Mastering A/B Testing with SurveyMonkey Strategies
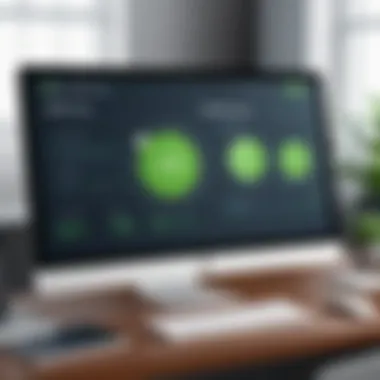
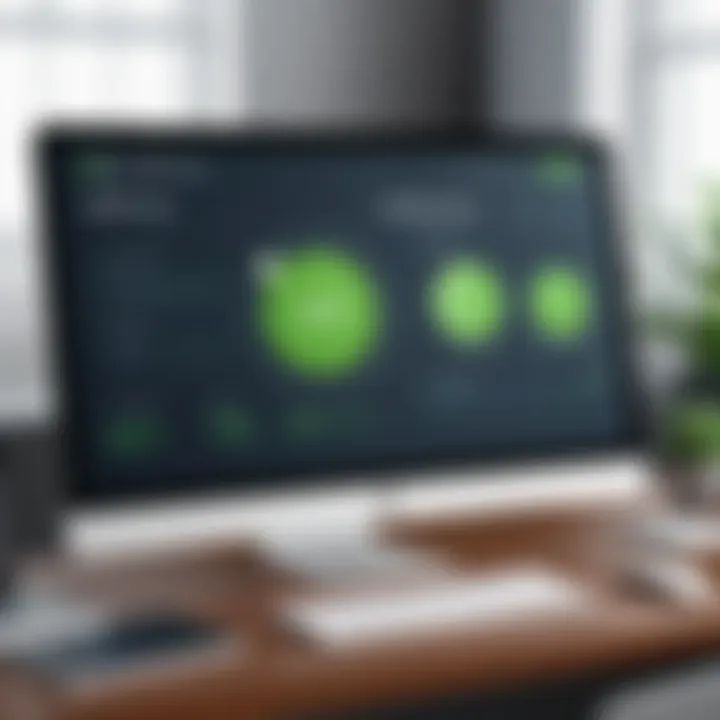
Intro
In a world driven by data, decision-makers are constantly faced with the challenge of choosing the right path forward in numerous areas, be it marketing, product development, or even customer service. To navigate this realm of uncertainty, A/B testing has emerged as a vital strategy. This method enables organizations to compare two versions of a product or service to determine which one performs better based on specific metrics. Notably, SurveyMonkey offers a robust platform tailored for A/B testing, making it easier to harness insights that directly contribute to strategic business decisions.
Understanding the basics of A/B testing with SurveyMonkey not only demystifies this powerful tool but also opens up a treasure trove of opportunities for optimizing user experiences. Thus, diving into how A/B testing functions, along with the intricacies associated with using SurveyMonkey, becomes essential for any enterprise aiming to enhance its performance.
While it may seem straightforward at first glance, A/B testing is layered with methodologies and practices that can turn mere data collection into actionable insights. This article uses real-world examples and practical guidance to help leaders make the most out of A/B testing, ensuring they stay ahead of the curve by experimenting and learning about their audience all the while.
The journey through A/B testing with SurveyMonkey begins with an exploration of its key software features. Knowing what tools are available can shed light on crafting effective experiments that yield valuable outcomes.
Prelims to A/B Testing
A/B testing has carved a space for itself in the realm of data-driven decision-making, especially in today’s digital world, where every click, interaction, and conversion matters. It serves as a critical tool for organizations aiming to optimize their strategies, facilitating a more informed approach toward understanding user preferences. This section dives into what A/B testing is fundamentally about, the essence of its practice, and why decision-makers ought to prioritize it within their digital strategy framework.
Defining A/B Testing
At its core, A/B testing is a method for comparing two versions of a single variable to determine which one performs better. Imagine you're at a bakery with two types of pastries. You want to figure out which one your customers would love more. By offering both options simultaneously to different groups, you can analyze their preferences.
In the digital landscape, A/B testing typically applies to websites, emails, or any digital touchpoint. It involves showing one group of users version A and another group version B, tracking metrics such as click-through rates and conversions.
This systematic approach allows businesses to make empirical decisions based on actual user behavior rather than gut feelings or assumptions, allowing for a more precise targeting of user needs and market demands.
Importance of A/B Testing in Digital Strategy
Integrating A/B testing into a digital strategy is not just smart; it's fundamental. Here are a few key reasons why:
- Data-Driven Decisions: Knowledge is power. A/B testing empowers companies with real, actionable data rather than relying on anecdotal evidence.
- Improved User Experience: Constantly tweaking and refining based on user feedback means a more engaging product or service. Users tend to stick around longer when they feel that their opinions and actions matter.
- Higher Conversion Rates: A/B testing focuses directly on enhancing user journeys, which translates to improved conversion rates. A small change in a call-to-action button can lead to significant results.
- Learning Opportunities: Beyond just immediate results, A/B testing paves the way for ongoing learning about audience preferences and behaviors, refining marketing messages, and tailoring services.
"A/B testing can take personas and user journey maps and ground them in reality, offering insights that are often unseen in traditional methods."
In summary, A/B testing is not just a tool but a core component that informs strategic decision-making. For entrepreneurs and IT professionals, acknowledging its significance could mean the difference between simply existing in the market or leading it.
SurveyMonkey Overview
SurveyMonkey stands as a venerated name in the field of online survey tools, offering a platform that isn’t just about collecting data but also about shaping insights that can transform business strategies. Understanding its role is pivotal for digital marketers, product managers, and decision-makers who lean heavily on data-driven insights to guide their actions.
History and Evolution of SurveyMonkey
Founded in 1999, SurveyMonkey began under the auspices of Dave Goldberg and his co-founders with a vision for easy and accessible survey tools. Initially, the platform aimed to democratize surveys, empowering users from all backgrounds to collect information without being bogged down by complex processes. Fast forward to today, and SurveyMonkey has matured into a multifaceted platform, continually adapting to advancements in technology and user expectations. Its growth journey mirrors the expansion of digital communication, evolving from simple text-based surveys to incorporating multimedia elements, enriched analytics, and integrations with a burgeoning number of other applications. As the demand for actionable insights continues to escalate, SurveyMonkey thrives by tapping into emerging trends, such as mobile compatibility and real-time reporting capabilities.
Features and Capabilities of SurveyMonkey
SurveyMonkey is more than just a survey creator—it's an arsenal for those serious about gathering and interpreting data. Here are some noteworthy features that set it apart:
- User-Friendly Interface: The design makes it easy for anyone to craft a survey without needing any specialized training. Just point and click!
- Customizable Templates: Users can choose from a variety of templates tailored for different industries and objectives, saving valuable time in survey creation.
- Advanced Analytics: Beyond simple result tallying, SurveyMonkey provides analysis tools that allow users to interpret data deeply. Visualization features help in presenting findings that are easily digestible.
- Integration with External Apps: It plays well with others, offering integration with tools such as Mailchimp and CRM systems, streamlining the workflow for marketers.
- Robust Security Measures: For businesses dealing with sensitive information, SurveyMonkey provides various security options to ensure that data remains confidential and protected.
"With the right tools like SurveyMonkey, the complexity of data collection becomes a straightforward process, empowering organizations to focus on what really counts—insightful decision-making."
SurveyMonkey encompasses many capabilities that cater to both individual users and large teams, making it an essential tool in a world increasingly driven by data. Whether adjusting marketing strategies or understanding customer sentiments, it provides the necessary elements to conduct effective A/B testing that informs larger business decisions.
The A/B Testing Process
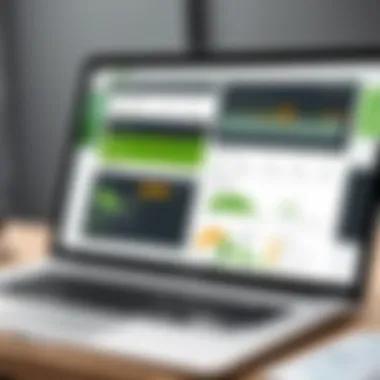
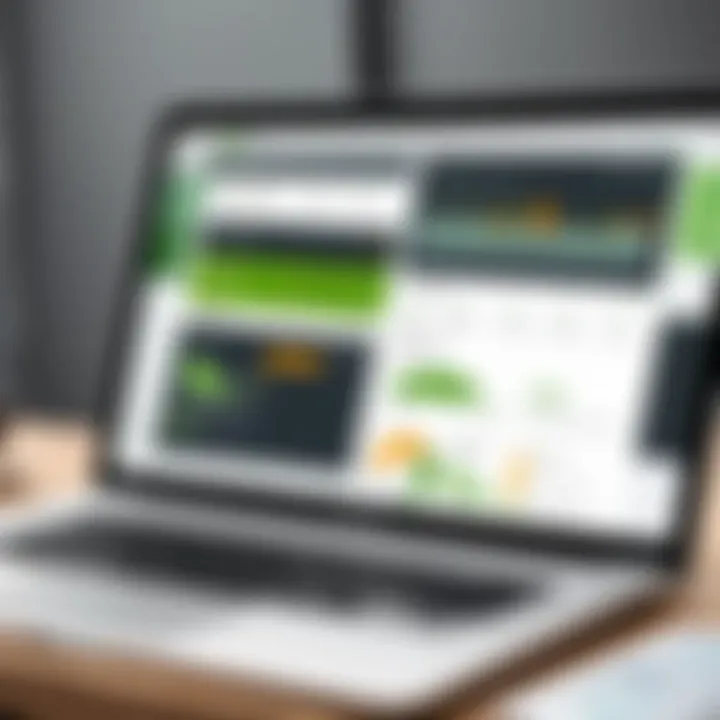
The A/B testing process serves as the backbone of evaluating how different versions of a web element or marketing strategy perform compared to one another. By clearly defining hypotheses and systematically testing them, businesses can fine-tune offerings and maximize the impact of their campaigns. This process not only enhances decision-making based on data, but it also fosters an environment of sustainable growth. When implemented correctly, A/B Testing provides insights that can pivot a company's strategy in new and beneficial directions.
Setting Clear Objectives
Before diving headfirst into A/B testing, establishing clear and concise objectives is imperative. These objectives serve as a roadmap, guiding the entire testing phase. For instance, a business looking to increase the click-through rate of its email campaigns might aim to answer specific questions, like:
- "What subject line resonates best with recipients?"
- "Does a personalized greeting impact engagement?"
By defining these goals, teams can avoid the common pitfall of wandering aimlessly through tests without actionable insights. This lets you focus on metrics that genuinely matter. One effective method is using the SMART criteria, ensuring that each objective is Specific, Measurable, Achievable, Relevant, and Time-bound. In practice, setting an objective such as "increase the newsletter sign-ups by 15% by the end of Q2" provides a clear target to aim for.
It's about hitting the nail on the head and ensuring everyone involved understands what success looks like.
Identifying Key Metrics
After setting objectives, the next on the agenda is figuring out what key metrics will illustrate success or failure. Think of these metrics as the compass guiding your testing direction. Depending on your objectives, relevant metrics might include:
- Conversion Rates: The percentage of users who complete a desired action, like making a purchase or signing up for a newsletter.
- Engagement Levels: Measured through click rates, time spent on a page, or interactions with content.
- Bounce Rates: The percentage of visitors who leave a page without further interaction can be especially telling when assessing page design or content.
Choosing the right metrics doesn't just help evaluate outcomes; it also informs adjustments along the way. The trick is to select metrics that align closely with your objectives. For example, if your goal is to increase user engagement, focusing solely on conversion rates might not tell the complete story. Thus, understanding which metrics matter to your objectives is like choosing the right tool for a specific job—crucial for success.
Designing Your Experiment
When it's time to put the plan into action, experiment design becomes vital. This phase is where creativity meets structure—the essence of A/B testing lies in its ability to compare variations effectively. Key factors to take into account when designing your tests include:
- Control and Variation: Establishing a control group is crucial, as it serves as the baseline against which the variations are measured. For example, if you want to test two different types of call-to-action buttons, the first version (say, a classic red button) will act as your control while the second version (a striking blue button) plays the role of the variant.
- Sample Size: Ensure you have a statistically significant sample size. Small sample sizes can introduce variability, leading to unreliable results. As a ballpark figure, larger campaigns may require hundreds or thousands of respondents to yield meaningful data.
- Duration of the Test: Timing is also an essential factor. Depending on traffic and user interaction patterns, it’s advisable to run tests long enough to account for variance due to factors like time of day or day of the week.
Each design choice can significantly influence the outcomes, making this step crucial in ensuring the tests yield reliable, actionable insights. It's not merely about launching a test and hoping for the best; every decision should be made with a strategic lens aimed at garnering the most informative results and fine-tuning your marketing efforts.
Implementing A/B Testing with SurveyMonkey
In the realm of digital marketing and user experience design, A/B testing stands as a cornerstone for optimizing strategies and ensuring every decision is backed by solid data. When we talk about implementing A/B testing effectively using SurveyMonkey, it’s all about making informed choices based on user interaction and feedback. This process does not just include the act of testing different versions; it's about creating a structured methodology that can lead to substantial insights and measurable improvements.
Creating Variants for Testing
The first step when rolling out A/B tests is to create distinct variants of your content or interface. Variants are basically the different versions you wish to test against each other. For instance, if you have an email marketing campaign, you might want to test two subject lines: "Exclusive Offer Just for You!" versus "Match Your Style with Our Latest Collection!".
When designing these variants, ensure that each is meaningful and strategically conceived. Don’t just swap colors or change the font as if they are mere prettifiers; focus instead on elements that matter to user engagement, like calls to action, layout adjustments, or even wording that could bring a different emotional response. Think about how these changes align with your overall objective. In doing so, you not only make the test relevant but also increase its effectiveness.
Using SurveyMonkey’s A/B Testing Tools
SurveyMonkey provides several tools tailored to assist with A/B testing. These tools allow you to design, conduct, and analyze your tests seamlessly. To start using these features, first set up your variants in their platform. You can utilize features such as custom branding and skip logic to mold the experience to match the expectations of your target audience.
Beyond just setting up tests, take advantage of built-in analytics features that SurveyMonkey offers. You can track responses and interactions automatically, which eliminates much of the manual data crunching often associated with A/B testing efforts. Additionally, review recorded metrics such as completion rates, responses based on variant interactions, and overall engagement times. This data provides invaluable insights on which version performs best based on real user behavior.
"A/B testing isn't just a box to check; it’s a continuous journey of learning that saves time and enhances decision-making."
Sampling and Targeting Audiences
Choosing the right audience for your A/B tests is crucial. If you cast a wide net, you might find meaningful data, but often it won't represent your ideal customer. Instead, buckle down on targeting. Look closely at your sampling methods. Would they accurately reflect your demographic? In some cases, it might be beneficial to segment users based on characteristics such as age, location, or previous purchase behavior.
When you gather feedback, divide your audience effectively across the variants. Ensure that the sampling process is random to help mitigate biases. This way, your test data will be more reliable, leading to decisions that genuinely enhance user experience. Also, keep in mind the sample size; larger sample groups lead to more reliable outcomes. To finalize your selections, evaluate current data on your user base, ensuring that the audiences for your A/B tests align with your marketing goals.
Implementing these A/B testing strategies through SurveyMonkey can yield fantastic insights and refine your approach, creating a more user-centered and data-driven framework for your digital strategies.
Analyzing A/B Test Results
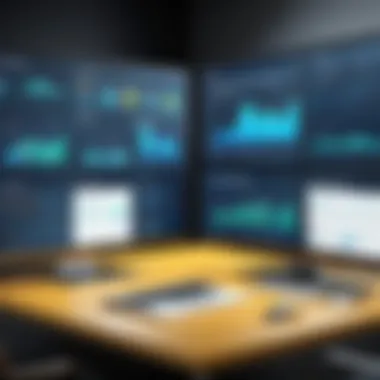
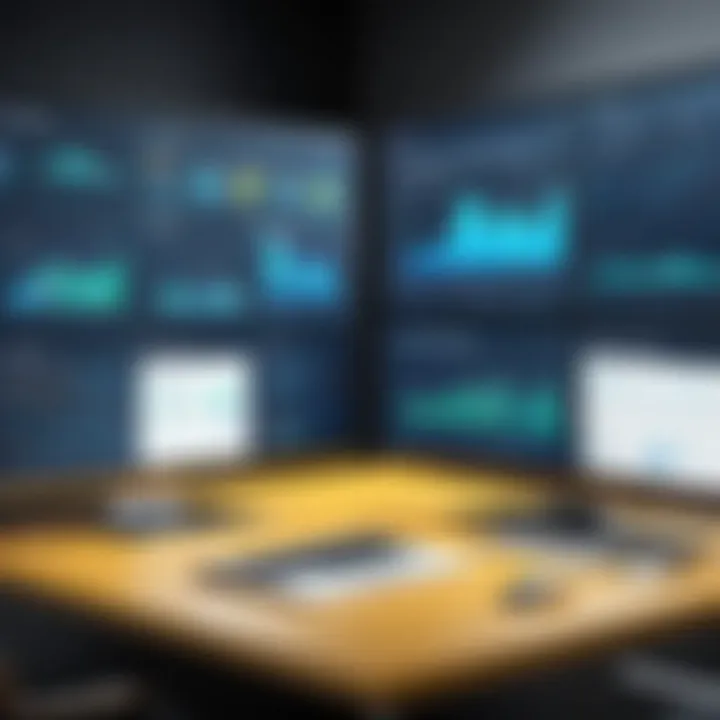
Analyzing A/B test results is a crucial phase in any testing process, serving as the bridge between conducting experiments and making informed decisions based on those outcomes. This stage doesn't merely consist of viewing numbers and percentages; it’s about deriving actionable insights that can impact strategy and operational effectiveness. In essence, the depth of analysis transforms raw data into a narrative that can shape future actions.
The effectiveness of A/B testing is gauged best not in its execution but in its analysis.
Interpreting Data from Surveys
The process of interpreting data from surveys requires a fine-tuned approach. When results come in, first things first: examine the response rates and demographic breakdowns. Higher response rates are more indicative of accurate data, offering a clearer view of your target audience's preferences. It’s also essential to sift through qualitative responses alongside quantitative metrics. The hard numbers provide scale, but the comments often reveal the 'why' behind choices.
In practical terms, one might encounter a scenario such as a landing page A/B test that shows Variant A securing a 65% sign-up rate, while Variant B lags behind at 50%. At first glance, it’s a slam dunk for Variant A. But if qualitative feedback indicates that users are confused by the messaging on Variant A, your analysis should steer you towards understanding that while Variant A performs better in metrics, Variant B might offer longer-term user engagement due to clearer communication. This kind of insight pushes decision-makers to think beyond numbers and consider deeper questions.
Statistical Significance in A/B Testing
Statistical significance is often a linchpin in interpreting A/B testing outcomes. Without it, the conclusions drawn from the tests may lead to misguided strategies rather than informed decisions. Statistical significance quantifies whether the observed differences between variants are likely to be genuine or just chance fluctuations. Using a standard alpha threshold of 0.05 is common, meaning there's only a 5% risk that the results occurred by random chance.
For further clarity, if you discover that your Variant A has a p-value of 0.03 against Variant B, it indicates strong statistical significance. This suggests that you can reasonably conclude that A's performance isn't just a fluke. Conversely, a p-value above 0.05 would caution against assuming that any difference is meaningful. Monitoring these values should be paired with a comprehensive understanding of your audience, as statistical significance may not always speak to practical significance, which is equally important.
Recognizing and leveraging statistical significance not only strengthens your strategic outcomes but also instills confidence in stakeholders who rely on data-backed validation for decision-making.
Utilizing the strength of SurveyMonkey’s tools, you can automate much of this analysis process, ensuring that your insights are both robust and reliable.
In summary, analyzing A/B test results is an intricate mix of understanding numbers, context, and audience sentiment. It encourages a deeper dive into the implications of the data while also cultivating a mindset of continuous improvement rooted in evidence-based decisions.
Best Practices for A/B Testing
Utilizing A/B testing effectively ensures that organizations optimize their strategies, making data-driven decisions that lead to better user engagement and increased conversions. Best practices guide the process, allowing teams to pinpoint what truly resonates with their audience while avoiding common traps that could derail an otherwise fruitful experiment.
A well-structured A/B testing approach not only enhances reliability but also streamlines the path to actionable insights. Indeed, embarking on this journey is about more than merely swapping elements; it’s about weaving a systematic narrative that provides clarity and direction.
Common Pitfalls to Avoid
Every strategist knows that navigating the A/B testing landscape presents a maze of potential missteps. Identifying and avoiding these pitfalls can save time and resources. Here are a few critical areas to pay attention to:
- Testing Too Many Variables at Once: While it may be tempting to assess various elements simultaneously, this technique often muddies the waters. Focus on one change at a time.
- Neglecting Sample Size: A small sample can lead to skewed results. Insufficient participants may prevent capturing a statistically significant outcome.
- Ignoring External Factors: Market trends or seasonal changes can influence user behavior. Ensure that tests are conducted in a controlled environment to avoid interference.
- Failing to Define Success Metrics Clearly: Before diving in, establish what success looks like. This clarity serves as a beacon throughout the testing phase.
Taking heed of these common traps can drastically enhance the precision of the testing process, allowing for more reliable conclusions.
Iterative Testing and Continuous Improvement
The beauty of A/B testing lies in its nature of iteration. Rather than treating each test as a definitive end, it should be viewed as a pivotal step in a larger journey. Continuous improvement cultivates a culture of growth and adaptability within the organization.
- Refining Tests Regularly: After each round of testing, analyze outcomes. Gather insights and make informed adjustments for future tests.
- Implementing Feedback Loops: Just as the tides change, user preferences do too. Establish mechanisms to gather ongoing feedback. This ensures that testing evolves with the audience’s needs.
- Learning from Failures: Not every test will yield a favorable outcome. However, these failures can impart valuable lessons. Acknowledge what didn’t work and pivot from there.
By creating and nurturing an environment where iterative testing thrives, organizations can not only foster innovation but also strengthen their decision-making framework. This relentless focus on improvement carves out a path towards sustained user satisfaction and business growth.
Case Studies of Successful A/B Testing
In the realm of digital strategy, case studies reign supreme as they provide concrete evidence of the effectiveness of A/B testing. They illustrate how systematic experimentation can lead to enhanced user interactions, increased conversion rates, and ultimately, a healthier bottom line. This section underscores the significance of learning from real-life applications of A/B testing, particularly through the lens of SurveyMonkey. By observing how various businesses across different industries have benefited from A/B testing, decision-makers can glean insights that may inform their own strategies and improve outcomes.
Notable Examples in Various Industries
When it comes to A/B testing, success stories abound, showcasing diverse applications across sectors. Here are a few remarkable examples:
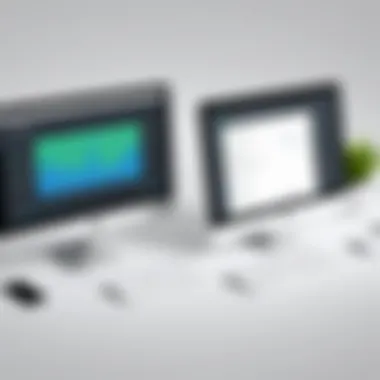
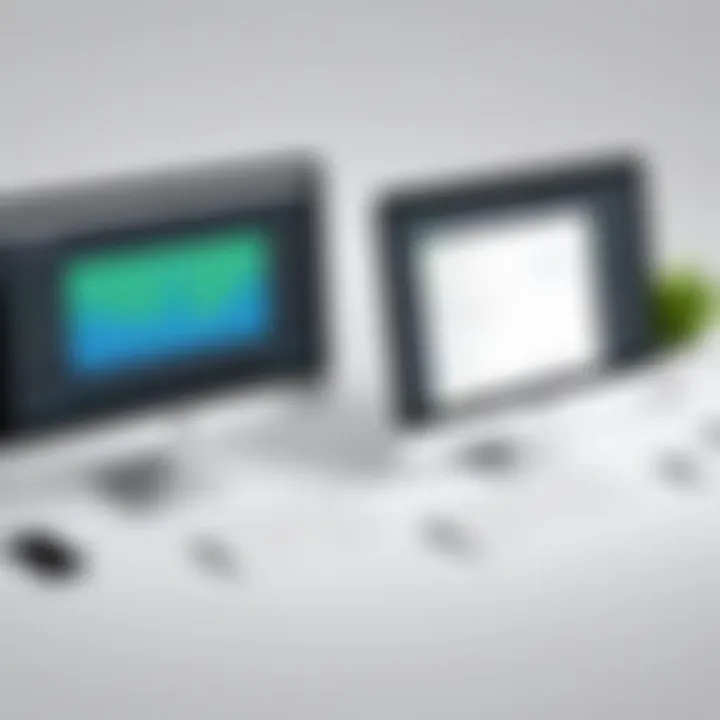
- E-commerce Platforms: A leading online retail company found that changing the color of their "Buy Now" button from green to red resulted in a staggering 20% increase in sales. This quick tweak not only highlighted the importance of aesthetics in user action but also how small changes can have major impacts.
- SaaS Companies: A popular project management software conducted A/B testing on their landing page. By placing testimonials higher up on the page, they boosted user sign-ups by 15%. This case exemplifies the value of social proof in driving conversions, particularly in a competitive software market.
- Content-Driven Websites: A prominent traveler’s blog utilized A/B testing to determine which type of call-to-action (CTA) garnered more email newsletter sign-ups. By experimenting with different wording and button placements, they improved their subscription rates by nearly 30%. Not only did this enhance their mailing list, but it also expanded their audience reach significantly.
Each of these cases illustrates the nuanced way A/B testing can yield actionable insights. It’s not just about numbers; it’s about understanding user behavior, making informed choices, and fostering engagement.
Lessons Learned from A/B Testing Experiences
Analyzing A/B testing outcomes offers more than just positive results; it provides critical insights into the journey of testing and adaptation. Here are some valuable lessons drawn from various experiences:
- The Importance of Hypothesis: Formulating a clear hypothesis before testing guides the process. It helps in setting expectations and provides a focus for analysis.
- Embrace Iteration: A/B testing is not a one-and-done affair. Ongoing testing allows businesses to continually optimize and adapt to changing consumer preferences.
- Value of Segmentation: Different segments of the audience may react differently to changes. Tailoring tests to specific demographics can reveal unique insights that lead to better targeted strategies.
- Data-Driven Decisions: While intuition can guide strategies, aligning decisions with robust data is paramount. Successful companies often rely on calculated insights rather than assumptions when pivoting their approaches.
After all, A/B testing isn’t just about what works; it’s about understanding why it works and how businesses can build on that knowledge to evolve continuously.
"To succeed in business, you have to be willing to experiment, learn, and adapt. A/B testing is a fundamental piece of that puzzle."
The narratives from these case studies reinforce the notion that A/B testing is not merely a method for refining processes; it is a strategic approach that can transform businesses. By learning from those who have blazed the trail, others can avoid pitfalls and leverage the power of data to make informed decisions.
Future Trends in A/B Testing
As the fields of digital marketing and user experience continue to evolve, it’s imperative for businesses to stay ahead of the curve. The future of A/B testing is no exception. With rapid advancements in technology, particularly in artificial intelligence and machine learning, the landscape of A/B testing is set to transform significantly. Understanding these trends helps organizations make informed decisions, ultimately leading to improved user engagement and conversion rates.
Artificial Intelligence and Machine Learning Integration
The integration of artificial intelligence and machine learning into A/B testing processes boosts not only efficiency but also effectiveness. These technologies can analyze data at an unprecedented pace and depth, turning mountains of information into actionable insights.
Consider the following benefits of AI and ML in A/B testing:
- Automated Decision-Making: AI can identify patterns in user behavior, predicting outcomes based on historical data. This means that decisions can be made faster, reducing the need for manual data crunching.
- Personalization: Machine learning algorithms can tailor user experiences based on real-time data. For example, if a user consistently responds better to certain styles or formats, AI can adjust testing variants accordingly.
- Dynamic Testing: Instead of fixed A/B tests, AI can adapt experiments in real-time, automatically shifting resources to the variant performing better. This maximizes potential gains from experimentation.
By leveraging these technologies, businesses can not only enhance their testing processes but also gain deeper insights into user preferences, allowing for a more strategic approach to optimization.
Evolving User Behavior Insights
As user behaviors and preferences shift, so too must the strategies used in A/B testing. Understanding these evolving insights allows companies to stay relevant. Here are several key factors to consider:
- Mobile Versus Desktop: User interaction on mobile devices often differs significantly from desktop interaction. A/B tests need to account for these variances to optimize the user experience effectively.
- Geographical Influences: Different regions may have distinct user behaviors. Tailoring tests to reflect these differences can lead to more relevant outcomes.
- Changing Expectations: As technology advances, users’ expectations evolve. What worked last year may not suffice now. Continuous testing and adaptation to these shifts ensures that companies remain aligned with their customers' needs.
A/B testing must be seen as an ongoing process, not just a one-time effort. Staying attuned to user behavior is essential for sustained success.
Epilogue
A/B testing forms the backbone of data-driven decision-making in today's digital ecosystem. As businesses continue to navigate the complexities of customer expectations and market dynamics, revisiting the value of A/B testing becomes paramount. This method provides clarity, which is crucial for optimizing user experiences. The importance of A/B testing lies not just in its ability to inform strategic choices, but also in how it fosters a culture of continuous improvement. It’s about fine-tuning every interaction to align with audience preferences. This focus ensures not only increased conversion rates but also heightened customer satisfaction.
Revisiting the Importance of A/B Testing
A/B testing offers multifaceted benefits that cannot be overlooked. It allows businesses to experiment without the fear of making sweeping changes based on gut feelings alone. Instead, they can back decisions with solid data garnered through tests. This data-driven approach helps underline several critical aspects:
- Incremental Gains: Even minor tweaks can lead to significant improvements in user engagement.
- Enhanced Understanding: Testing illuminates user behavior patterns, helping teams grasp what resonates with their audience.
- Reduced Risk: By validating changes through A/B testing, organizations limit the potential backlash from large-scale changes.
In essence, A/B testing is akin to holding a mirror up to your strategies, showing what works and what doesn't. Ignoring this powerful tool means companies might miss out on aligning their offerings with market needs.
Final Thoughts on Utilizing SurveyMonkey
When it comes to utilizing SurveyMonkey for A/B testing, the platform offers a robust set of features tailored for marketers and decision-makers. Its intuitive interface simplifies the A/B testing process, making it accessible for users at all levels of expertise. The tools allow for the collection and analysis of valuable feedback, which further drives strategic decisions.
Utilizing SurveyMonkey brings added benefits:
- Flexibility: Create varied question types that suit diverse testing needs.
- Comprehensive Analytics: Access insightful analytics that aid in deriving meaningful interpretations from test results.
- Wide Reach: Tap into a vast audience to ensure your tests reflect genuine user preferences.
Finally, integrating A/B testing into your strategy isn’t just a nice-to-have anymore; it’s a necessity. As competition grows fiercer, leveraging these methodologies not only informs marketing decisions but also contributes to a holistic approach toward achieving business objectives. Ultimately, applying A/B testing through tools like SurveyMonkey can transform insights into action, ensuring that businesses remain agile and responsive to their audiences.