In-Depth Analytics Software Comparison for Professionals
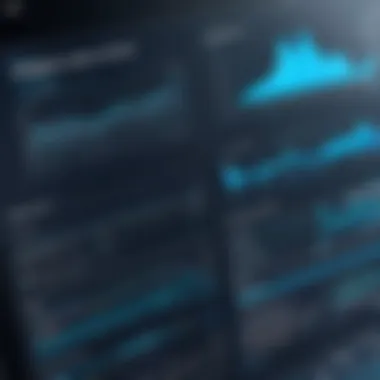
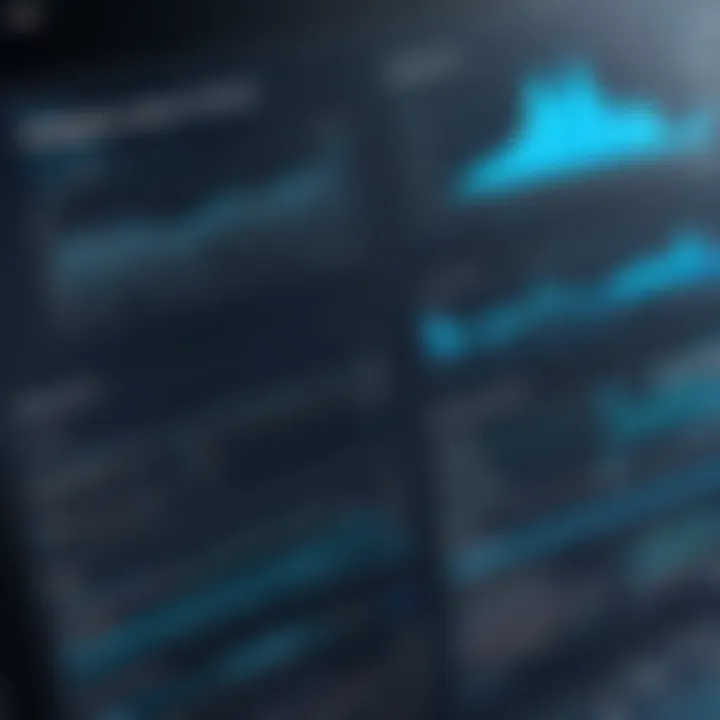
Intro
In today’s data-centric world, choosing the right analytics software is crucial for success. Organizations face an ongoing challenge in selecting the most suitable tool to meet their specific needs. A wide range of options are available, each offering unique features and functionalities. This article aims to explore various analytics software solutions by analyzing their fundamental aspects. By delving into key features and essential comparison metrics, we aim to equip business professionals and tech enthusiasts with the information needed to make informed decisions regarding software investments.
Understanding analytics software is not simply about features, but also how they fit within the broader context of business strategy. As the digital landscape evolves, the significance of data analysis increases. Selecting an analytics tool should align with an organization’s objectives while considering cost, performance, and future growth.
Key Software Features
In any analytics software, certain features stand out as essential components. Identifying these functionalities can streamline the decision-making process for professionals.
Essential Functionalities
Many analytics tools offer essential functionalities that form the foundation of effective data analysis. Some of these include:
- Data Collection: Tools must facilitate seamless data gathering from various sources. This includes databases, third-party services, and even manual uploads.
- Data Visualization: Effective visualization options allow users to interpret complex data effortlessly. Charts, graphs, and dashboards are necessary for making data-driven decisions.
- Reporting Capabilities: Automated reporting tools can help generate regular insights tailored to user preferences, enhancing the overall user experience.
- Integration: The ability to connect with other software solutions like CRM systems and databases enhances the analytical capacity of any tool.
Advanced Capabilities
Beyond the essentials, advanced capabilities are crucial for organizations seeking deeper insights. These may include:
- Predictive Analytics: Utilizing algorithms to forecast future trends based on historical data can give organizations a competitive edge.
- Machine Learning: Advanced tools use machine learning to automatically improve data analysis over time, adapting to user behavior patterns.
- Real-Time Analytics: Some tools can process data in real-time, allowing immediate insights and quick decision-making.
Understanding these features and functionalities helps in choosing software tailored to specific organizational requirements.
Comparison Metrics
To further aid in decision-making, potential buyers must evaluate specific metrics that indicate how well different software solutions perform.
Cost Analysis
The cost of analytics software can vary widely from one tool to another. Decision-makers should consider:
- Licensing Fees: Some software may have a one-time fee, while others require ongoing subscriptions.
- Implementation Costs: Initial setup and training expenses can add to the overall investment.
- Hidden Costs: Consider any additional fees, such as add-ons or charges related to data storage.
Performance Benchmarks
Performance benchmarks provide insight into how analytics software meets user expectations. Important indicators might include:
- Data Processing Speed: The efficiency at which software can handle large datasets is critical for timely insights.
- User Satisfaction Ratings: Reviews and ratings across platforms can offer a glimpse into user experiences with the software.
- Support Services: Analyzing support services helps gauge their responsiveness and effectiveness in resolving issues.
By considering these comparison metrics, professionals can understand the strengths and weaknesses of each software solution before making a commitment.
Understanding Analytics Software
Analytics software has a prominent role in today’s data-driven landscape. Understanding this software is crucial for organizations looking to harness the power of data effectively. Businesses generate enormous amounts of data every day. Analytics software allows for the extraction of significant insights from this data, thus facilitating better decision-making processes.
Definition of Analytics Software
Analytics software refers to a set of programs designed to analyze data sets, uncover patterns, and provide actionable insights. This software can process and interpret complex data to help organizations understand trends and performance. Common tools in this category include Tableau, Power BI, and Google Analytics.
These programs vary in capabilities, allowing users to conduct descriptive analytics, predictive analysis, and more. It is essential to have a clear understanding of what analytics software does, as this knowledge determines how effectively a business can leverage its features.
Importance in Data-Driven Decision Making
Data-driven decision making is the practice of making decisions based on data analysis rather than intuition or observation alone. Analytics software is integral to this approach. It provides the tools necessary to convert raw data into usable information.
Utilizing analytics software has numerous benefits:
- Enhanced Decision Making: With access to accurate data insights, managers and decision-makers can make informed and timely decisions that align with business goals.
- Identifying Trends: Businesses can spot trends and patterns that would otherwise go unnoticed. This capability can lead to new business opportunities or risk mitigation strategies.
- Efficiency Improvements: By automating data analysis, organizations save time and resources, allowing them to focus on strategic initiatives instead of data collection.
- Competitive Advantage: Businesses leveraging analytics software gain advantages over competitors who may not be utilizing data effectively.
"Data is the new oil. It’s valuable, but if unrefined it cannot really be used. It has to be refined to extract the insights." - Clive Humby
Key Features to Consider
In choosing analytics software, the key features play a crucial role in ensuring that organizations find the right fit for their specific needs. Each feature affects usability, efficiency, and the overall effectiveness of data analysis. Thus, decision-makers must pay attention to several aspects when evaluating options. Here, we delve into the principal features that should influence your decision on analytics software.
User Interface and Experience
The user interface (UI) and user experience (UX) are vital because they influence how effectively users can navigate and utilize the software. A clean and intuitive UI minimizes the learning curve. This is especially important for teams that may not have extensive technical training.
When evaluating software, look for:
- Clarity in design: Layout should be structured and logical.
- Customization options: Users should adapt the interface to their preferences.
- Accessibility: Software should be usable across devices and screen sizes.
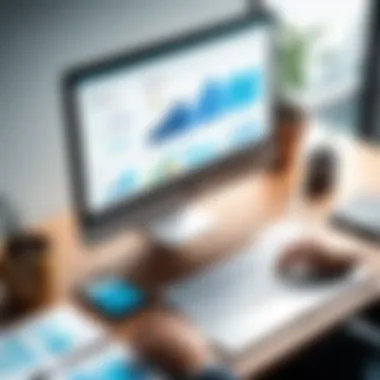
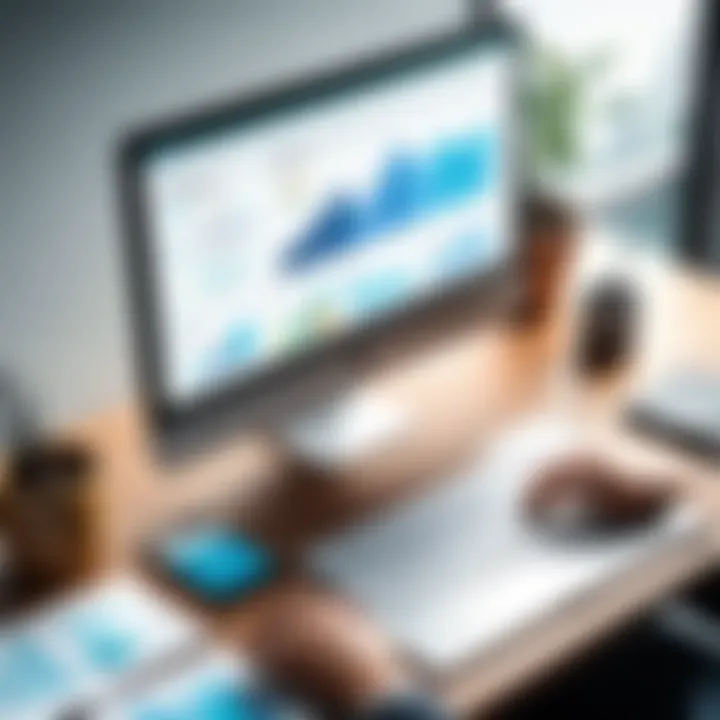
The right UI and UX contribute to increased productivity. If the software is not user-friendly, users may miss out on powerful features simply because they struggle to use them.
Data Visualization Capabilities
Data visualization enhances the understanding of complex data sets. Effective analytics software should provide robust visualization tools that transform raw data into meaningful insights. With well-designed charts, graphs, and dashboards, users can quickly identify trends, outliers, and patterns.
Key points to consider include:
- Variety of visual formats: The software should support various types of visualizations.
- Interactivity: Users must be able to manipulate data visualizations for deeper insights.
- Real-time data updates: Visualization should reflect data changes promptly.
Well-executed data visualization leads to better decision-making, as stakeholders can grasp insights quickly and effectively.
Integration with Other Tools
Integration is crucial for seamless data flow between systems. The ability of analytics software to work with other tools amplifies its usefulness. This is particularly relevant for businesses utilizing multiple software solutions across departments.
When assessing integration capabilities, consider:
- Compatibility with existing systems: The software should easily integrate with your CRM, ERP, or other analytics tools.
- Pre-built connectors: Look for software that offers ready-to-use integrations to simplify setups.
- API access: This allows for customized integration options based on specific business needs.
Strong integration capabilities mean teams can spend less time on data transfers and more time on analysis.
Data Processing Speed and Capacity
Performance is a key feature to evaluate. Analytics software should efficiently handle large data sets, allowing users to process and analyze significant amounts of data swiftly. Slow processing speeds can hinder timely decision-making.
Consider the following performance indicators:
- Processing time: How fast can the software generate results from extensive data?
- Capacity limits: Is there a maximum data volume the software can manage?
- Scalability: Can the software expand as the data needs grow?
Efficiency in data processing enhances productivity and drives quicker insights, allowing organizations to act on their data more effectively.
Security and Compliance Features
Security remains a top concern in any software solution, especially with data privacy regulations in place. Robust security measures protect sensitive business information and ensure compliance with regulations like GDPR and HIPAA.
Important security features include:
- Data encryption: Ensures that data remains secure both at rest and in transit.
- User access controls: Organizations should define who can access specific data sets and functionalities.
- Audit trails: Maintaining records of user actions helps in monitoring and accountability.
Choosing software with strong security and compliance features safeguards the integrity of data and builds trust among users.
Types of Analytics Software
Understanding the types of analytics software is vital for organizations seeking to derive actionable insights from data. Each category serves a distinct purpose and is tailored to address specific business needs. Familiarity with these types can help decision-makers select the right tool that aligns with their strategic goals. Let’s delve into the four main categories: descriptive, diagnostic, predictive, and prescriptive analytics.
Descriptive Analytics
Descriptive analytics provides a summary of past events and data. It answers the question, "What has happened?" by utilizing historical data to describe outcomes and trends. This type allows businesses to access a clear view of incidents, performance, and patterns over time.
Key benefits of descriptive analytics include:
- Data Summarization: It condenses large datasets, making it easier to comprehend.
- Trend Identification: Helps in spotting recurring trends which can signal opportunities or risks.
- Performance Benchmarking: Offers metrics that businesses can measure against for performance evaluation.
Effective tools for descriptive analytics often include robust data visualization tools like Tableau and Microsoft Power BI, which transform raw data into comprehensible visual formats.
Diagnostic Analytics
While descriptive analytics informs about past occurrences, diagnostic analytics digs deeper to explain why events happened. This type helps organizations understand the causes behind trends and metrics. It answers the question, "Why did it happen?"
A few characteristics of diagnostic analytics are:
- Root Cause Analysis: It identifies the factors contributing to specific outcomes.
- Comparative Analysis: Allows side-by-side comparisons to assess variations and deviations.
- What-if Analysis: This offers insights into hypothetical scenarios based on existing data.
Common diagnostic tools include Google Analytics and IBM Watson Analytics, which analyze data for a more profound understanding of performance metrics.
Predictive Analytics
Predictive analytics leverages statistical models and machine learning techniques to forecast future outcomes based on historical data. It answers the question, "What could happen?" by identifying patterns and correlating data points that may influence future trends.
Benefits of predictive analytics involve:
- Risk Management: Helps organizations anticipate potential risks before they occur.
- Customer Insights: Provides detailed predictions about customer behavior and preferences.
- Resource Allocation: Improves decision-making around resource deployment based on expected future scenarios.
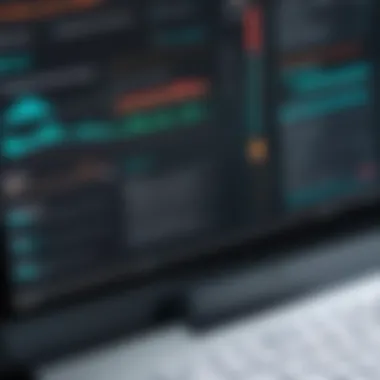
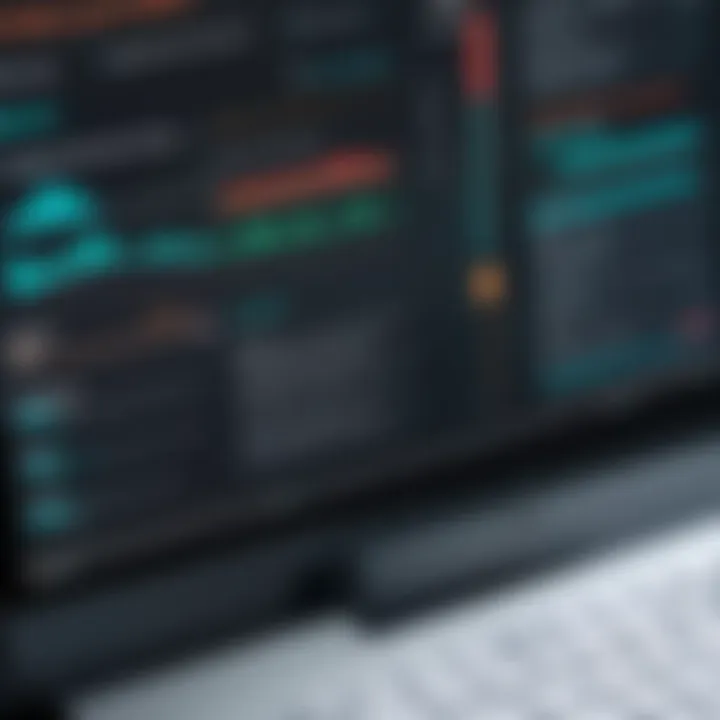
Software such as SAS and RapidMiner are popular choices for constructing predictive models that aid in strategic planning.
Prescriptive Analytics
The most advanced form, prescriptive analytics goes beyond forecasting future trends to recommend actions that can lead to desired outcomes. It answers, "What should we do?" by suggesting actions based on predictions and assumptions about potential future scenarios.
Key aspects of prescriptive analytics include:
- Optimization: Analyzes scenarios to find the best course of action.
- Decision Support Systems: Provides comprehensive insights that can guide decision-making processes.
- Dynamic Simulation: Models how changes affect outcomes in real-time.
Prescriptive analytics tools, such as IBM Planning Analytics and FICO’s Decision Management Suite, equip decision-makers with the necessary frameworks to enact informed, strategic decisions based on comprehensive analyses.
In summary, each type of analytics software offers distinct advantages tailored to different business needs. Understanding these categories aids in selecting a solution that aligns with specific analytical goals.
Market Overview of Analytics Software
The market for analytics software is a rapidly evolving landscape marked by a variety of tools and technologies designed to facilitate data analysis and informed decision-making. Understanding this market is essential for businesses aiming to leverage data effectively. With increasing reliance on data for strategic planning, the importance of selecting the right analytics solution cannot be overstated. This section outlines the key players and emerging trends, equipping readers with insights necessary for making educated software choices.
Leading Analytics Software Providers
Leading analytics software providers have shaped the market through their robust solutions tailored to diverse business needs. Some notable players include:
- Tableau: Focused on data visualization, Tableau has garnered praise for its user-friendly interface and powerful dashboard capabilities.
- Microsoft Power BI: This tool integrates seamlessly with other Microsoft products, enhancing data analysis within organizations already using Microsoft solutions.
- Qlik: Known for its associative data model, Qlik offers unique features for uncovering insights hidden in complex datasets.
These companies excel due to their ability to cater to a wide range of industries while providing tools that are not only comprehensive but also accessible to users without extensive technical training. Their solutions often feature scalability, making them suitable for both small enterprises and large organizations.
Emerging Players in the Market
Emerging players in the analytics software market present innovative solutions that challenge established companies. These newer providers often bring fresh perspectives and technologies, focusing on specific niches or offering advanced features. Some noteworthy names include:
- Looker: Acquired by Google, Looker emphasizes data exploration and utilizes a modern architecture that integrates well with cloud services.
- Domo: Aims to enable data-driven decision making by offering a platform that combines various data sources and presents metrics in real-time.
- Sisense: Known for its ability to handle complex data queries and transform data into interactive dashboards quickly.
The entry of these companies introduces competition, pushing leading providers to enhance their offerings. As such, decision-makers should keep an eye on this segment as it often provides innovative solutions that can meet unique or unaddressed needs in the analytics software ecosystem.
Comparative Analysis of Top Analytics Software
A thorough comparative analysis of top analytics software offers essential insights into the vast array of options available. This process is crucial for any business looking to leverage data more effectively. Understanding the strengths and weaknesses of various tools enables decision-makers to choose the most suitable solution tailored to their specific needs. Key factors to consider include functionality, user experience, integration capabilities, and cost-effectiveness.
When evaluating analytics software, the analysis can guide stakeholders in distinguishing between products that might seem similar at first glance. By diving into detailed descriptions of each solution, businesses can better anticipate the potential impact on operations and overall efficiency. Assured clarity in what each software offers helps avoid common pitfalls associated with software implementation.
Software A Overview and Features
Software A is designed with versatility in mind. Its intuitive user interface simplifies the complexity of data analysis, making it accessible for users with varying levels of technical expertise. Key features include advanced data visualization tools that allow for real-time monitoring of metrics and trends. The software integrates seamlessly with popular platforms like Salesforce and Google Analytics, enhancing its usability. Furthermore, its robust analytics engine can handle multi-source data processing efficiently.
Software B Overview and Features
Software B stands out due to its predictive analytics capabilities. This software utilizes machine learning algorithms to identify patterns and forecast future trends effectively. Among its notable features is an extensive library of pre-built reports that can be customized to meet organizational needs. The user can easily drill down into data, facilitating deeper insights. Additionally, Software B is known for its extensive customer support, including a dedicated help center and regular webinars that keep users informed about new features and best practices.
Software Overview and Features
Software C focuses on collaboration within organizations. Its standout feature is a shared workspace that allows users to collaborate on data projects in real-time. The software is equipped with powerful data cleaning tools which ensure data accuracy. Users appreciate the simple onboarding process and the availability of detailed tutorials. Integration capabilities with tools like Slack and HubSpot further enhance its utility for teams prioritizing seamless communication and data sharing.
Side-by-Side Comparison
| Features | Software A | Software B | Software C | | User Interface | Intuitive | Complex | User-friendly | | Data Visualization | Advanced | Standard | Excellent | | Integration | High | Moderate | High | | Predictive Modeling | No | Yes | No | | Collaboration | Limited | Limited | Extensive | | Customer Support | Standard | Excellent | Good |
In summary, comparing these analytics software options allows companies to understand which tool aligns best with their needs. Software A may suit enterprises with straightforward needs, while Software B appeals to those seeking predictive insights. Software C is ideal for teams that rely on collaboration.
"Understanding your analytics software options ensures you select the right tool for your organization's needs and expectations."
Evaluating Value for Money
Evaluating the value for money of analytics software is crucial for organizations that aim to maximize their return on investment while minimizing unnecessary expenditures. In this context, value for money encompasses not only the initial costs associated with purchasing the software but also the ongoing costs that may accompany its deployment and usage. Decision-makers must consider the full scope of investments as they relate to the productivity gains and operational efficiencies that the software is expected to deliver. The ability to analyze these factors can often determine whether an organization remains competitive in a data-driven landscape.
The following points provide a framework for understanding the importance of evaluating value for money:
- Cost Efficacy: The software should provide adequate features relative to its price point, ensuring that organizations do not overspend on capabilities that may not be fully utilized.
- Equitable Cost Structures: Various products offer differing cost structures, including monthly subscriptions, one-time licenses, and tiered pricing based on usage. Understanding these structures allows businesses to make choices that align with their financial strategies.
- Long-term Savings: Advanced analytics tools can lead to significant cost savings over time. Tools that integrate seamlessly with existing software or improve processes can yield returns that justify the initial costs.
Ultimately, the goal of this evaluation is to ensure alignment between the analytical needs of the organization and the software's capabilities, thereby recognizing true value instead of merely lowest cost.
Cost Structures of Different Solutions
When considering analytics software, organizations encounter diverse cost structures. It is essential to examine these costs in detail to understand the financial commitment required from the outset. Common cost structures include:
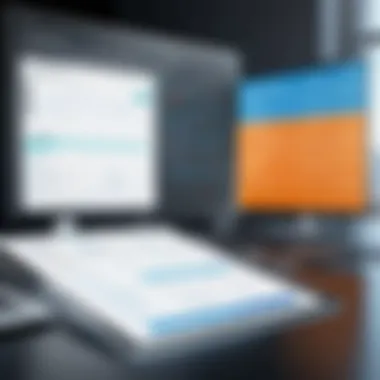
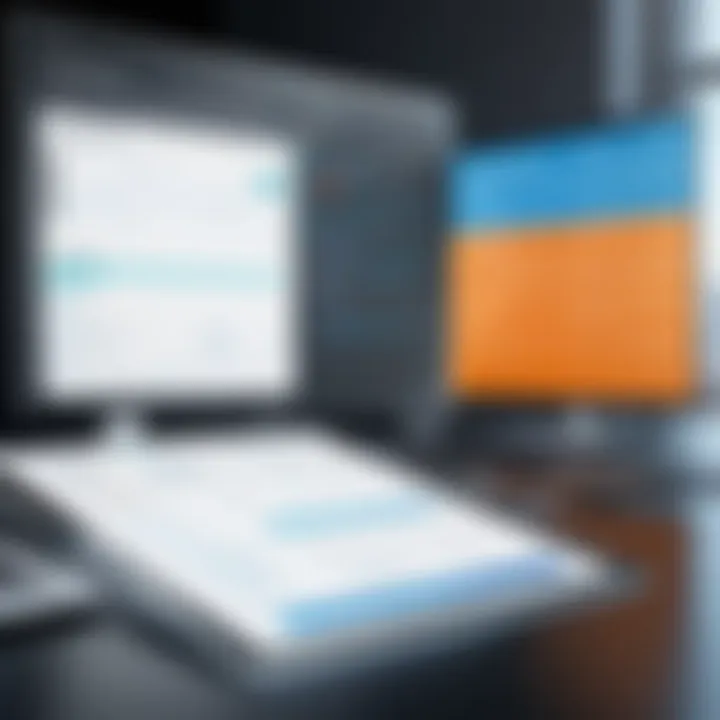
- Subscription Fees: Many software products operate on a subscription model, requiring monthly or yearly payments. This method can be beneficial for budgeting, allowing businesses to scale their usage and associated costs as needed.
- One-time Licenses: Some analytics tools require a single upfront payment for long-term use. While this option may seem appealing due to its lack of recurring fees, it could also entail significant initial costs that may not fit every budget.
- Freemium Models: Certain software companies offer a basic version of their tool at no cost, with the option to upgrade for more features. While this can provide initial access to necessary tools, businesses should be cautious about the limitations of free versions and ensure that they align with their operational needs.
- Usage-based Pricing: Some providers charge based on the amount of data processed or the number of users. This can be advantageous for companies with fluctuating needs but could also lead to unpredictable costs as usage increases.
Assessing these structures helps businesses identify opportunities for budget optimization.
Return on Investment Considerations
When discussing analytics software, return on investment (ROI) serves as a vital metric for decision-makers. ROI not only justifies the expenditure but also highlights the broader implications of deploying such tools within an organization. Key considerations include:
- Operational Efficiency: An effective analytics platform should streamline processes, thereby increasing productivity. Measuring the time saved or improvements in employee output can play a significant role in calculating ROI.
- Enhanced Decision-Making: By delivering insightful data analyses, the right software supports strategic decisions that can lead to revenue growth and market share expansion. Tracking performance metrics related to these outcomes can provide valuable insights.
- Cost Reductions: Identifying areas wherepreviously high expenses can be lowered represents a direct impact on ROI. For example, automating manual reporting processes can free up resources for more valuable tasks.
- Customer Satisfaction and Retention: Software that enables improved customer experiences can drive trust and loyalty, ultimately resulting in repeat business or referrals. Monitoring customer feedback and retention rates can offer quantifiable data for ROI.
To maximize ROI from analytics software, organizations should set clear expectations and align software capabilities with business goals. Evaluating these criteria ensures that investments yield the desired outcomes.
Case Studies and Use Cases
Incorporating case studies and use cases into the discussion of analytics software significantly enhances understanding. These real-world examples illustrate how various sectors implement analytics to achieve specific business outcomes. They offer insights into successful strategies and potential pitfalls, demonstrating the practical applicability of different analytics tools. Business leaders making decisions about software investments benefit from seeing tangible results rather than abstract ideas.
Successful Implementation in Retail
In the retail industry, analytics software is crucial for understanding customer preferences and optimizing inventory management. For instance, a major retailer implemented Tableau to visualize sales data across different regions. By analyzing this data, they identified trends in customer purchases, allowing them to adjust stock levels accordingly. This led to a notable increase in sales and a reduction in excess inventory.
Data-driven decisions in retail not only boost sales but also enhance customer satisfaction. Incorporating analytics allows retailers to predict demand more accurately. Retailers can analyze historical purchase data alongside seasonal trends to prepare for peak periods. Consequently, they can tailor marketing efforts and promotions to align with consumer behavior.
"Analytics are essential in retail environments where customer preferences can change rapidly. Using data provides competitive advantage."
Application in Financial Services
In financial services, the stakes are often high. Here, analytics software is not only useful but essential for risk assessment and customer insights. A leading bank used SAS Analytics to improve its credit risk models. By analyzing customer data, the bank identified high-risk borrowers with a greater level of accuracy. This implementation resulted in substantial reductions in loan defaults.
Additionally, financial institutions leverage analytics to enhance customer experiences. By analyzing transaction patterns, institutions can identify fraud more effectively, leading to improved security measures. Furthermore, by segmenting customers based on their financial behavior, banks can tailor their services to meet specific needs.
Future Trends in Analytics Software
The landscape of analytics software is rapidly evolving as businesses embrace new technologies. Understanding these trends is crucial for decision-makers, IT professionals, and entrepreneurs who rely on data-driven insights. Newer technologies empower organizations to stay competitive and responsive to market demands. A comprehensive grasp of future trends allows professionals to make informed choices about software investments.
Artificial Intelligence and Machine Learning Integration
Artificial Intelligence (AI) and Machine Learning (ML) represent significant advancements in analytics software. These technologies enhance data analysis capabilities, offering predictive analytics and automation that were previously unattainable. By integrating AI and ML, analytics software can uncover patterns and insights much faster than manual analysis.
With AI-driven algorithms, software can process vast amounts of data from multiple sources, providing real-time insights. This capability boosts efficiency and allows businesses to make quicker decisions. For example, tools like IBM Watson and Google Cloud AI use predictive models to forecast outcomes based on historical data, enabling businesses to strategize proactively.
Benefits of AI and Integration:
- Improved accuracy in predictions
- Automation of routine tasks
- Enhanced personalization for user experiences
- Ability to adapt to changing data patterns
As organizations adopt AI and ML, they position themselves at an advantage—leveraging advanced analytics to meet their custom business needs so. This evolution emphasizes the need for professionals to stay informed about these technologies to harness their full potential.
Advancements in Data Processing Techniques
Data processing techniques are also undergoing significant change, central to analytics software functionality. The shift from traditional processing methods to cloud-based solutions has transformed how data is stored, analyzed, and shared. Cloud platforms enable scalability and flexibility, allowing businesses to adapt their operations seamlessly as data demands grow.
Additionally, the rise of real-time processing capabilities enhances the ability to glean insights instantly. Streaming analytics is becoming more commonplace. This technique allows businesses to analyze data as it is generated instead of waiting for batch processing. Tools like Apache Kafka exemplify this shift, providing robust frameworks for real-time data processing.
Key Advancements in Data Processing Techniques:
- Transition to cloud computing
- Adoption of real-time analytics
- Enhanced data storage solutions, such as NoSQL databases
- Increased focus on data quality and governance
These advancements impact how businesses interact with their data and ultimately make data-driven decisions. By understanding the latest processing techniques, decision-makers can optimize their data strategies and improve operational efficiency.
Final Considerations
The topic of final considerations plays a critical role in the context of analytics software selection. It encapsulates the primary elements that decision-makers must reflect on to ensure they make an informed choice. Given the complexity of the digital landscape, a systematic approach to selecting analytics software helps align business needs with technological capabilities. Key factors to think about include usability, scalability, support, and the overall alignment of the software with the organization’s goals.
Effective decision-making relies on evaluating both the tangible and intangible benefits of each software option like how well it can function within the existing infrastructure. This helps avoid common pitfalls such as costly implementation failures or choosing a product that does not meet future needs. Being diligent in the final consideration phase thus serves as a safeguard against potential issues.
"The right analytics software can pave the way for enhanced decision-making, driving efficiency and growth across all business dimensions."
Choosing the Right Analytics Software for Your Needs
Selecting the right analytics software is paramount for any organization. It requires a clear understanding of both the technical requirements and the strategic goals of the business. When evaluating different options, one must consider the specific analytics needs, the type of data being analyzed, and who will ultimately use the software.
- Identify Key Requirements: List down the features that are critical for your organization, such as real-time analytics, customizable dashboards, and advanced data visualization tools.
- Engage Stakeholders: Involve key team members from various departments in discussions about their requirements. This promotes a more inclusive approach and ensures that diverse needs are met.
- Consider Future Growth: Choose software that not only meets current needs but is also scalable, allowing for future growth and adaptation. This may involve evaluating the software’s capacity to integrate with other tools and systems.
By taking the time to analyze these aspects, organizations can better position themselves to select a software solution that aligns with their long-term objectives and operational strategies.
Getting Started with Implementation
Initiating the implementation process is the next step after selecting the appropriate analytics software. A well-planned approach minimizes disruption and maximizes the utility of the new system. Here are a few considerations to help ease the transition:
- Create an Implementation Team: Gather a group of individuals who are versed in analytical processes as well as IT staff who can manage the technical aspects of the deployment.
- Develop a Roadmap: Outline a clear implementation roadmap that includes timelines and milestones. It is crucial to set achievable goals and regularly update stakeholders on progress.
- Training and Support: Making sure that all users receive adequate training on how to use the new software is essential. Consider arranging for ongoing support post-implementation to address any challenges that may arise.
In summary, a thoughtful implementation process not only facilitates smoother operation but also ensures that users fully capitalize on the capabilities of the analytics software, translating insights into strategic benefits.