Big Data and Health Informatics: Transforming Healthcare
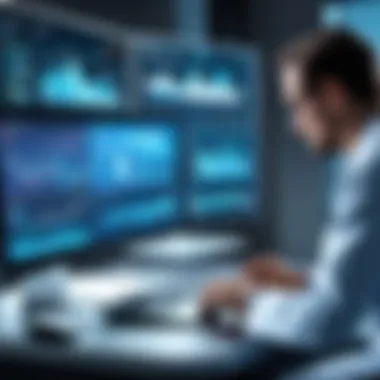
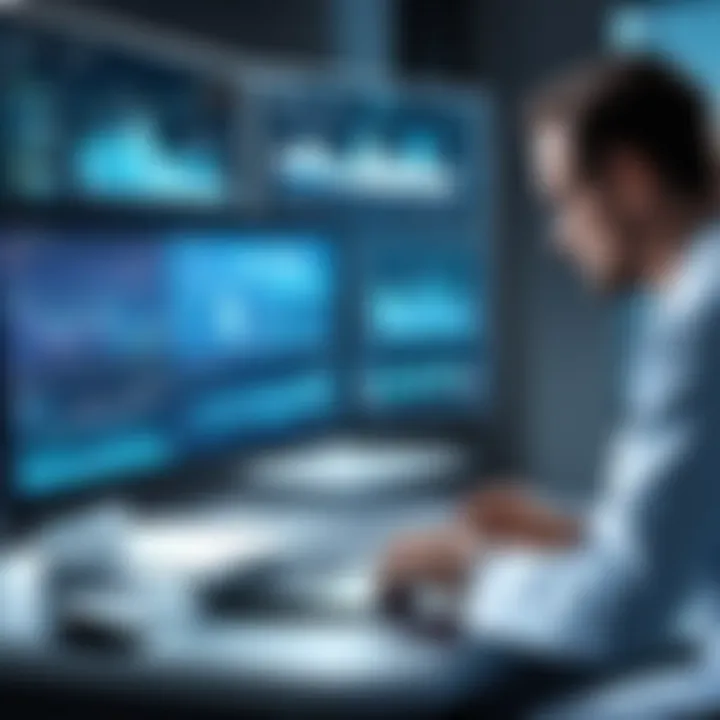
Intro
The field of health informatics is undergoing significant transformation due to the burgeoning presence of big data. As healthcare systems across the globe increasingly rely on data to inform clinical decision-making, integrate patient care, and streamline operations, the nexus of big data and health informatics emerges as a critical area of exploration. This article will investigate the essential aspects of this intersection, providing a nuanced understanding of its relevance, applications, and implications for the future of healthcare.
Key Software Features
In the realm of health informatics, software is pivotal for managing and analyzing large volumes of data. The functionality varies across platforms, but several features stand out as essential for effective integration.
Essential functionalities
- Data Storage and Management: Systems must effectively handle vast amounts of patient data, ensuring scalability and accessibility.
- Interoperability: Health informatics software needs to support communication between diverse health information systems, promoting seamless data exchange.
- Analytics Capabilities: Tools should provide robust analytical features to derive actionable insights from data, including predictive analytics and visual reporting.
- User-Friendly Interface: Given the diverse range of users, an intuitive interface remains crucial for adoption and ensuring data can be used with minimal training.
Advanced Capabilities
- Artificial Intelligence: Incorporating AI can significantly enhance data analysis, revealing patterns and predictive trends that may not be readily apparent.
- Real-Time Data Processing: The ability to analyze data in real-time allows for Instant decision-making and improved patient outcomes.
- Patient Engagement Tools: Solutions offering direct access to health records and predictive health alerts contribute to proactive patient management.
- Compliance and Security Features: Ensuring patient data privacy and adhering to regulations such as HIPAA are essential components of any software deployment in health informatics.
"The integration of big data in healthcare not only improves efficiency but also enhances the quality of care."
Comparison Metrics
Evaluating different health informatics software often requires careful consideration of various metrics.
Cost analysis
Understanding the cost implications involves more than just the upfront expenditure. Factors such as maintenance, training, and software updates should also be accounted for.
Performance benchmarks
Performance metrics, including processing speed, accuracy of analytics, and user satisfaction ratings, provide essential insights into the efficacy of software solutions. Regular benchmarking against industry standards is crucial for decision-makers aiming for best practices in health informatics.
Prologue to Big Data Health Informatics
The realm of healthcare is rapidly evolving, driven by technological advancements and an increasing volume of data. Understanding Big Data in health informatics is crucial because it serves as a bridge between large datasets and actionable insights. This intersection not only impacts patient outcomes but also enhances the efficiency of healthcare systems. With the integration of vast amounts of data, health informatics emerges as a foundational element that supports decision-making processes in clinical and operational settings.
Big Data typically refers to data sets that are so large or complex that traditional data processing software cannot manage them effectively. The relevance of Big Data in healthcare lies in its potential to facilitate improved patient care and operational efficiencies. Harnessing various data sources can yield significant insights into disease patterns, treatment effectiveness, and overall health trends. Therefore, understanding how to leverage this data is essential for industry professionals.
Defining Big Data in Healthcare
Big Data in healthcare encompasses a wide range of information collected from clinical settings, wearable devices, electronic health records (EHRs), and even social media. This data can be structured, semi-structured, or unstructured. Common examples include:
- Clinical Data: Patient records, treatment histories, and clinical trial information.
- Genomic Data: Genetic profiles and sequences that play a role in personalized medicine.
- Operational Data: Metrics related to hospital efficiency, such as patient wait times and resource allocation.
The defining characteristic of Big Data is the Three Vs: volume, velocity, and variety. Volume refers to the immense amount of data generated daily. Velocity pertains to the speed at which data is generated and processed. Lastly, variety indicates the different forms of data, from numerical to text and images.
Importance of Health Informatics
Health informatics stands as a critical domain that merges health care, information science, and computer technology. The importance of this field cannot be overstated. It enhances the capability of healthcare providers to deliver effective patient care by transforming raw data into useful information. Key aspects include:
- Patient Safety: By analyzing data trends, healthcare practitioners can identify potential risks to patient safety and make informed decisions to mitigate them.
- Research and Innovation: Health informatics supports research initiatives, enabling data-driven studies that can lead to new treatment protocols and healthcare innovations.
- Regulatory Compliance: With stricter regulations in healthcare, informatics helps organizations manage and maintain compliance with data protection laws.
"The successful integration of health informatics into routine care practices holds the potential to revolutionize patient engagement and satisfaction."
Through digital health records and real-time analytics, decision-makers in healthcare can make more informed choices. The field enables improved communication among healthcare teams and fosters collaboration across disciplines, ultimately benefiting patients.
In summary, understanding the basics of Big Data health informatics sets the stage for a deeper exploration of its applications, benefits, and challenges. The health sector's ability to adapt and evolve with these technologies will be imperative in addressing future healthcare demands.
The Role of Big Data in Health Informatics
Big Data plays a transformative role in health informatics. By harnessing vast amounts of data, healthcare systems can gain insights that significantly improve patient care, streamline operations, and foster innovation. This section will explore how the integration of massive data sets contributes to advancements in healthcare, highlighting the specific benefits and considerations.
Enhancing Patient Care
Patient care has been revolutionized by the insights derived from big data analytics. The ability to analyze electronic health records, genomic data, and real-time patient monitoring enables healthcare providers to understand patient needs comprehensively. This leads to more accurate diagnoses and tailored treatment plans. For instance, predictive analytics can identify patients at risk of certain conditions, allowing for early intervention.
Furthermore, the real-time data collected from wearable devices can be analyzed to provide patients constant feedback on their health status. This encourages patients to take a more active role in their healthcare decisions, improving adherence to treatments and lifestyle changes.
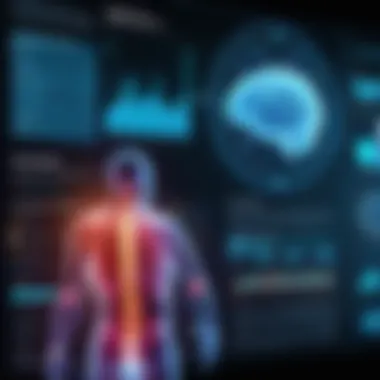
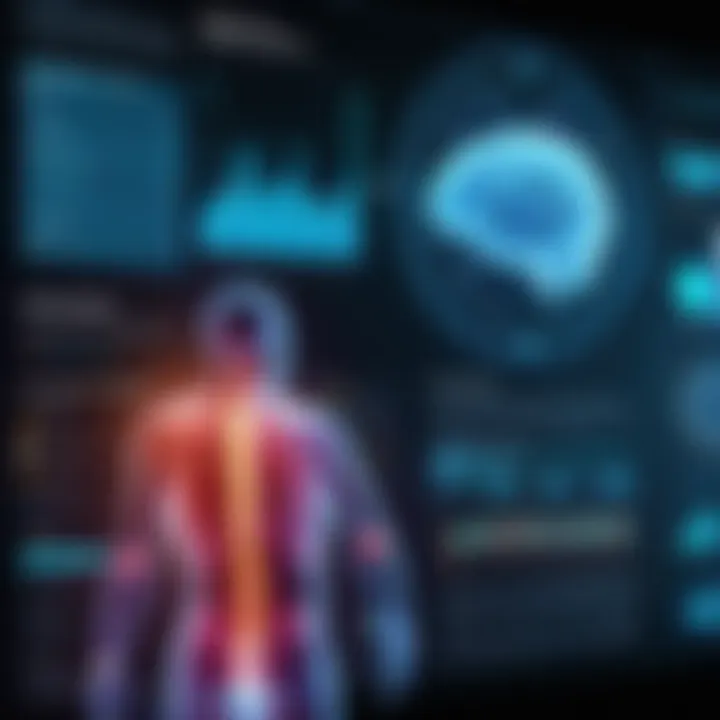
Access to a patient’s complete health history can also improve communication among care teams. When all members can view the same information, it can reduce chances of medical errors and enhance collaborative care.
Facilitating Research and Innovation
Big data serves as a foundation for groundbreaking research in medicine and public health. Researchers can access, analyze, and interpret extensive datasets to discover correlations and causal relationships that were previously undiscovered. For example, analyzing large-scale clinical trials or population health data allows researchers to identify trends in treatment efficacy across diverse populations.
Innovative solutions emerge from this data. By leveraging machine learning algorithms, researchers can predict the onset of diseases and suggest preventive measures. Moreover, insights from big data not only improve current treatment protocols but also inform future pharmaceutical developments, potentially speeding up the drug discovery process.
By sharing anonymized data among institutions, researchers can work collaboratively across the globe, fostering an environment of transparency and innovation. This collaboration can lead to the rapid advancement of knowledge and the development of novel healthcare solutions.
Improving Operational Efficiency
Operational efficiency is critical for healthcare organizations facing financial pressures. Big data analytics can streamline logistics in health informatics by optimizing resource allocation and reducing waste. For example, predictive analytics can forecast patient admissions, enabling better staff scheduling and resource management.
Moreover, big data tools assist in identifying bottlenecks in care delivery. Analyzing patterns in patient flow can highlight areas where processes slow down, allowing healthcare administrators to enhance workflows and ensure timely care.
Another aspect is the utilization of data to monitor expenditures and budget effectively. Real-time analysis of financial data can reveal spending patterns, making it easier to identify areas for cost reduction without compromising quality of care. In essence, the effective integration of big data can lead to a more profitable and patient-centric healthcare system.
"The integration of big data analytics is not just a trend—it is becoming essential for modern healthcare practices aiming to improve outcomes and manage costs effectively."
Data Sources in Health Informatics
In the landscape of health informatics, the significance of data sources cannot be underestimated. The integration of diverse data sources provides healthcare professionals with a holistic view of patient health and operational efficiency. Understanding the types of data available is crucial for making informed decisions that can enhance patient care and optimize health systems. With the convergence of big data and health informatics, leveraging these data sources enables new insights into patterns and trends that affect health outcomes.
Clinical Data
Clinical data forms the cornerstone of health informatics. This includes information collected during patient interactions with healthcare providers, such as electronic health records (EHRs), diagnostic images, and lab test results. The use of EHRs has revolutionized the way clinical data is collected and managed, leading to improved patient documentation and better coordination of care.
Key benefits of clinical data include:
- Enhanced patient history tracking
- Improved decision-making through access to comprehensive data
- Facilitation of evidence-based practice and research
However, challenges exist in ensuring the quality and accuracy of clinical data. Inconsistencies in data entry practices and varying standards across institutions can lead to issues in data reliability.
Wearable Devices and IoT
Wearable devices and the Internet of Things (IoT) represent a growing source of health data that contributes to personalized patient care. Devices such as smartwatches and fitness trackers collect real-time health metrics, including heart rate, activity levels, and sleep patterns. The integration of these technologies into health informatics presents several advantages:
- Continuous monitoring of patient health metrics
- Timely interventions based on real-time data
- Increased patient engagement in their own health management
However, the vast amount of data generated by these devices raises concerns regarding data privacy and security. Protecting sensitive information shared through these platforms is critical.
Patient Generated Health Data
Patient-generated health data (PGHD) encompasses any health data that is recorded or created by patients themselves, typically outside of clinical settings. This may include information gathered through surveys, mobile health applications, or social media interactions about health experiences. Utilizing PGHD in health informatics offers numerous benefits:
- Greater patient involvement in health decisions
- Enabling healthcare providers to better understand patient circumstances
- Identifying trends and outcomes directly from the patient perspective
Despite its potential, incorporating PGHD into formal healthcare systems poses challenges. The validity and accuracy of self-reported data can be questionable, and there is a need for standardized methods of data collection.
"Data sources in health informatics are not just about gathering information; they represent the building blocks for creating a responsive healthcare ecosystem."
Technologies Driving Big Data in Health Informatics
The significance of technologies in harnessing big data for health informatics cannot be overstated. These technologies enable health organizations to collect, analyze, and utilize vast amounts of data to improve patient care, streamline processes, and foster innovation. To fully leverage big data's potential, it is imperative to understand the various technologies driving this transformation.
Data Analytics Tools
Data analytics tools serve as the backbone of big data initiatives in healthcare. They allow organizations to sift through complex data sets and extract meaningful insights. By employing tools such as Apache Hadoop, Tableau, and SAS, healthcare providers can analyze patient records, treatment outcomes, and operational efficiencies. These analytics not only enhance clinical decision-making but also support predictive modeling and population health management.
"Data analytics in healthcare can lead to more informed decisions and improved patient outcomes."
The integration of real-time analytics capabilities enables healthcare professionals to monitor patient data continuously. This results in timely interventions and improved quality of care. Additionally, the incorporation of visual analytics tools aids in data presentation, making it easier for stakeholders to interpret the results and drive strategic initiatives.
Artificial Intelligence and Machine Learning
Artificial Intelligence (AI) and Machine Learning (ML) represent revolutionary fronts in the domain of health informatics. These technologies analyze patterns in large datasets, enabling predictive analytics. Machine learning algorithms can identify risk factors for diseases and suggest personalized treatment plans based on historical data.
Health systems are also adopting AI-powered diagnostic tools that assist in detection of conditions such as radiological abnormalities, which can enhance accuracy and reduce diagnostic errors. According to recent studies, the integration of AI in diagnostic processes has shown significant improvement in health outcomes.
The use of AI and machine learning not only streamlines operations but also optimizes resource allocation. Hospitals can anticipate patient inflow and manage staff workloads effectively, ensuring better operational efficiencies and ultimately, patient satisfaction.
Cloud Computing Solutions
Cloud computing has emerged as a game-changer for health informatics. It enables scalable data storage and retrieval, making it easier for organizations to handle large volumes of data without heavy investment in infrastructure. Solutions like Amazon Web Services and Microsoft Azure provide healthcare entities with the flexibility to access data from anywhere, promoting collaborative research and data sharing among institutions.
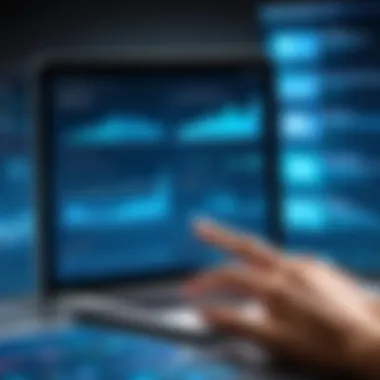
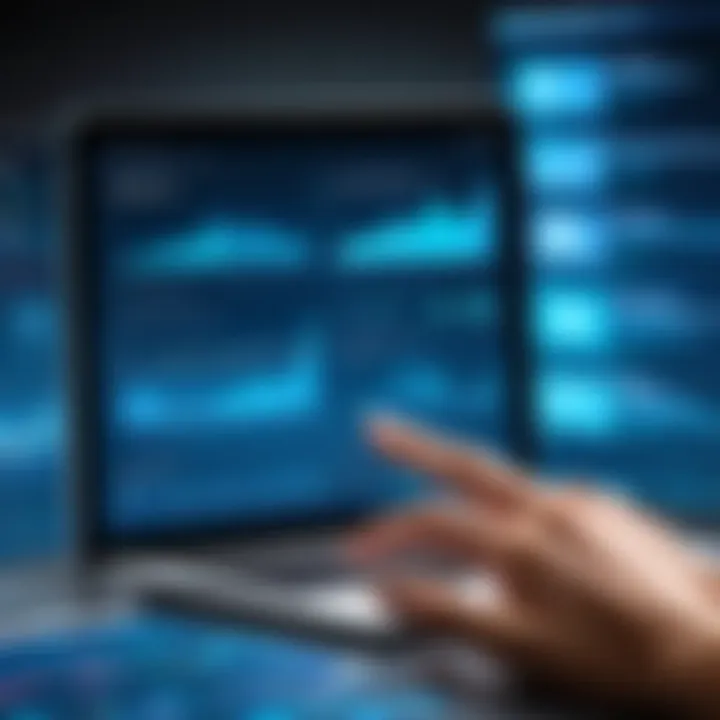
Cloud platforms also offer enhanced security features to protect sensitive health data. By utilizing cloud solutions, healthcare organizations can ensure compliance with regulations such as HIPAA while maintaining high service availability.
Furthermore, the scalability of cloud computing supports the integration of advanced analytics and AI tools, which enhances the capability to analyze data efficiently. This integration allows organizations to adapt quickly to changing healthcare needs and innovations.
Challenges in Big Data Health Informatics
The integration of big data into health informatics promises substantial improvements across various healthcare dimensions. However, these advancements are accompanied by notable challenges. Understanding these challenges is essential for stakeholders, as they influence the effectiveness and reach of big data applications in healthcare.
As big data systems expand, it raises concerns about data privacy and security. Organizations must address these issues to maintain patient trust and comply with regulations. Furthermore, data integration and interoperability present significant technical barriers that can obstruct the seamless flow of data between systems. Lastly, ensuring the quality and reliability of data is vital. Without accurate data, insights and decisions derived from analytics become questionable.
Navigating these challenges requires concerted efforts from all sectors involved. By identifying and addressing these obstacles, healthcare professionals can harness the full potential of big data in innovative ways.
Data Privacy and Security Issues
Big data encompasses vast amounts of sensitive patient information. Therefore, data privacy and security are paramount. Organizations need to deploy robust security protocols to protect against breaches and unauthorized access. Failure to do so can lead to severe consequences, including financial penalties and damage to reputation.
Regulatory frameworks such as the Health Insurance Portability and Accountability Act (HIPAA) in the United States play a critical role in guiding organizations in safeguarding data. Compliance with these regulations is non-negotiable. Stakeholders must adopt encryption, secure data storage, and continuous monitoring of data access to strengthen their defense against potential threats. Furthermore, there need to be consistent training and awareness programs to educate staff about potential vulnerabilities.
Data Integration and Interoperability
Data integration and interoperability are critical for a cohesive health informatics framework. Various systems generate data, from clinical records to laboratory results. These systems must communicate effectively to establish a holistic view of a patient’s health status. Unfortunately, many organizations use disparate systems that cannot easily share information, leading to silos.
Investing in solutions that facilitate interoperability is a necessity. Implementing standardized data formats and protocols can help alleviate compatibility issues. Additionally, engaging with industry stakeholders to develop shared frameworks can lead to improved data integration efforts.
Quality and Reliability of Data
The effectiveness of big data analytics hinges on the quality and reliability of the data it processes. Inaccurate or incomplete data can lead to misleading results, which may negatively impact patient care and operational decisions. Organizations need to ensure that the data collected is precise and up-to-date.
Implementing data validation techniques and routine audits can enhance data integrity. It is also essential to adopt comprehensive data governance strategies. This includes establishing clear data ownership, accountability standards, and processes for regular data review.
"Investing in data governance is as critical as investing in technology itself. Without accountable data management, no amount of big data will bring desired outcomes."
In summary, addressing the challenges associated with big data health informatics is a multifaceted endeavor. Stakeholders must prioritize data privacy, integration, interoperability, and quality to foster an environment where big data can truly excel in transforming healthcare practices.
The Future of Big Data in Health Informatics
The future of big data in health informatics is poised to shape the healthcare sector in numerous significant ways. As data continues to proliferate across various healthcare platforms, the utilization of this data will become increasingly critical. Enhanced analytics capabilities and sophisticated algorithms will enable stakeholders to derive actionable insights. It is vital for decision-makers and IT professionals to understand the implications of these advancements as they navigate the evolving landscape of healthcare technology.
The integration of big data can result in improved patient outcomes through personalized treatments and enhanced decision-making processes. As healthcare providers increasingly rely on data-driven solutions, dynamic diagnostic tools will emerge, allowing for more accurate predictions of health events. These innovations engender a more responsive and proactive healthcare system.
Emerging Trends and Technologies
Several trends are currently reshaping the landscape of big data in health informatics. The rise of artificial intelligence and machine learning, for instance, has provided healthcare organizations with robust tools for analyzing vast datasets. Algorithms capable of processing and interpreting complex data patterns will enable more informed clinical decisions and predictive healthcare.
- Wearable Technology: Wearable devices are revolutionizing how health data is collected, as they allow real-time monitoring of patient vitals. This enhances how providers assess patient conditions, leading to timely interventions.
- Telemedicine: The growth of telehealth services has highlighted the importance of data analytics in remote care delivery. It allows providers to monitor patient data remotely and provide care without geographical limitations.
- Blockchain Technology: The incorporation of blockchain can enhance data security and privacy, which are paramount in health informatics. It allows for secure sharing of patient data among various stakeholders, fostering trust in data integrity.
Potential Use Cases
The potential use cases for big data in health informatics are vast. These applications can lead to innovative solutions that reshape patient care.
- Predictive Analytics: By harnessing historical health data, predictive analytics can anticipate disease outbreaks or patient readmission risks. This allows for tailored prevention strategies.
- Personalized Medicine: Big data enables the customization of treatment plans based on individual genetic profiles and lifestyles. This can significantly enhance therapeutic effectiveness.
- Operational Efficiency: Analyzing workflow data can reveal inefficiencies within healthcare systems. Streamlining these processes can result in cost savings and improved service delivery.
Regulatory Considerations
As big data continues to evolve, regulatory frameworks must adapt to ensure patient safety and data privacy. Key considerations include:
- Data Protection Regulations: Compliance with regulations such as HIPAA in the United States and GDPR in the European Union must be at the forefront of data management practices. These laws set strict guidelines for how patient information is handled and shared.
- Ethical Standards: Establishing ethical standards around the use of AI in healthcare is crucial. This ensures transparency and accountability when algorithmic decisions impact patient care.
- Interoperability Standards: Promoting the creation of universal guidelines for data sharing will enhance interoperability among systems, facilitating better collaboration between health informatics stakeholders.
Ensuring that these considerations are addressed will help to cultivate an environment where big data can thrive, ultimately benefiting all participants in the healthcare ecosystem.
"The potential for big data to transform healthcare is immense, provided that privacy and ethical standards are established clearly."
Through a comprehensive approach to embracing big data, healthcare professionals can pave the way for innovations that not only enhance clinical outcomes but also ensure a more efficient healthcare delivery model.
The Impact of Big Data on Stakeholders
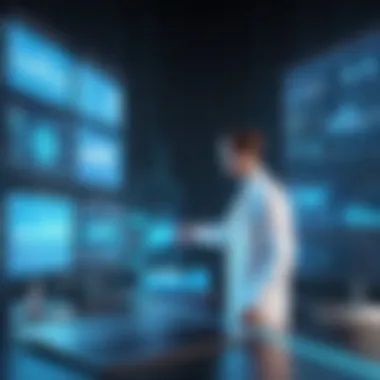
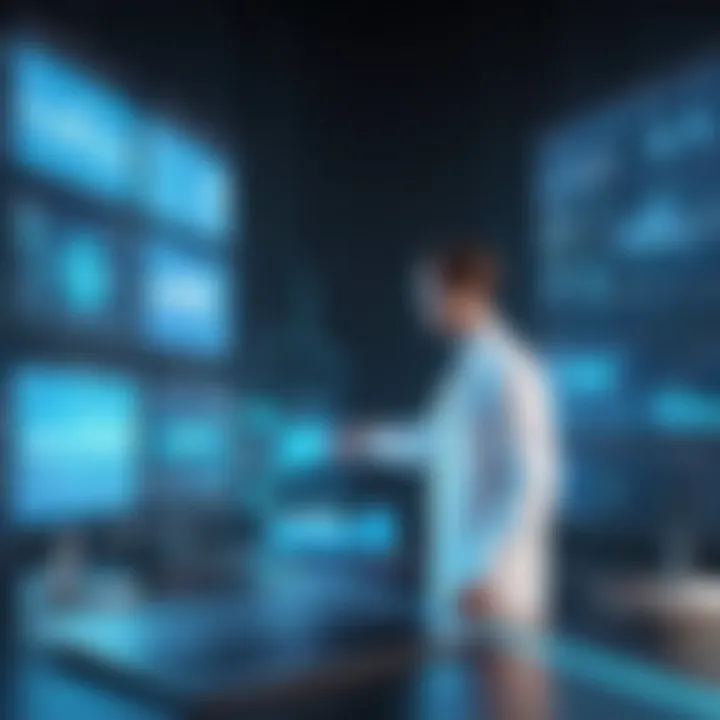
Understanding the effects of big data on various stakeholders in healthcare is essential for grasping the full potential of health informatics. Each group—healthcare providers, patients, and policy makers—experiences unique challenges and advantages. By focusing on these elements, we can see how big data not only optimizes practices but also reshapes patient and policy dynamics.
Healthcare Providers
Big data significantly enhances the capabilities of healthcare providers. Advanced analytics and data collection methods allow for improved patient diagnostics and customized treatment strategies. For instance, predictive analytics can identify patient risks before they manifest, enabling preemptive interventions that improve patient outcomes.
Moreover, data from electronic health records can lead to streamlined workflows and reduced administrative burdens. This efficiency gain has dual benefits: providers can focus more on patients and less on paperwork. With real-time access to consolidated patient information, healthcare providers can make informed decisions promptly. This leads to better care coordination and, ultimately, higher patient satisfaction.
In addition to operational enhancements, big data fosters a culture of learning among providers. By analyzing treatment outcomes across patient populations, providers can identify successful protocols and adopt evidence-based practices. Continuous feedback loops from data analytics mean that healthcare becomes more adaptive and patient-centered.
Patients
Patients are also major beneficiaries of big data. Access to personalized health information promotes a greater involvement in their own care. Healthcare analytics facilitate tailored health plans that address individual patient needs, preferences, and behaviors. This improves not just outcomes but encourages more engaged patient participation.
Furthermore, the use of wearables and mobile health applications allows patients to monitor their health metrics actively. These data can be shared with healthcare providers, leading to well-informed healthcare decisions. Patients become partners in their care rather than passive recipients. This transformation in the patient-provider dynamic results in a more collaborative approach to health management.
However, there are also challenges that patients face concerning data privacy. With increased data collection, concerns about how data is used and who has access to it are paramount. Maintaining robust data security measures is crucial to building patient trust and ensuring the ethical use of health information.
Policy Makers
For policy makers, big data presents both opportunities and responsibilities. Lawmakers can utilize aggregated data to determine health trends, allocate resources, and design more effective healthcare policies. Understanding population health dynamics through big data can lead to targeted public health initiatives aimed at preventing disease outbreaks and managing chronic conditions.
Moreover, data-driven insights strengthen the argument for the allocation of funding and support for various healthcare programs. Evidence showing the impact of specific interventions can lead to more informed policy decisions. Nonetheless, this demands a commitment to data governance frameworks that ensure transparency and accountability in how data is used.
Policy makers must navigate the complexities of regulations and standards as well. Ensuring compliance while maximizing the benefits of big data requires ongoing dialogue with stakeholders. This is vital to balance innovation with safeguarding public interests and ethical considerations.
"The convergence of big data and health informatics presents a transformative opportunity to enhance outcomes across the entire healthcare ecosystem."
In summary, the impact of big data on stakeholders is profound. Healthcare providers, patients, and policy makers each play a crucial role in leveraging these insights for improved health outcomes and system efficiencies. The interplay among these groups creates opportunities for innovation while posing challenges that need careful attention.
Case Studies in Big Data Health Informatics
Case studies in big data health informatics serve as vital demonstrations of how data analysis can directly influence healthcare practices. These case studies provide concrete examples of successful implementations, highlighting meaningful impacts on patient outcomes and operational efficiencies. They also shed light on challenges faced by healthcare organizations. Understanding these real-world applications offers insights that are crucial for stakeholders aiming to harness the potential of big data.
Successful implementations illustrate the potential benefits of integrating big data analytics into healthcare systems. By presenting tangible results, these examples encourage wider adoption.
Successful Implementations
The success stories in big data health informatics often reveal transformative changes in healthcare delivery. One notable case is that of Mount Sinai Health System in New York, which utilized big data analytics to reduce hospital readmission rates. By analyzing patient data, they identified high-risk patients and tailored follow-up care accordingly. This proactive approach not only enhanced patient outcomes but also resulted in significant cost savings.
Similarly, the use of predictive analytics in population health management at Geisinger Health has shown promising results. The organization implemented a data-driven strategy, leading to improved management of chronic diseases. By focusing on high-risk population segments, Geisinger was able to allocate resources more effectively, resulting in enhanced patient care and reduced hospital visits.
These examples highlight key benefits such as:
- Improved patient outcomes
- Enhanced operational efficiencies
- Resource optimization
- Cost reduction
Lessons Learned from Failures
While successful implementations are critical, examining failures is equally important. Learning from unsuccessful attempts provides valuable insights and helps avoid repeating mistakes.
For instance, the case of a large health system that attempted to implement an electronic health record system illustrates significant challenges. The implementation was plagued by poor interoperability and resistance from staff, resulting in workflow disruptions. Ultimately, the project fell short of its goals, leading to wasted resources and dissatisfaction among healthcare providers.
Another example includes a predictive analytics initiative that failed to deliver anticipated results due to inadequate data quality. The project underestimated the importance of clean, reliable data for analysis. This highlights the necessity for healthcare organizations to prioritize data governance and quality control before embarking on big data projects.
Key takeaways from these failures include:
- Ensuring system interoperability
- Fostering staff engagement
- Prioritizing data quality
- Maintaining realistic expectations
"Learning from both success and failure allows healthcare organizations to navigate the complexities of big data effectively."
Epilogue
In this article, we have delved into the critical relationship between big data and health informatics. The convergence of these two fields is not merely a trend; it is a profound transformation that reshapes healthcare systems worldwide. Understanding the implications of big data in health informatics is vital for various stakeholders, including healthcare providers, patients, and policy-makers.
Consolidating Insights and Future Directions
Big data offers the potential to revolutionize healthcare. It provides insights into patient care, operational efficiencies, and innovation. However, realizing these benefits requires navigating several challenges. Key among these are data privacy and security issues, data integration obstacles, and concerns regarding the quality and reliability of the data.
As we look to the future, the following elements emerge as critical considerations:
- Innovation in Data Analytics Tools: Continued advancement in analytics technology will further refine how data is utilized in healthcare. Tools capable of processing and analyzing vast datasets will become standard practice.
- Integration of AI and Machine Learning: These technologies will be essential for predictive analytics in patient care and resource management. They can help identify trends, enhance treatment protocols, and even predict disease outbreaks.
- Regulatory Frameworks: The evolution of big data in health informatics must align with regulatory requirements. Policymakers need to create frameworks that protect patient data while promoting innovation.
- Stakeholder Engagement: Collaboration between various stakeholders can foster a more integrated approach to big data applications in health care. Stakeholders must work together to understand needs and establish best practices.
Big data in health informatics stands at a pivotal point in its development. With the right investments, policies, and collaborations, the healthcare landscape can evolve significantly. Through these ongoing efforts, the potential benefits can be fully harnessed for enhancing patient outcomes and improving overall system efficiencies.