanced functionality. However, organizations may face challenges in achieving optimal performance if their source systems are not robust or properly configured.
Tool I: Industry Applications
Lastly, Tool I has made its mark in sectors like telecommunications, where data management complexity requires streamlined solutions. The key benefit of Tool I is its capacity to correlate vast streams of data in real time, granting insights that propel operational strategies.
A unique highlight is Tool I's collaboration functionalities, allowing cross-departmental teams to work together on data projects seamlessly. However, the initial setup can be intensive, requiring dedicated resources to optimize effectively.
Cost Considerations for Data Integration Tools
When selecting a data integration tool, one mustn’t overlook the financial aspects that accompany these technologies. Cost considerations play a pivotal role in determining the long-term viability and effectiveness of the integration solution, as they not only affect initial budgeting but also dictate strategic planning and resource allocation for future needs.
Making a well-informed choice in this arena can lead to significant operational efficiency, often translating into improved performance metrics and better return on investment. Here are two main aspects to think about under the umbrella of cost considerations:
Licensing Models
A significant element in the cost arena is how the licensing for the data integration tool is structured. Different models exist:
- Perpetual License: This model usually requires a one-time payment, allowing you to use the software indefinitely. While it might seem cost-effective upfront, maintenance costs or additional fees for upgrades can add up over time.
- Subscription-Based: In contrast, a subscription model offers a lower initial investment, usually charged monthly or annually. This can be appealing for startups or companies looking to avoid hefty upfront costs. However, over an extended period, the total costs can exceed that of a perpetual license.
- Usage-Based Licensing: This method allows billing based on actual usage or data volume processed. Although it offers flexibility, predicting costs can be tricky.
Selecting the right licensing model requires careful consideration of your organization's operational scale and future growth plans.
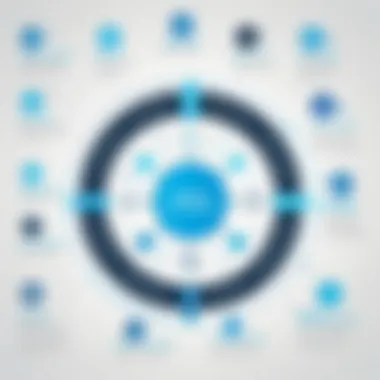
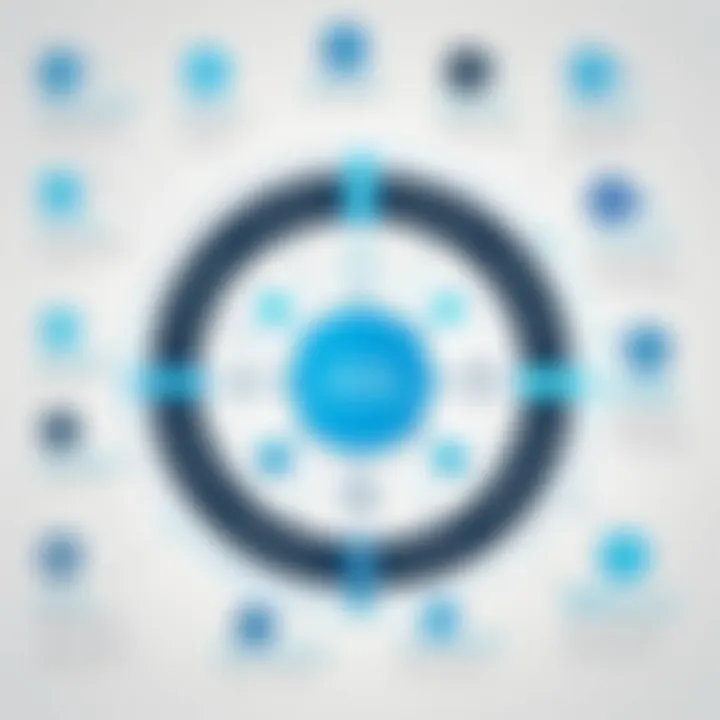
Total Cost of Ownership
Understanding the total cost of ownership (TCO) is another critical step in the decision-making process. TCO encompasses all costs associated with the tool over its lifetime, including:
- Acquisition Costs: Initial purchase or subscription fees.
- Operational Costs: Ongoing expenses related to maintenance, training, and support staff required to manage the tool.
- Upgrade Expenses: Costs incurred for enhancements or newer versions of the software.
- Opportunity Costs: Any potential revenue loss or inefficiency resulting from poor integration, or missed features that may necessitate switching tools later.
"A wise investment requires more than a glance at the price tag; it demands a thorough assessment of its long-term impacts."
It's crucial to look beyond sticker prices and understand how the choice of a data integration tool will affect not just the immediate budget but also overall operational capacity over time. Aligning both licensing models and total cost of ownership with the organization's long-term strategy ensures a smoother journey through the complexities of data management, ultimately paving the way for enhanced productivity and streamlined operations.
Best Practices for Implementing Data Integration
Implementing data integration is no walk in the park. It requires a clear strategy to ensure that all systems work harmoniously together. Getting it right can lead to enhanced efficiency and productivity, but you have to navigate tougher waters to reap the rewards. This section will discuss best practices that can streamline the integration process and avoid common pitfalls.
Establishing Clear Objectives
Setting clear objectives is the first step towards successful data integration. Without a well-defined aim, you may find yourself wandering aimlessly like a ship lost at sea. Objectives serve as a compass, keeping you on course. Here are some key considerations when setting your objectives:
- Identify Key Stakeholders: Engaging various teams can give you a well-rounded view of what is needed. Different departments have different requirements, and aligning these can create a unified system that meets everyone’s needs.
- Assess Existing Infrastructure: Before you dive into integration, take stock of current tools and technologies. Knowing what’s already in place helps to avoid redundancy.
- Define Success Metrics: How will you measure success? Whether it’s improved data quality, faster access to data, or something else, clear metrics will guide your implementation.
Continuous Monitoring and Optimization
Integration doesn’t just end after implementation—it’s an ongoing journey that requires diligent monitoring and adjustment. Think of it like a garden; if you don’t tend to it, the weeds will take over. Here are some strategies to ensure everything runs smoothly:
- Establish Regular Check-Ins: Schedule periodic evaluations of your system's performance. Are your objectives being met? If not, it's time to reassess.
- Use Analytics Tools: Employing analytics tools can provide insights into performance bottlenecks. Data visualization often helps in spotting patterns that may not be visible at first glance.
- Adapt and Iterate: Technology evolves rapidly. Be prepared to adapt your integration strategies to align with new trends or technologies. Staying proactive rather than reactive can save time and effort in the long run.
"In the world of data, stagnation is a recipe for disaster. Continuous improvement is the way to keep ahead of the curve."
In short, a successful data integration strategy is a moving target, requiring you to be flexible and alert. By establishing clear objectives and continuously monitoring your systems, you can navigate the rough waters of data management while ensuring that your organization remains competitive.
Future Trends in Data Integration
The realm of data integration is not stagnant; it evolves rapidly to keep pace with technological advancements. Understanding future trends in this area is not just beneficial but essential for organizations striving to stay ahead in a data-driven world. Companies can leverage these trends to drive innovation, enhance operational efficiency, and optimize their data management processes.
AI and Machine Learning in Data Integration
Artificial Intelligence (AI) and Machine Learning (ML) are at the forefront of many discussions surrounding data integration. These technologies are no longer just buzzwords; they are transforming how organizations handle data.
By implementing AI-driven data integration solutions, organizations can automate many of the labor-intensive tasks associated with data management. For instance, AI can help in data cleansing by identifying and correcting errors automatically. This enhances data quality and reduces the time and resources that organizations must allocate to data preparation.
Furthermore, Machine Learning algorithms can analyze patterns and predict future data changes, allowing businesses to adapt their integration strategies proactively. For instance, when handling customer data, a ML model could identify emerging trends in consumer behavior, enabling companies to tailor their marketing efforts accordingly.
- Benefits of AI and ML in Data Integration:
- Automated data quality control
- Enhanced predictive analytics
- Streamlined operational workflows
Cloud-based platforms, often incorporating AI capabilities, are becoming the norm. They allow for quicker deployment and scalability, providing organizations with the agility they need in response to market changes.
Cloud-Based Data Integration Solutions
Cloud computing has revolutionized many industries, and data integration is no exception. Organizations are increasingly opting for cloud-based data integration solutions due to several compelling reasons.
By utilizing cloud platforms, businesses no longer have to invest heavily in on-premise infrastructure, which can be both cost-prohibitive and cumbersome. Instead, they can choose subscription models that fit their current needs and scale as required. This flexibility can lead to significant cost savings and allows for better allocation of resources.
Moreover, cloud-based solutions often provide enhanced collaboration features. Teams spread across different geographical locations can access the same data in real-time, making decision-making faster and more cohesive. Additionally, these solutions generally come with robust security features, crucial in today’s data-sensitive environment.
"In the modern landscape, leveraging cloud-based services for data integration can provide a competitive edge that is difficult to achieve otherwise."
- Key Considerations for Cloud-Based Solutions:
- Evaluate the security measures in place
- Analyze the pricing models available
- Consider integration capabilities with existing systems
End
In the realm of data integration, arriving at meaningful conclusions is essential for businesses looking to maximize their data assets. As explored throughout this guide, selecting the right data integration tools isn't merely about plugging in software; it’s about aligning these solutions with your organizational goals, technical environment, and user needs.
The benefit of a well-chosen data integration tool can’t be understated. It can streamline operations, improve data quality, and provide a robust framework for analytics. With the ever-growing volume of data in various formats, integrating these disparate data sources into a coherent structure is no longer a luxury but a necessity. In short, it'd be akin to trying to make a puzzle without all the pieces—it just doesn't add up.
Here are several considerations that should linger in the minds of decision-makers as they contemplate their data integration strategies:
- Return on Investment: Think about the Total Cost of Ownership, including potential savings from operational efficiencies.
- Scalability: As needs grow, can the tool accommodate more data sources without breaking a sweat?
- Technology Landscape: How well can these tools mesh with the existing tech stack? Interoperability can save headaches down the line.
Adopting newer trends, like AI and machine learning in data integration practices, can yield insights that traditional tools might overlook. Companies that stay ahead of these trends are better situated to adapt and thrive in a data-driven world.
To encapsulate, data integration is not just a tech exercise—it’s a strategic move. Investing time in understanding the available tools will ensure that organizations are not only reacting to current data management needs but also preparing for future challenges that lurk just beyond the horizon. Making informed choices today can lead to innovative solutions tomorrow, ultimately enhancing operational efficiencies and equipping organizations to harness the full potential of their data.
"The richness of data integration lies not just in the tools themselves, but in how they empower organizations to unlock their hidden insights."
By engaging with this comprehensive guide, readers can better navigate the complex landscape of data integration tools and make choices that are deliberate and strategic. The goal should always be to cultivate a robust data integration environment that evolves with the organization, enabling better decision-making and driving success.